Convolutional Neural Network For 3d Object Recognition Using Volumetric Representation
2016 FIRST INTERNATIONAL WORKSHOP ON SENSING, PROCESSING AND LEARNING FOR INTELLIGENT MACHINES (SPLINE)(2016)
摘要
Following the success of Convolutional Neural Networks (CNNs) on object recognition using 2D images, they are extended in this paper to process 3D data. Nearly most of current systems require huge amount of computation for dealing with large amount of data. In this paper, an efficient 3D volumetric object representation, Volumetric Accelerator (VOLA), is presented which requires much less memory than the normal volumetric representations. On this basis, a few 3D digit datasets using 2D MNIST and 2D digit fonts with different rotations along the x, y, and z axis are introduced. Finally, we introduce a combination of multiple CNN models based on the famous LeNet model. The trained CNN models based on the generated dataset have achieved the average accuracy of 90.30% and 81.85% for 3D-MNIST and 3D-Fonts datasets, respectively. Experimental results show that VOLA-based CNNs perform 1.5x faster than the original LeNet.
更多查看译文
关键词
convolutional neural network,3D object recognition,3D volumetric object representation,volumetric accelerator,3D digit datasets,2D MNIST dataset,LeNet model,VOLA-based CNN
AI 理解论文
溯源树
样例
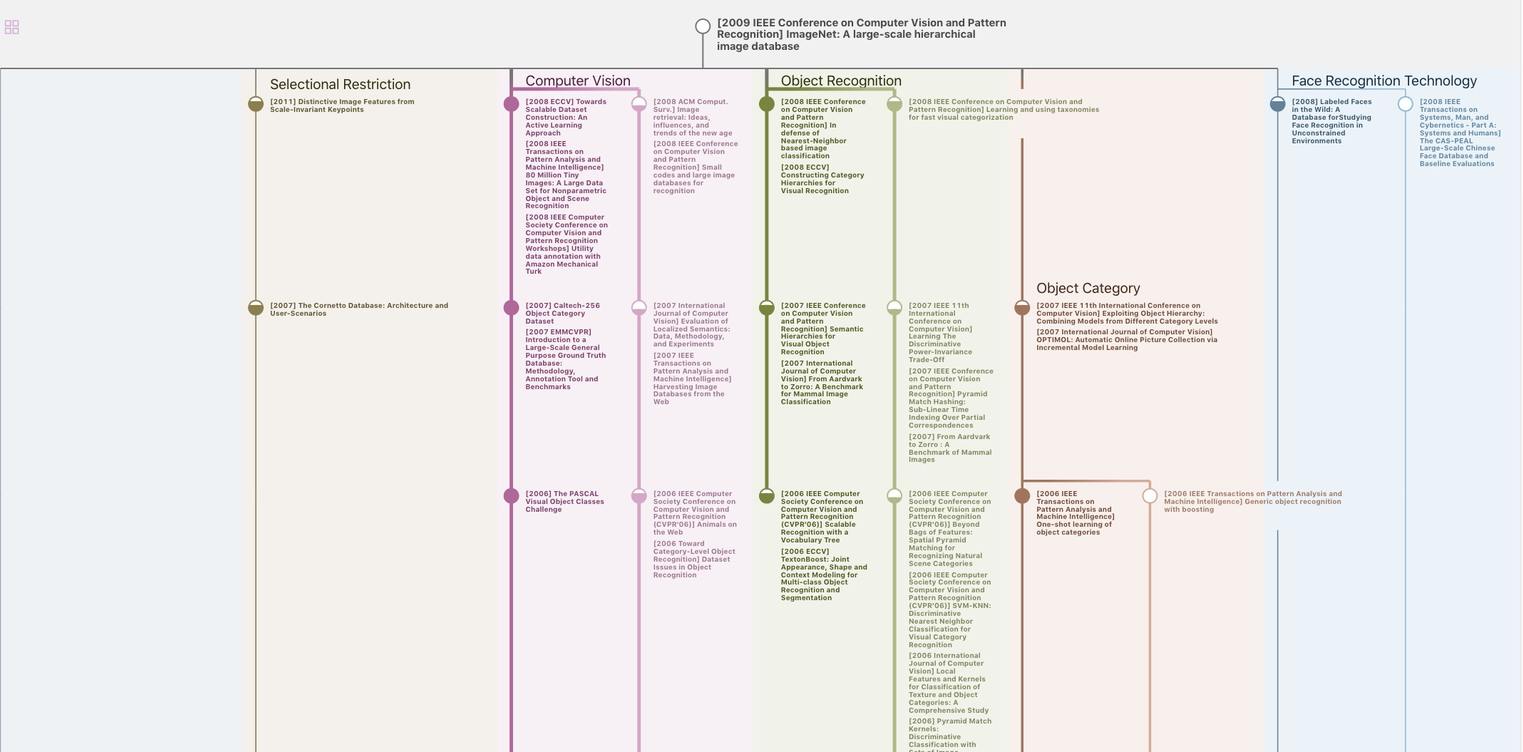
生成溯源树,研究论文发展脉络
Chat Paper
正在生成论文摘要