Parameter estimation for gene regulatory networks: a two-stage MCMC Bayesian approach.
2016 38th Annual International Conference of the IEEE Engineering in Medicine and Biology Society (EMBC)(2016)
摘要
Genetic regulatory networks have emerged as a useful way to elucidate the biochemical pathways for biological functions. Yet, determination of the exact parametric forms for these models remain a major challenge. In this paper, we present a novel computational approach implemented in C++ to solve this inverse problem. This takes the form of an optimization stage first after which Bayesian filtering takes place. The key advantage of such a flexible, general and robust approach is that it provides us with a joint probability distribution of the model parameters instead of single estimates, which we can propagate to final predictions. We apply these ideas to time series data from gene circuit models using state space representation. We show that unsound terms from a more generalized model can be efficiently pruned by our approach. We believe our work offers a new insight towards understanding the behaviour, mechanisms and thermodynamics of system biology.
更多查看译文
关键词
Algorithms,Bayes Theorem,Gene Regulatory Networks,Models, Theoretical,Probability
AI 理解论文
溯源树
样例
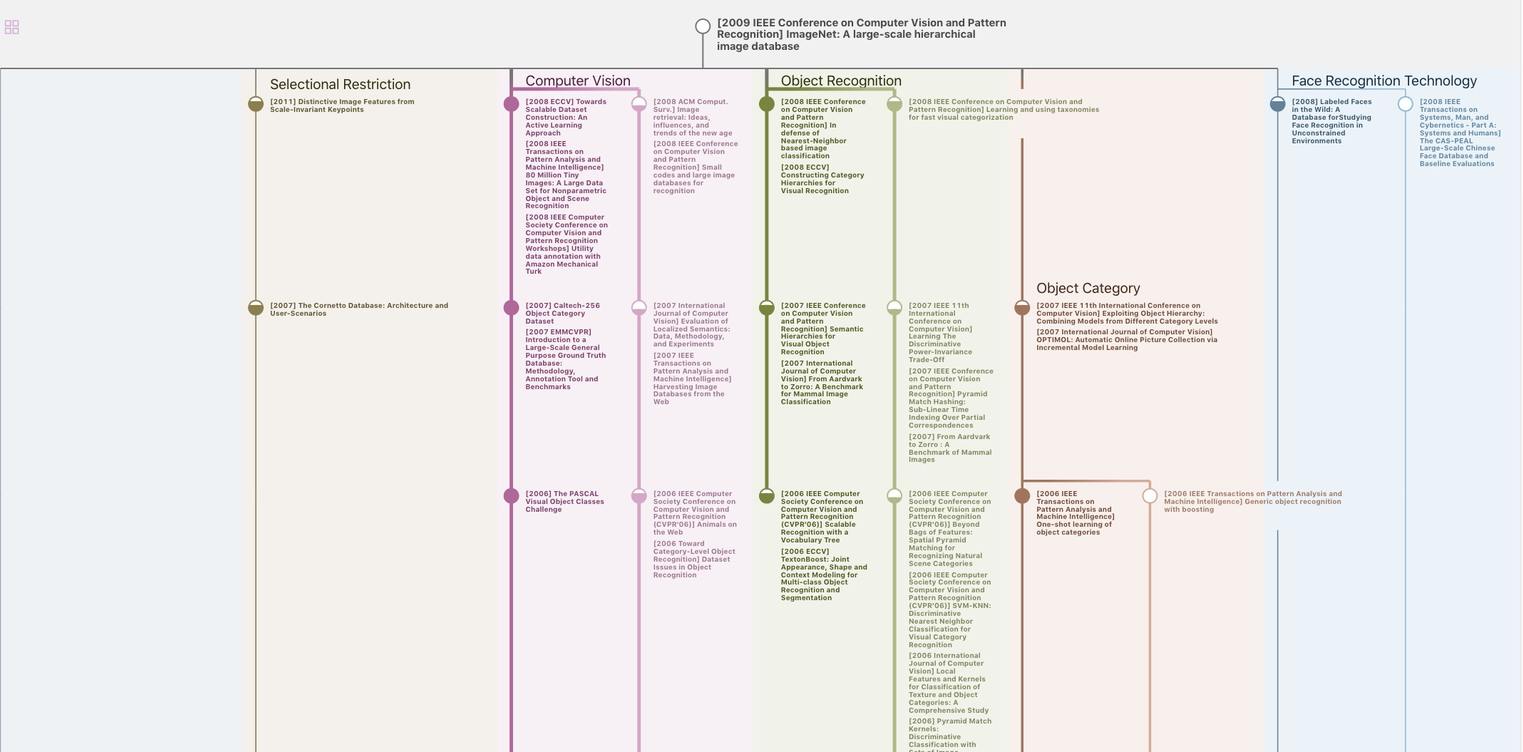
生成溯源树,研究论文发展脉络
Chat Paper
正在生成论文摘要