Tackling Label Noise With Multi-Class Decomposition Using Fuzzy One-Class Support Vector Machines
2016 IEEE International Conference on Fuzzy Systems (FUZZ-IEEE)(2016)
摘要
Class label noise is a data-level difficulty associated with training objects with incorrectly assigned labels. This problem may originate from poorly documented historic data, errors during data generation process or mistakes made by human experts. Inclusion of such examples during the training process will mislead the classifier by presenting a falsified class distribution and consequently lead to degradation of models' generalization abilities. This phenomenon becomes even more troublesome in multi-class scenarios that may be affected by highly complex intra-class noise. Decomposition strategies with binary classifiers were proven to alleviate this difficulty by using simplified binary subtasks that are less affected by the noise. In this paper we propose to extend this approach by using the one-class classification decomposition. In this scenario each class has assigned individual one-class classifier that aims at capturing its distinguishing characteristics. This allows to create a robust data description and then apply a dedicated classifier combination in order to reconstruct the original multi-class task. We further extend this concept by using fuzzy one-class classifiers that allow to associate membership values with each training objects. This allows us to reduce the influence of uncertain and potentially noisy samples on the shape of learned decision boundary. Experimental study backed-up with statistical analysis shows that fuzzy one-class classifier decomposition offers an excellent robustness to noise in multi-class classification.
更多查看译文
关键词
machine learning,label noise,one-class classification,multi-class decomposition,fuzzy classifiers data uncertainty
AI 理解论文
溯源树
样例
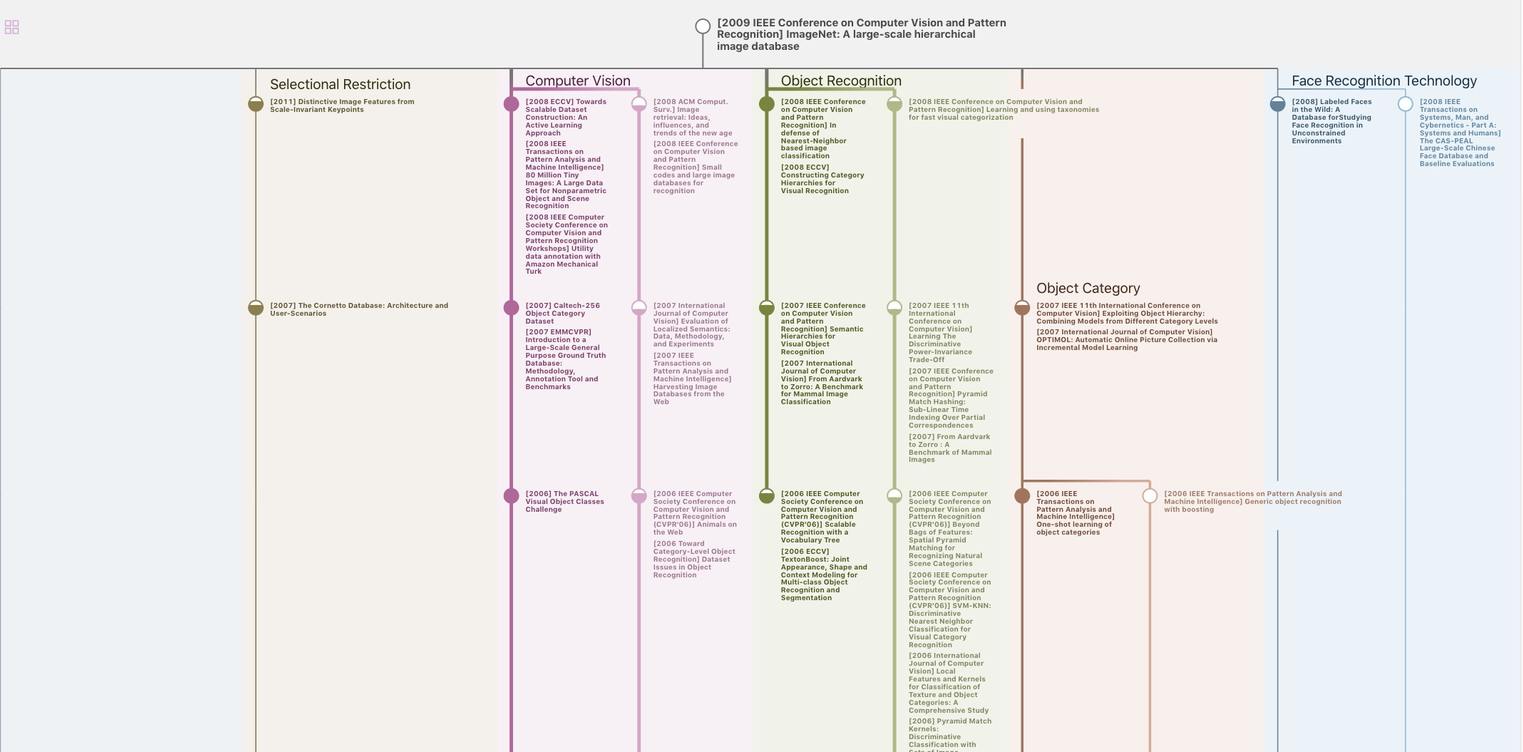
生成溯源树,研究论文发展脉络
Chat Paper
正在生成论文摘要