Binding affinity prediction of S. cerevisiae 14-3-3 and GYF peptide-recognition domains using support vector regression.
2016 38th Annual International Conference of the IEEE Engineering in Medicine and Biology Society (EMBC)(2016)
摘要
Proteins interact with other proteins and bio-molecules to carry out biological processes in a cell. Computational models help understanding complex biochemical processes that happens throughout the life of a cell. Domain-mediated protein interaction to peptides one such complex problem in bioinformatics that requires computational predictive models to identify meaningful bindings. In this study, domain-peptide binding affinity prediction models are proposed based on support vector regression. Proposed models are applied to yeast bmh 14-3-3 and syh GYF peptide-recognition domains. The cross validated results of the domain-peptide binding affinity data sets show that predictive performance of the support vector based models are efficient.
更多查看译文
关键词
14-3-3 Proteins,Computational Biology,Databases, Protein,Peptides,Protein Binding,Protein Domains,Saccharomyces cerevisiae Proteins,Support Vector Machine
AI 理解论文
溯源树
样例
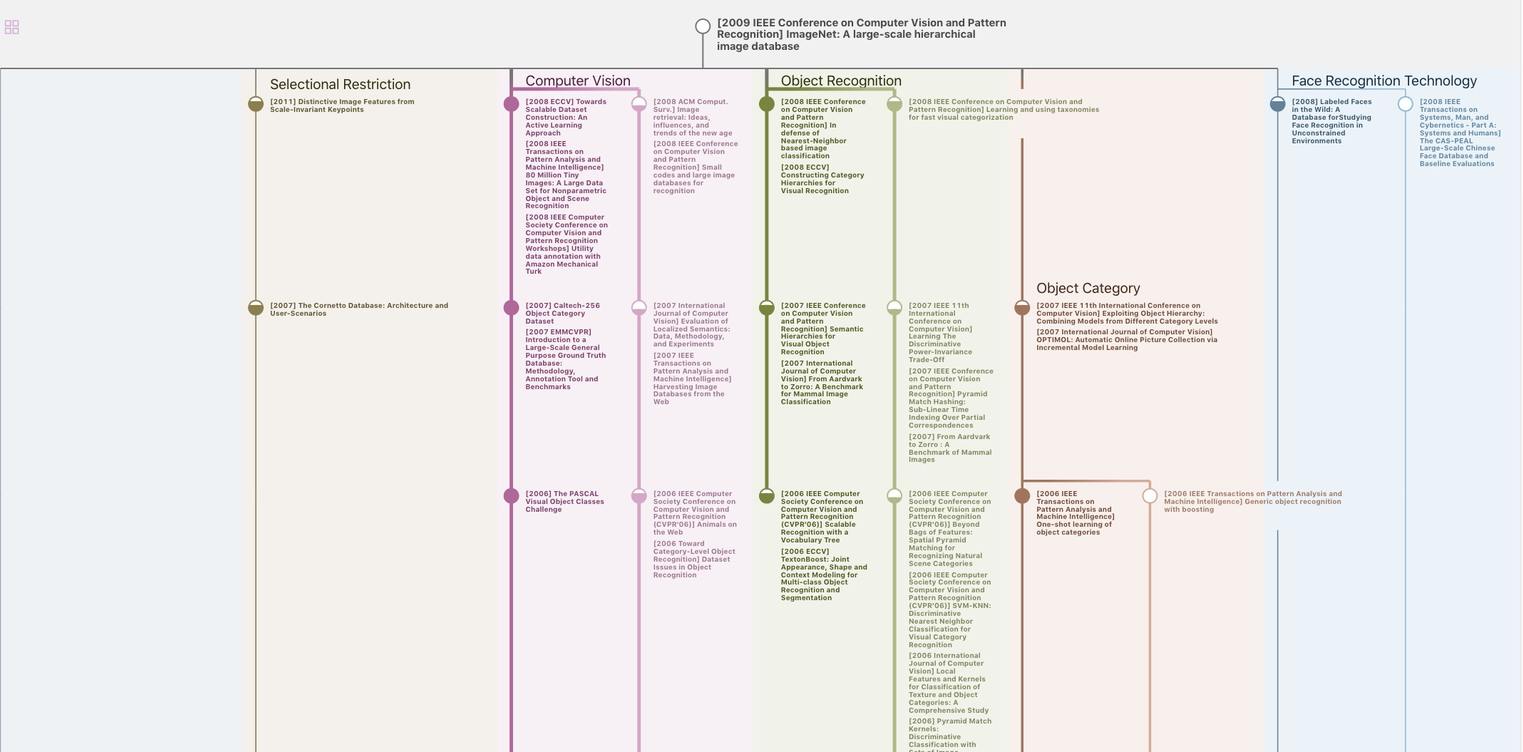
生成溯源树,研究论文发展脉络
Chat Paper
正在生成论文摘要