Identifying Style of 3D Shapes using Deep Metric Learning.
Comput. Graph. Forum(2016)
摘要
We present a method that expands on previous work in learning human perceived style similarity across objects with different structures and functionalities. Unlike previous approaches that tackle this problem with the help of hand-crafted geometric descriptors, we make use of recent advances in metric learning with neural networks deep metric learning. This allows us to train the similarity metric on a shape collection directly, since any low- or high-level features needed to discriminate between different styles are identified by the neural network automatically. Furthermore, we avoid the issue of finding and comparing sub-elements of the shapes. We represent the shapes as rendered images and show how image tuples can be selected, generated and used efficiently for deep metric learning. We also tackle the problem of training our neural networks on relatively small datasets and show that we achieve style classification accuracy competitive with the state of the art. Finally, to reduce annotation effort we propose a method to incorporate heterogeneous data sources by adding annotated photos found online in order to expand or supplant parts of our training data.
更多查看译文
关键词
Categories and Subject Descriptors (according to ACM CCS),I,3,5 [Computer Graphics]: Computational Geometry and Object Modeling,style similarity,deep metric learning
AI 理解论文
溯源树
样例
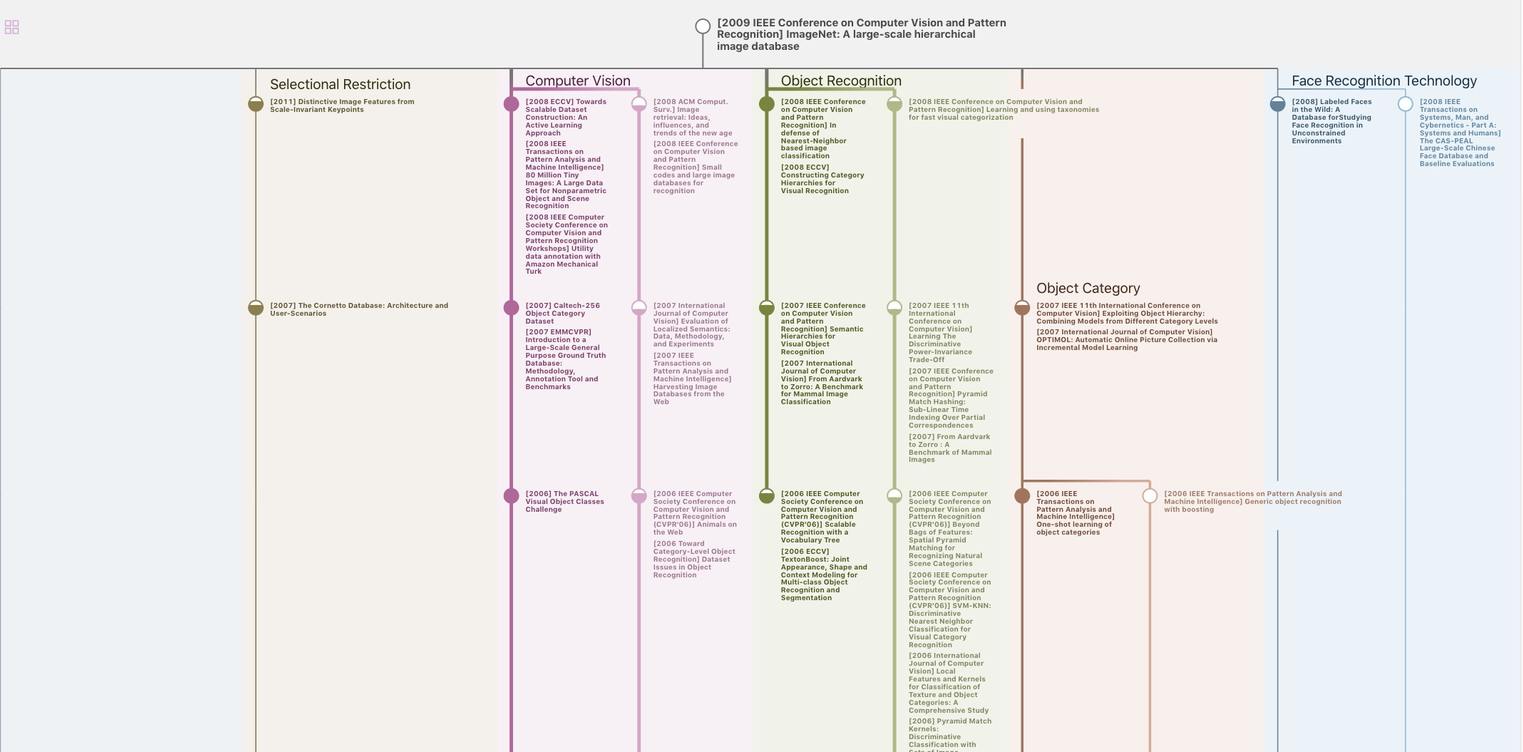
生成溯源树,研究论文发展脉络
Chat Paper
正在生成论文摘要