An Ensemble Blocking Scheme for Entity Resolution of Large and Sparse Datasets.
arXiv: Artificial Intelligence(2016)
摘要
Entity Resolution, also called record linkage or deduplication, refers to the process of identifying and merging duplicate versions of the same entity into a unified representation. The standard practice is to use a Rule based or Machine Learning based model that compares entity pairs and assigns a score to represent the pairsu0027 Match/Non-Match status. However, performing an exhaustive pair-wise comparison on all pairs of records leads to quadratic matcher complexity and hence a Blocking step is performed before the Matching to group similar entities into smaller blocks that the matcher can then examine exhaustively. Several blocking schemes have been developed to efficiently and effectively block the input dataset into manageable groups. At CareerBuilder (CB), we perform deduplication on massive datasets of people profiles collected from disparate sources with varying informational content. We observed that, employing a single blocking technique did not cover the base for all possible scenarios due to the multi-faceted nature of our data sources. In this paper, we describe our ensemble approach to blocking that combines two different blocking techniques to leverage their respective strengths.
更多查看译文
AI 理解论文
溯源树
样例
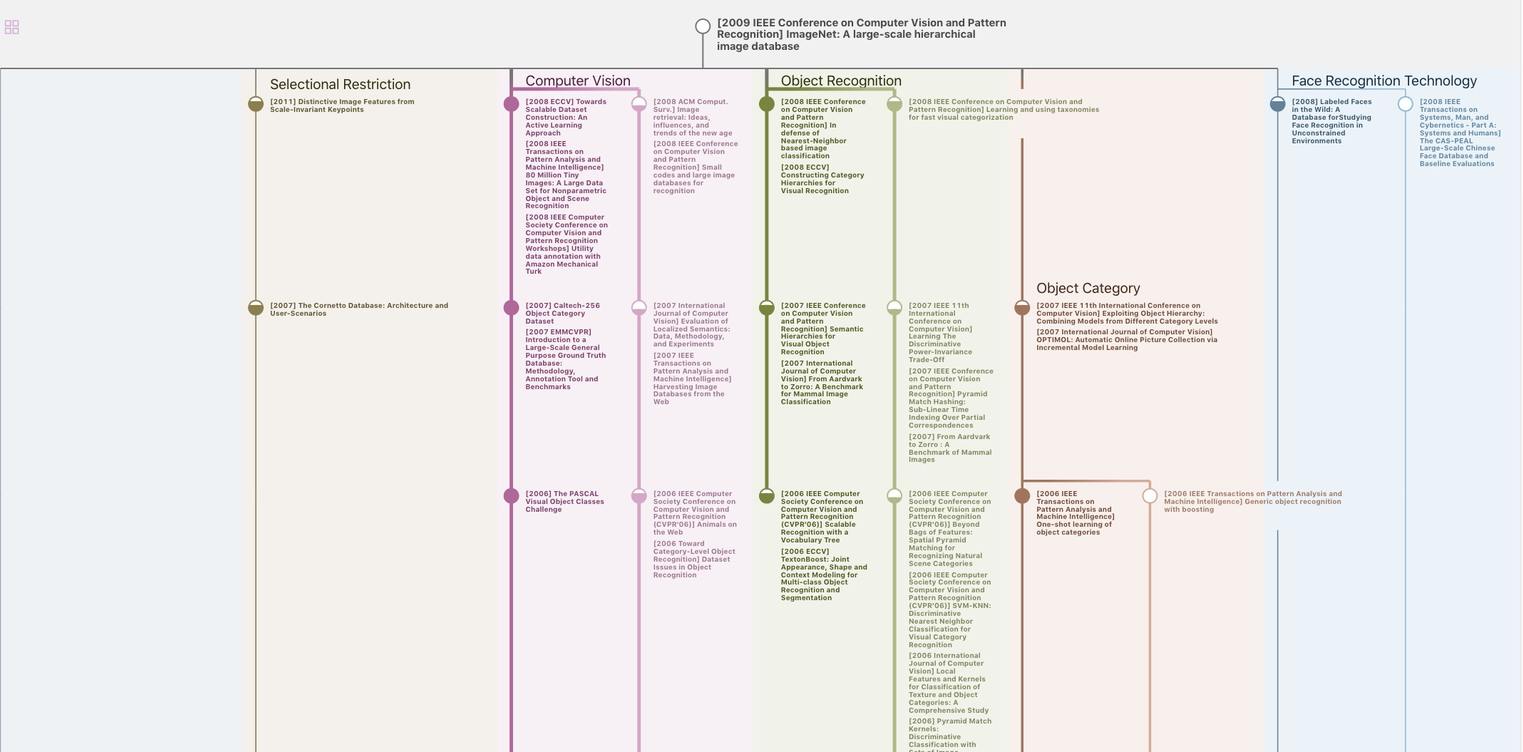
生成溯源树,研究论文发展脉络
Chat Paper
正在生成论文摘要