Porcine Lie Detectors: Automatic Quantification Of Posture State And Transitions In Sows Using Inertial Sensors
Computers and electronics in agriculture(2016)
摘要
This paper presents a novel approach to automated classification and quantification of sow postures and posture transitions that may enable large scale and accurate continuous behaviour assessment on farm. Automatic classification and quantification of postures and posture transitions in domestic animals has substantial potential to enhance their welfare and productivity. Analysis of such behaviours in farrowing sows can highlight the need for human intervention or lead to the prediction of movement patterns that are potentially dangerous for their piglets, such as crushing when the sow lies down. Data were recorded by a tri-axial accelerometer secured to the hind-end of each sow, in a deployment that involved six sows over the period around parturition. The posture state (standing, sitting, lateral and sternal lying) was automatically classified for the full dataset with a mean F-1 score (a measure of predictive performance between 0 and 1) of 0.78. Sitting was shown to present a greater challenge to classification with a F-1 score of 0.54, compared to the lateral lying postures, which were classified with an average F-1 score of 0.91. Posture transitions were detected with a F-1 score of 0.79. We automatically extracted and visualized a range of features that characterise the manner in which the sows changed posture in order to provide comparative descriptors of sow activity and lying style that can be used to assess the influence of genetics or housing design. The methodology presented in this paper can be applied in large scale deployments with potential for enhancing animal welfare and productivity on farm. (C) 2016 The Authors. Published by Elsevier B.V.
更多查看译文
关键词
Accelerometer,Farrowing,Activity recognition,Support Vector Machine,Computational behaviour assessment
AI 理解论文
溯源树
样例
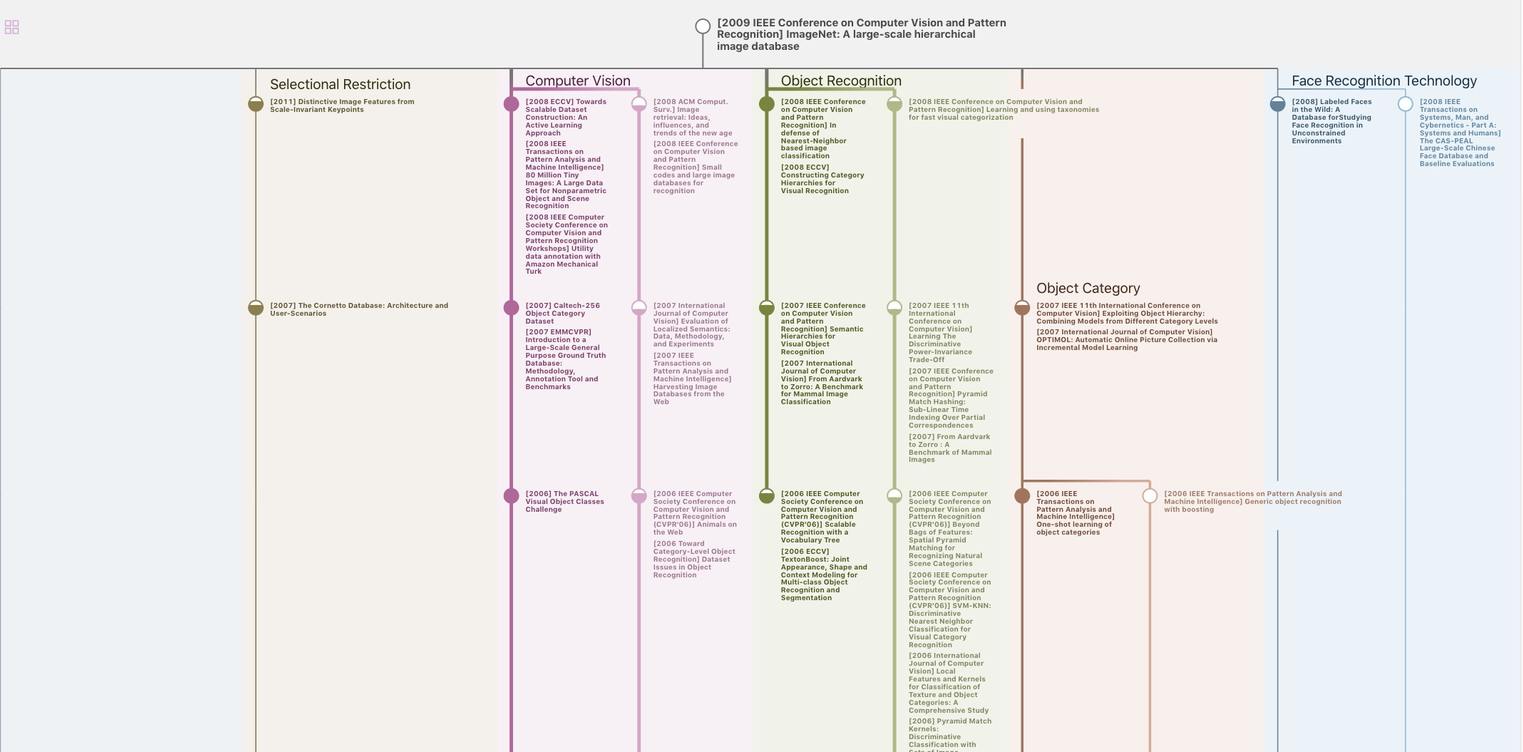
生成溯源树,研究论文发展脉络
Chat Paper
正在生成论文摘要