Rating Prediction with Contextual Conditional Preferences.
KDIR(2016)
摘要
Exploiting contextual information is considered a good solution to improve the quality of recommendations, aiming at suggesting more relevant items for a specific context. On the other hand, recommender systems research still strive for solving the cold-start problem, namely where not enough information about users and their ratings is available. In this paper we propose a new rating prediction algorithm to face the cold-start system scenario, based on user interests model called contextual conditional preferences. We present results obtained with three publicly available data sets in comparison with several state-of-the-art baselines. We show that usage of contextual conditional preferences improves the prediction accuracy, even when all users have provided a few feedbacks, and hence small amount of data is available.
更多查看译文
关键词
Recommender Systems,Context Awareness,Conditional Preferences,Rating Prediction,Cold-start Problem
AI 理解论文
溯源树
样例
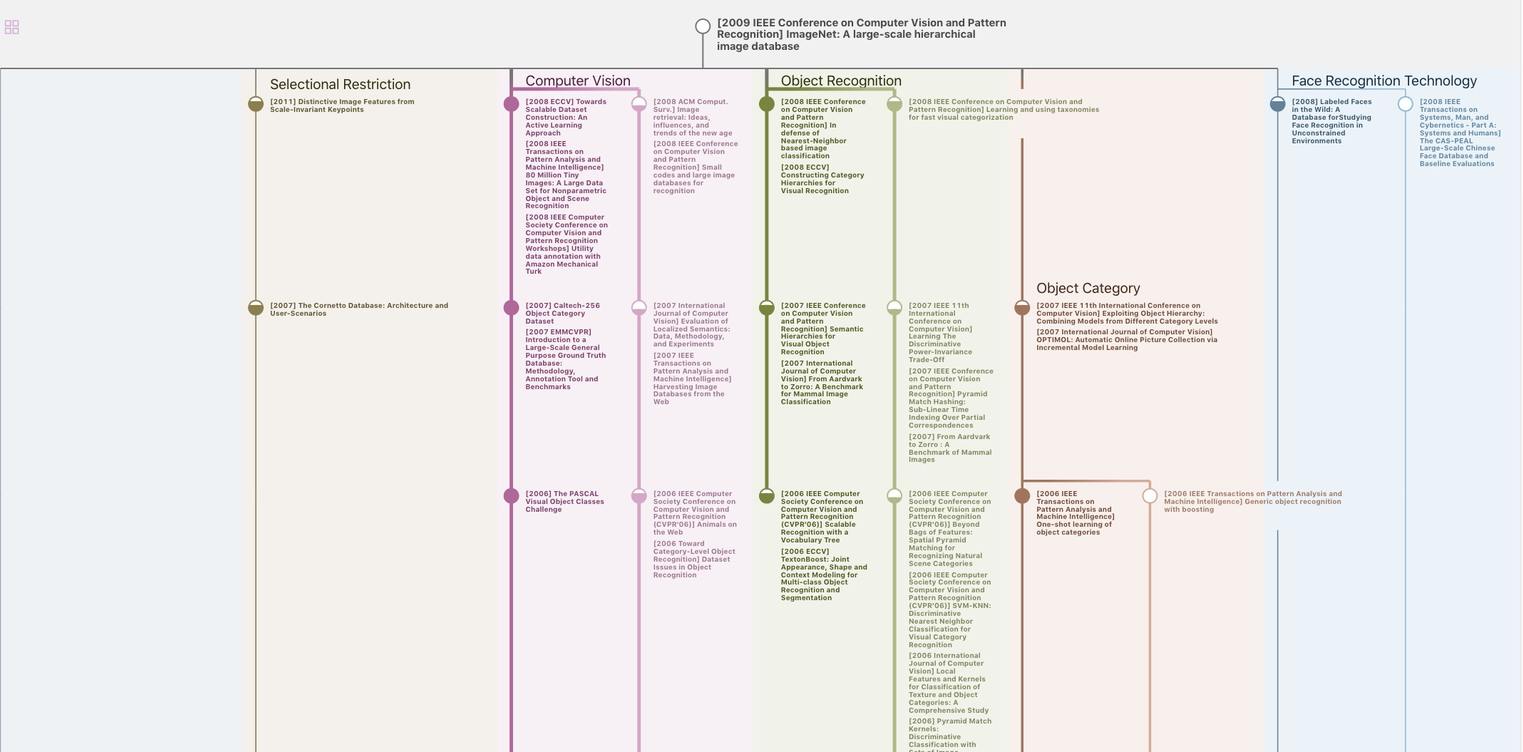
生成溯源树,研究论文发展脉络
Chat Paper
正在生成论文摘要