Adaptive, Personalized Diversity for Visual Discovery
RECSYS(2018)
摘要
Search queries are appropriate when users have explicit intent, but they perform poorly when the intent is difficult to express or if the user is simply looking to be inspired. Visual browsing systems allow e-commerce platforms to address these scenarios while offering the user an engaging shopping experience. Here we explore extensions in the direction of adaptive personalization and item diversification within Stream, a new form of visual browsing and discovery by Amazon. Our system presents the user with a diverse set of interesting items while adapting to user interactions. Our solution consists of three components (1) a Bayesian regression model for scoring the relevance of items while leveraging uncertainty, (2) a submodular diversification framework that re-ranks the top scoring items based on category, and (3) personalized category preferences learned from the user's behavior. When tested on live traffic, our algorithms show a strong lift in click-through-rate and session duration.
更多查看译文
关键词
Machine Learning,Submodular Functions,Diversity,Personalization,Explore-Exploit,Multi-Armed Bandits
AI 理解论文
溯源树
样例
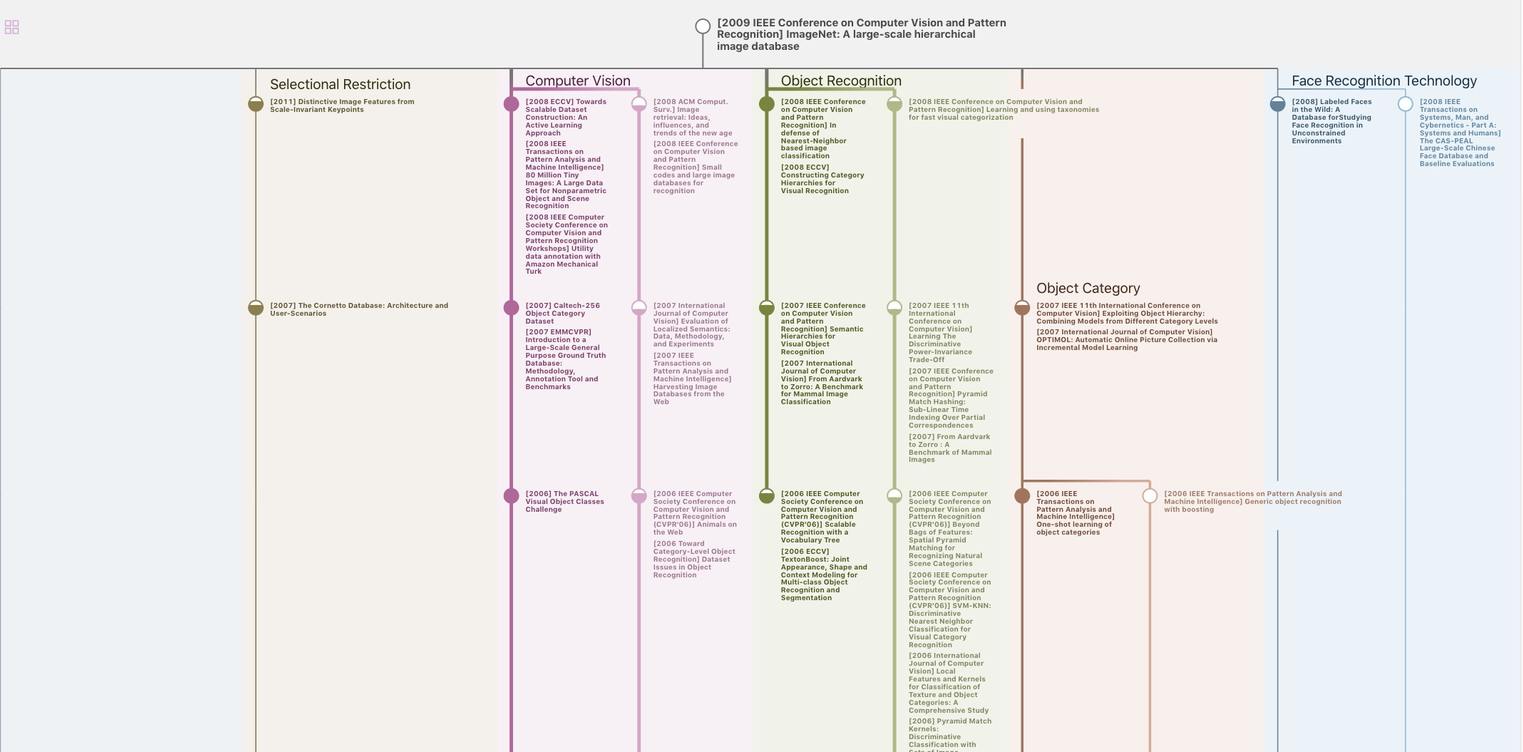
生成溯源树,研究论文发展脉络
Chat Paper
正在生成论文摘要