Making machine learning useable by revealing internal states update - a transparent approach
Periodicals(2016)
摘要
AbstractMachine learning ML techniques are often found difficult to apply effectively in practice because of their complexities. Therefore, making ML useable is emerging as one of active research fields recently. Furthermore, an ML algorithm is still a 'black-box'. This 'black-box' approach makes it difficult for users to understand complicated ML models. As a result, the user is uncertain about the usefulness of ML results and this affects the effectiveness of ML methods. This paper focuses on making a 'black-box' ML process transparent by presenting real-time internal status update of the ML process to users explicitly. A user study was performed to investigate the impact of revealing internal status update to users on the easiness of understanding data analysis process, meaningfulness of real-time status update, and convincingness of ML results. The study showed that revealing of the internal states of ML process can help improve easiness of understanding the data analysis process, make real-time status update more meaningful, and make ML results more convincing.
更多查看译文
关键词
machine learning, black-box, transparent, internal status update
AI 理解论文
溯源树
样例
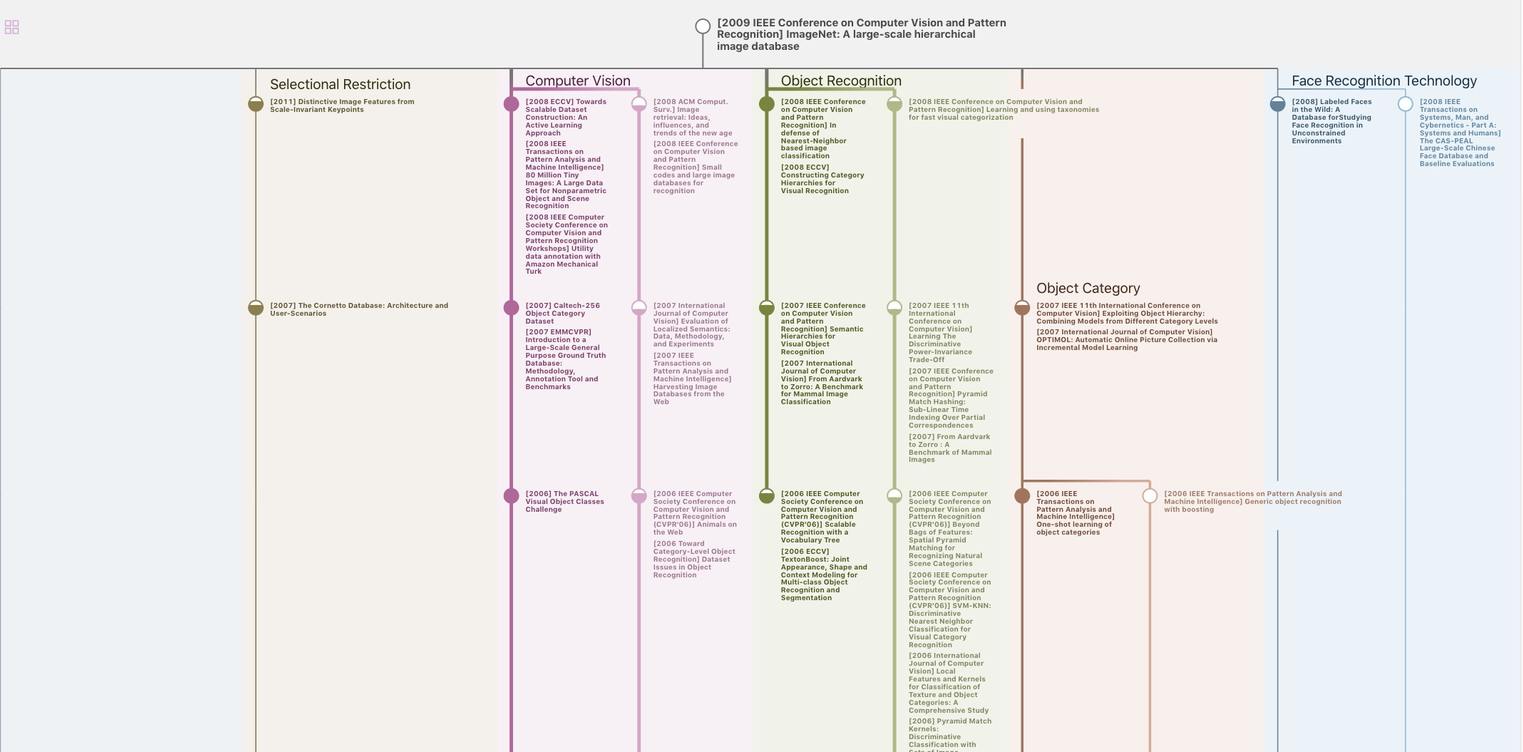
生成溯源树,研究论文发展脉络
Chat Paper
正在生成论文摘要