Learning in games via reinforcement and regularization
Mathematics of Operations Research(2016)
摘要
We investigate a class of reinforcement learning dynamics where players adjust their strategies based on their actions' cumulative payoffs over time - specifically, by playing mixed strategies that maximize their expected cumulative payoff minus a regularization term. A widely studied example is exponential reinforcement learning, a process induced by an entropic regularization term which leads mixed strategies to evolve according to the replicator dynamics. However, in contrast to the class of regularization functions used to define smooth best responses in models of stochastic fictitious play, the functions used in this paper need not be infinitely steep at the boundary of the simplex; in fact, dropping this requirement gives rise to an important dichotomy between steep and nonsteep cases. In this general framework, we extend several properties of exponential learning, including the elimination of dominated strategies, the asymptotic stability of strict Nash equilibria, and the convergence of time-averaged trajectories in zero-sum games with an interior Nash equilibrium.
更多查看译文
关键词
Bregman divergence,dominated strategies,equilibrium stability,Fenchel coupling,penalty functions,projection dynamics,regularization,reinforcement learning,replicator dynamics,time averages
AI 理解论文
溯源树
样例
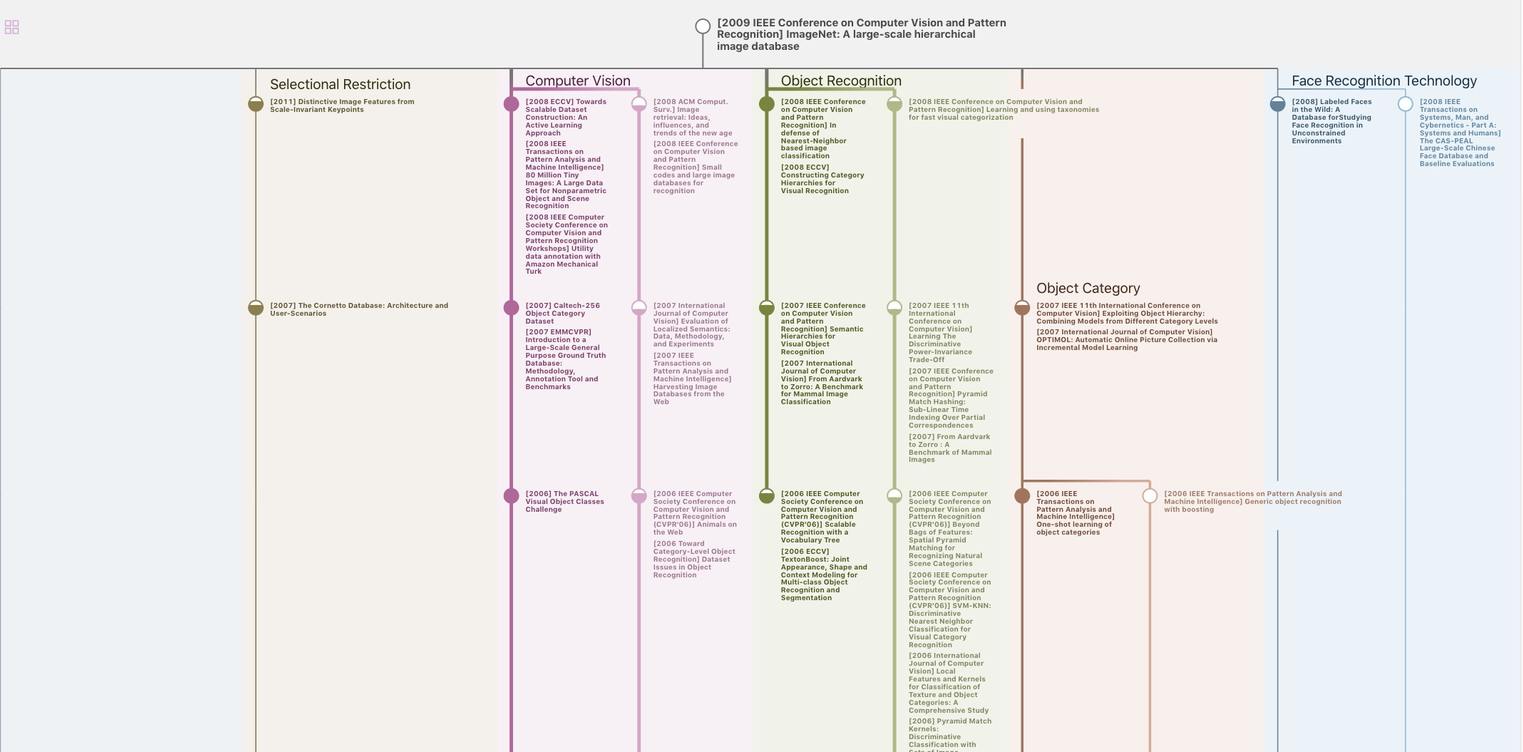
生成溯源树,研究论文发展脉络
Chat Paper
正在生成论文摘要