Adaptive Testing Using a General Diagnostic Model.
ADAPTIVE AND ADAPTABLE LEARNING, EC-TEL 2016(2016)
摘要
In online learning platforms such as MOOCs, computerized assessment needs to be optimized in order to prevent boredom and dropout of learners. Indeed, they should spend as little time as possible in tests and still receive valuable feedback. It is actually possible to reduce the number of questions for the same accuracy with computerized adaptive testing (CAT): asking the next question according to the past performance of the examinee. CAT algorithms are divided in two categories: summative CATs, that measure the level of examinees, and formative CATs, that provide feedback to the examinees at the end of the test by specifying which knowledge components need further work. In this paper, we formalize the problem of test-size reduction by predicting student performance, and propose a new hybrid CAT algorithm GenMA based on the general diagnostic model, that is both summative and formative. Using real datasets, we compare our model to popular CAT models and show that GenMA achieves better accuracy while using fewer questions than the existing models.
更多查看译文
关键词
General Diagnostic Model, Predicting Student Performance, Computerized Adaptive Testing (CAT), Reduced Testis Size, DINA Model
AI 理解论文
溯源树
样例
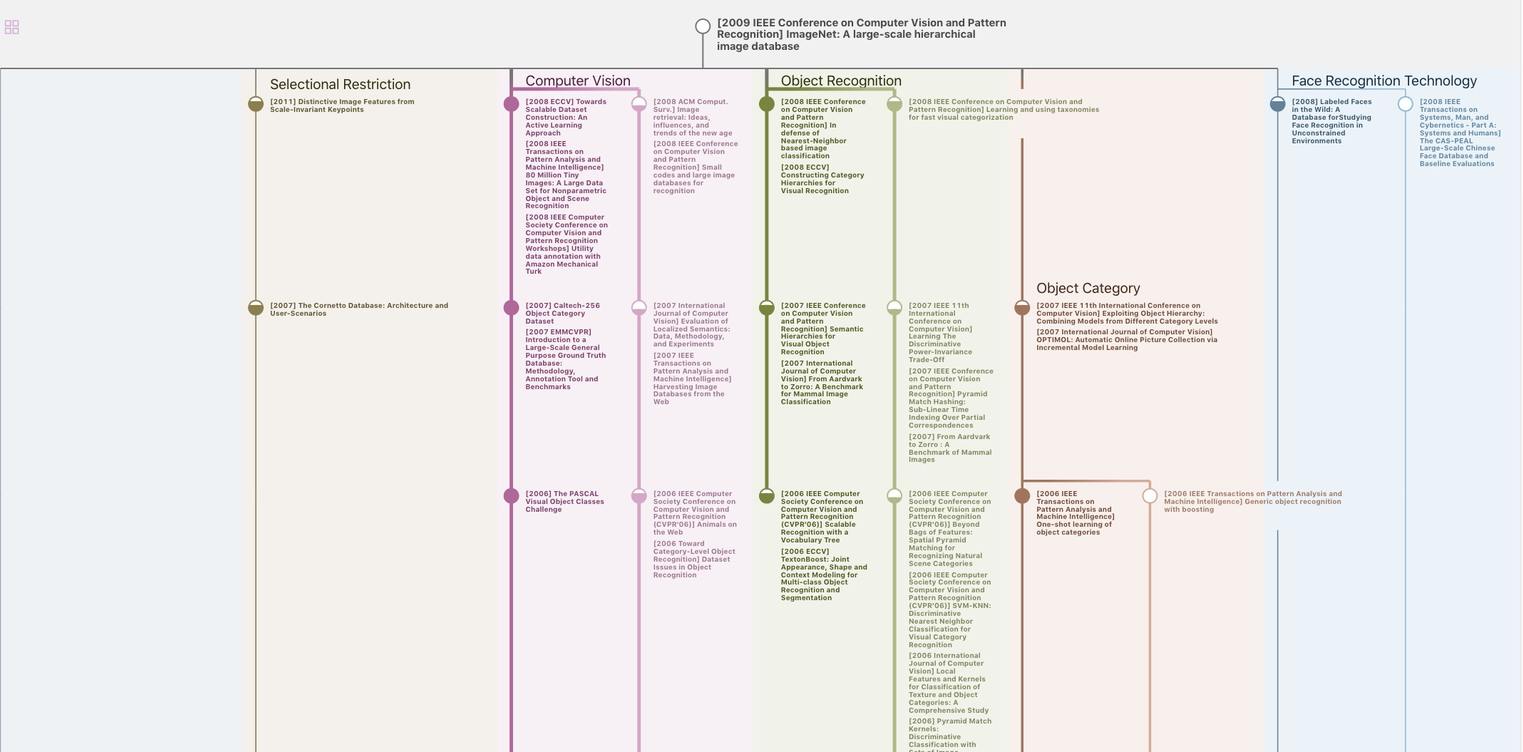
生成溯源树,研究论文发展脉络
Chat Paper
正在生成论文摘要