Tree Height Estimates In Boreal Forest Using Gaussian Process Regression
2016 IEEE International Geoscience and Remote Sensing Symposium (IGARSS)(2016)
摘要
Tree height is one of the continuous essential climate model variables. This work applies Gaussian process regression to tree height estimation. Estimates are produced in a coniferous boreal forest area in Southern Finland. GP regression produces both estimate and predictive variance for each pixel. Results show that Gaussian process regression produces estimates which are as good as the ones produced by existing methods. The root-mean-square error from GP regression is lower, estimates have less bias and maximum value is closer to the actual maximum. Predictions can be applied and ingested to carbon modelling where estimates are either direct input parameters or they serve for validation the parameters computed by the models.
更多查看译文
关键词
Boreal forest,Tree height,Pixel-level uncertainty,Gaussian process,Regression
AI 理解论文
溯源树
样例
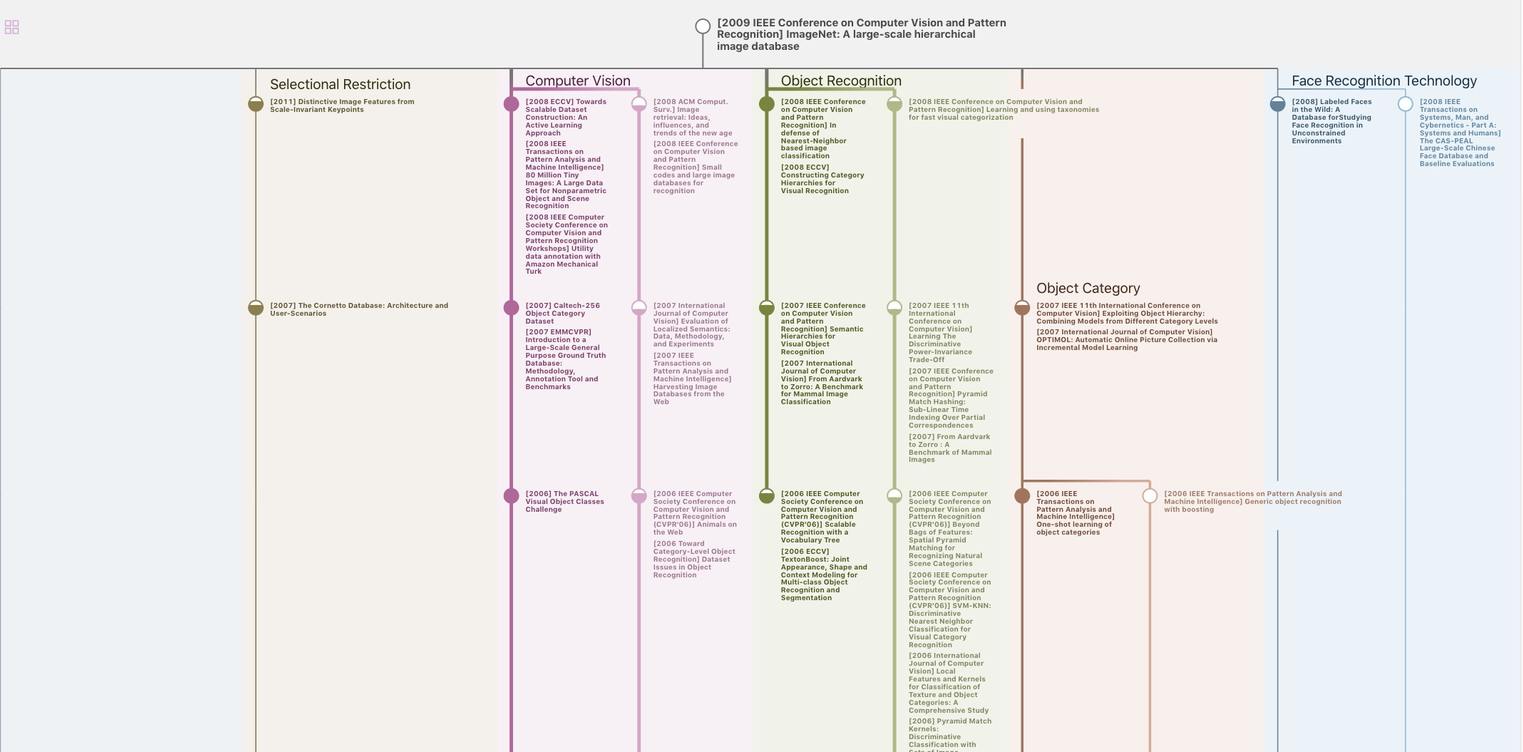
生成溯源树,研究论文发展脉络
Chat Paper
正在生成论文摘要