A Weakly Supervised Activity Recognition Framework For Real-Time Synthetic Biology Laboratory Assistance
UBICOMP(2016)
摘要
We describe the design of a hybrid system - a combination of a Dynamic Graphical Model (DGM) with a Deep Neural Network (DNN) - to identify activities performed during synthetic biology experiments. The purpose is to provide real-time feedback to experimenters, thus helping to reduce human errors and improve experimental reproducibility. The data consists of unlabeled videos of recorded experiments and "weakly supervised" information (i.e., "theoretical" and asynchronous knowledge of sets of high level activity sequences in the experiment) used to train the system. Multiple activity sequences are modeled using a trellis, and deep features are extracted from video images. Model performance is accessed using real-time online statistical inference. The trellis incorporates variations during experiment execution, making our model very general and capable of high performance.
更多查看译文
关键词
Activity Recognition,Dynamic Graphical Models,Deep Learning,Synthetic Biology
AI 理解论文
溯源树
样例
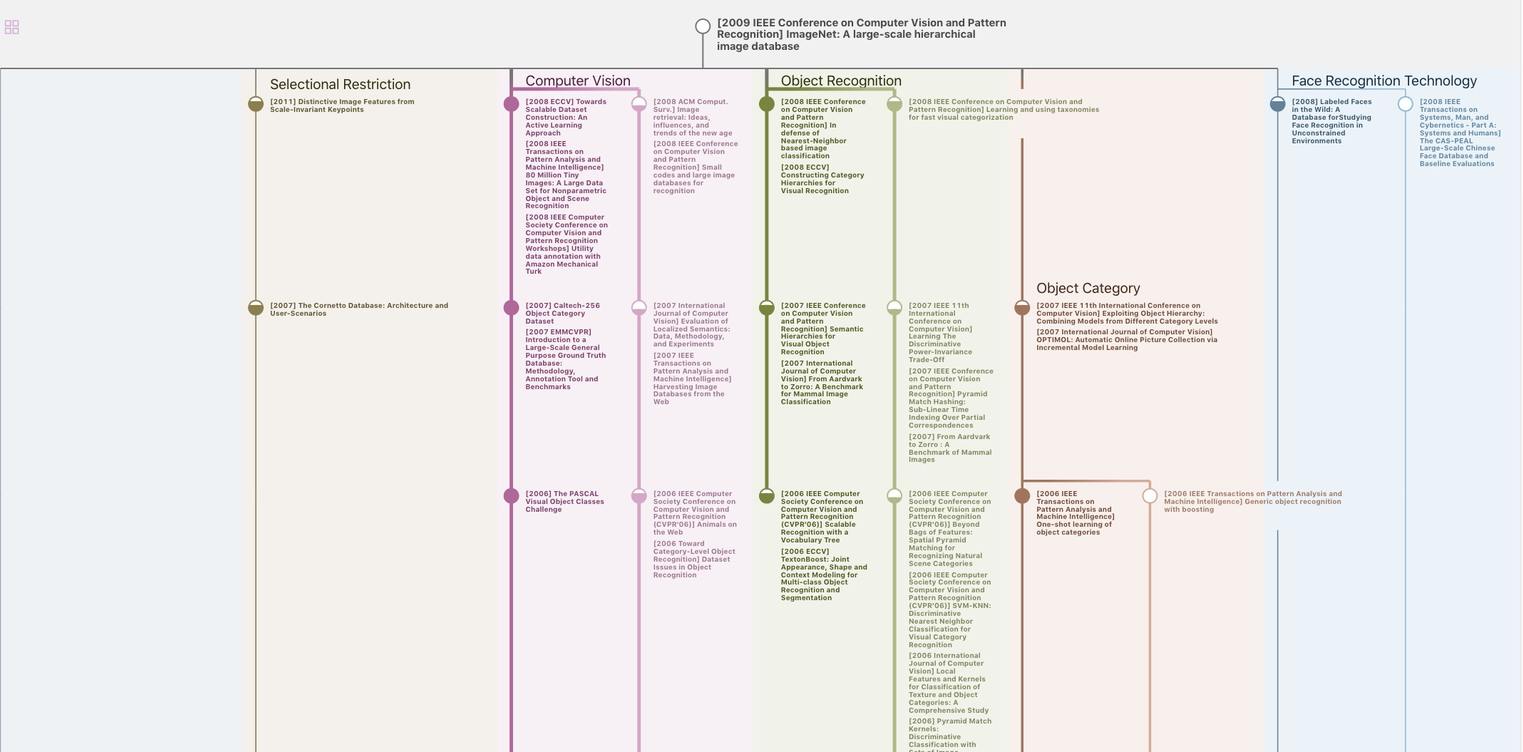
生成溯源树,研究论文发展脉络
Chat Paper
正在生成论文摘要