Fuzzy Clustering Based Traffic Pattern Identification
2016 IEEE International Conference on Fuzzy Systems (FUZZ-IEEE)(2016)
摘要
Automatic anomaly detection is of great importance in the big data era because the large volume of raw data can be accessed easily and the automatic method to analyze the data is desirable. This paper uses a framework based on fuzzy c-means clustering to detect anomaly in temporal traffic data. In this framework the sliding window is employed first to generate a collection of segments or subsequences of the time series. Then the fuzzy clustering is applied on those segments to reveal the outliers or abnormal segments in the series. The abnormal score for each segment is calculated according to the clustering results. To obtain the best setting of parameters and more meaningful abnormal scores, we design one novel performance index. The proposed approach is tested on the temporal traffic data set collected from Beijing, China, and the results demonstrate that the proposed approach can identify many valuable traffic patterns in the data.
更多查看译文
关键词
fuzzy clustering based traffic pattern identification,automatic anomaly detection,Big Data,automatic data analysis,fuzzy c-means clustering,temporal traffic data anomaly detection,sliding window,time series,abnormal score calculation,performance index,Beijing,China
AI 理解论文
溯源树
样例
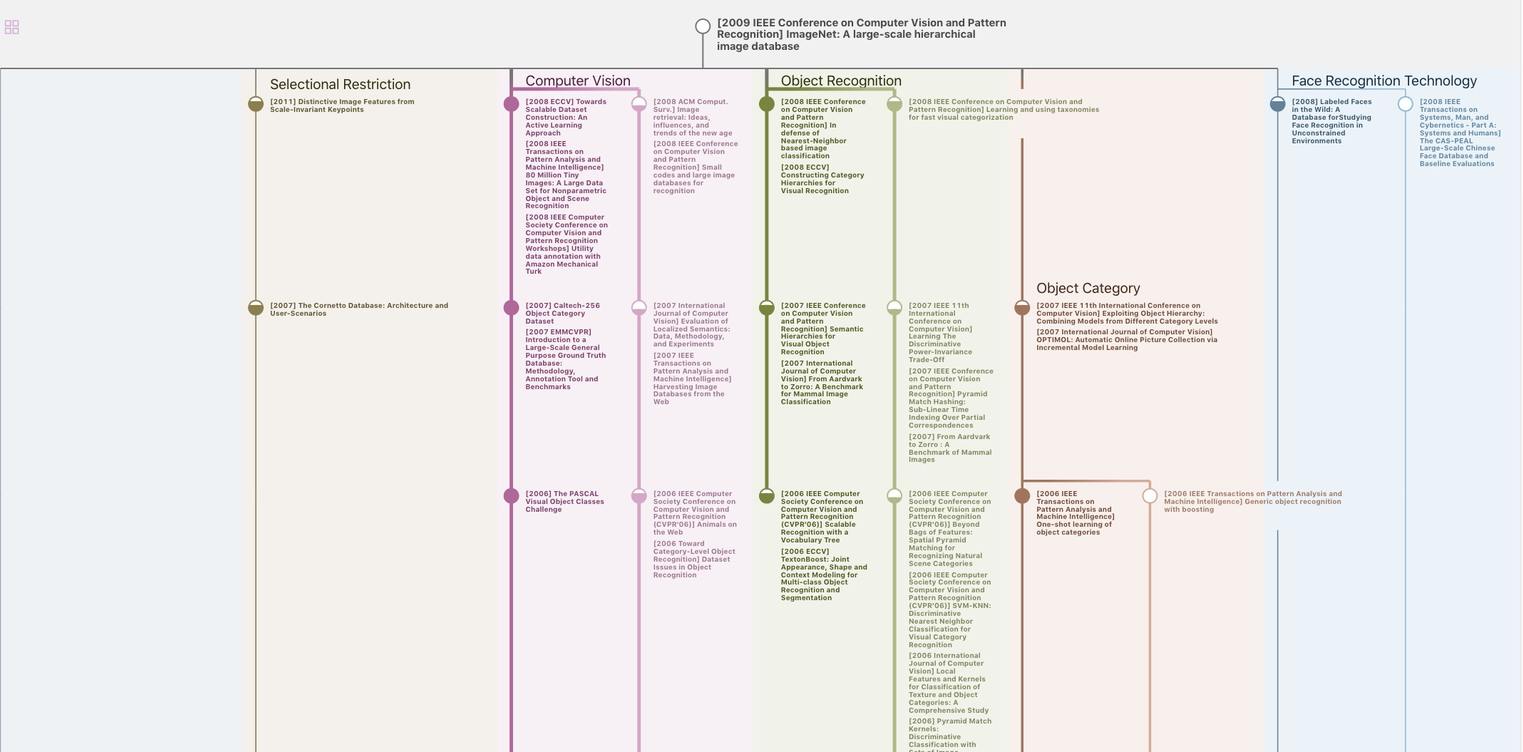
生成溯源树,研究论文发展脉络
Chat Paper
正在生成论文摘要