Variational inference for medical image segmentation.
Computer Vision and Image Understanding(2016)
摘要
We present a generalisation of the brain segmentation algorithm implemented in the SPM software, which exploits variational Bayesian inferenceWe test the accuracy and robustness of our method in segmenting brain tissues using synthetic and real MRI dataWe introduce an empirical Bayes framework to learn tissue specific intensity priors from large data sets Variational inference techniques are powerful methods for learning probabilistic models and provide significant advantages over maximum likelihood (ML) or maximum a posteriori (MAP) approaches. Nevertheless they have not yet been fully exploited for image processing applications. In this paper we present a variational Bayes (VB) approach for image segmentation. We aim to show that VB provides a framework for generalising existing segmentation algorithms that rely on an expectation-maximisation formulation, while increasing their robustness and computational stability. We also show how optimal model complexity can be automatically determined in a variational setting, as opposed to ML frameworks which are intrinsically prone to overfitting. Finally, we demonstrate how suitable intensity priors, that can be used in combination with the presented algorithm, can be learned from large imaging data sets by adopting an empirical Bayes approach.
更多查看译文
关键词
Image segmentation,Bayesian inference,Variational Bayes,Neuroimaging,MRI
AI 理解论文
溯源树
样例
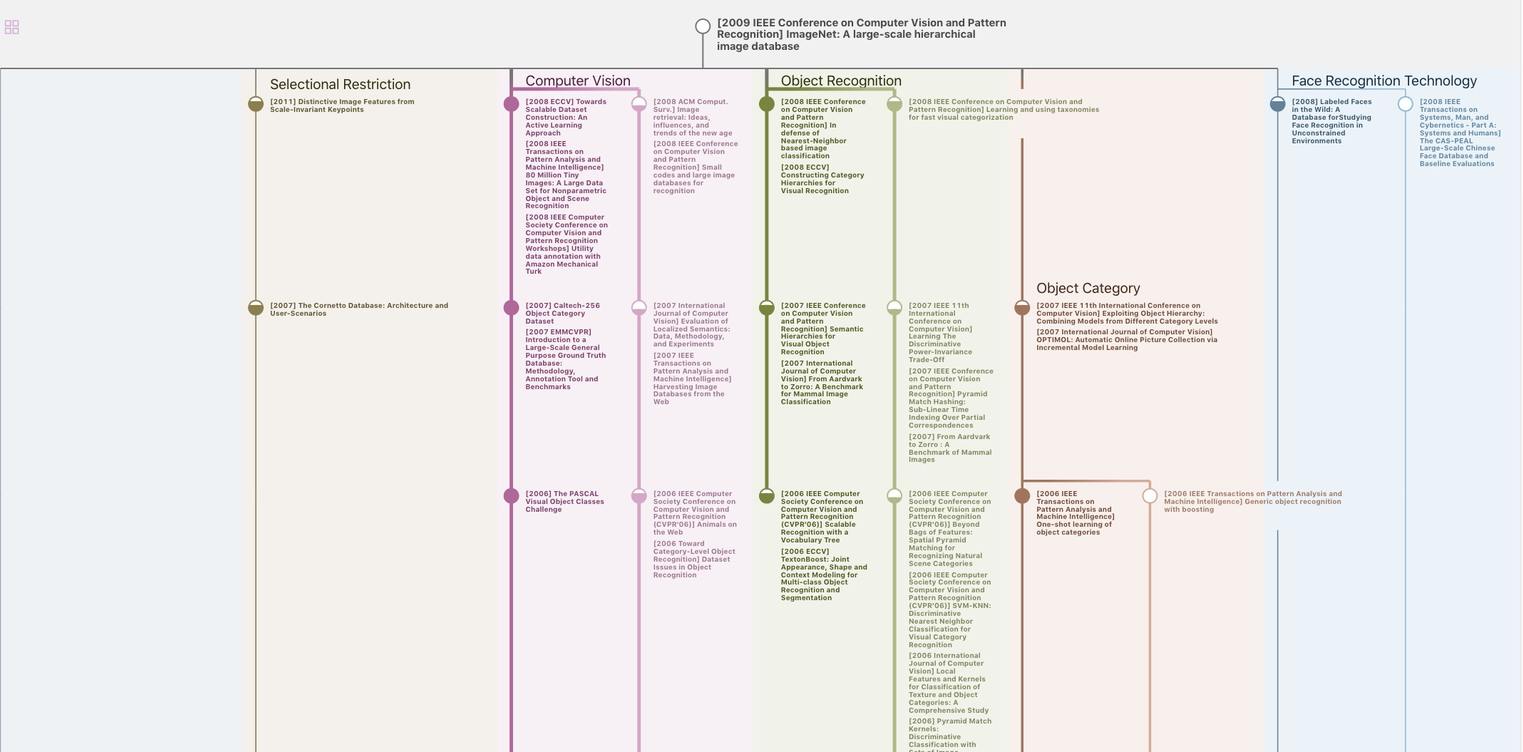
生成溯源树,研究论文发展脉络
Chat Paper
正在生成论文摘要