Sensor data quality processing for vital signs with opportunistic ambient sensing.
2016 38TH ANNUAL INTERNATIONAL CONFERENCE OF THE IEEE ENGINEERING IN MEDICINE AND BIOLOGY SOCIETY (EMBC)(2016)
摘要
Opportunistic ambient sensing involves placement of sensors appropriately so that intermittent contact can be made unobtrusively for gathering physiological signals for vital signs. In this paper, we discuss the results of our quality processing system used to extract heart rate from ballisto-cardiogram signals obtained from a micro-bending fiber optic sensor pressure mat. Visual inspection is used to label data into informative and non-informative classes based on their heart rate information. Five classifiers are employed for the classification process, i.e., random forest, support vector machine, multilayer, feedforward neural network, linear discriminant analysis, and decision tree. To compute the overall effectiveness of quality processing, the informative signals are processed to estimate interbeat intervals. The system was used to process, data collected from 50 human subjects sitting in a massage chair while performing different activities. Opportunistically collected data was obtained from the fiber optic sensor mat placed on the headrest of the massage chair. Using our classification approach, 57.37% of the dataset was able to provide informative signals. On the informative signals, random forest classifier achieves the best classification accuracy with a mean accuracy of 98.99%. The average of the mean absolute error between the estimated heart rate and the reference ECG is reduced from 13.2 to 8.47. Therefore, the proposed system shows a good robustness for opportunistic ambient sensing.
更多查看译文
关键词
Data Accuracy,Decision Trees,Discriminant Analysis,Heart Rate,Humans,Neural Networks (Computer),Support Vector Machine
AI 理解论文
溯源树
样例
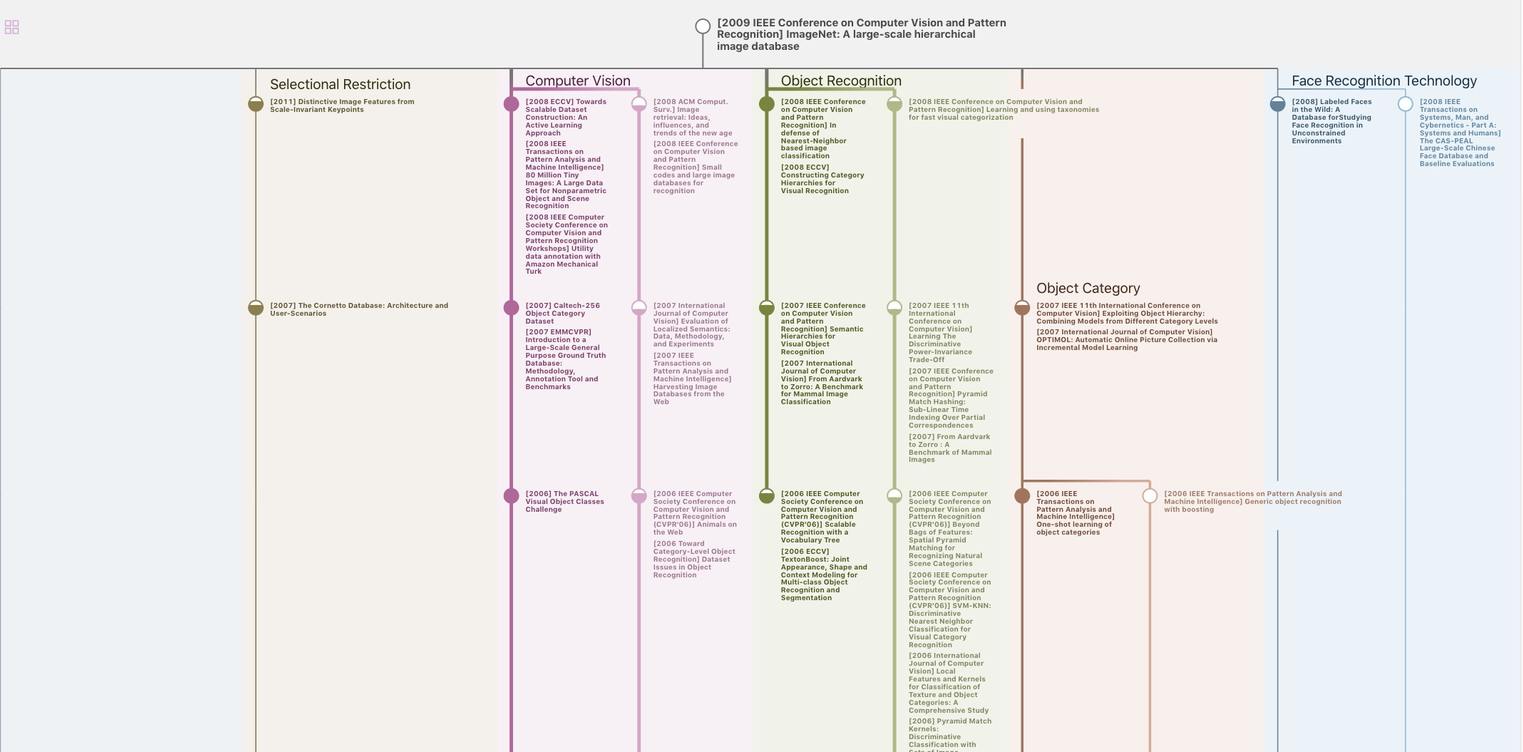
生成溯源树,研究论文发展脉络
Chat Paper
正在生成论文摘要