Preserving the value of large scale data analytics over time through selective re-computation
DATA ANALYTICS(2016)
摘要
A pervasive problem in Data Science is that the knowledge generated by possibly expensive analytics processes is subject to decay over time, as the data used to compute it drifts, the algorithms used in the processes are improved, and the external knowledge embodied by reference datasets used in the computation evolves. Deciding when such knowledge outcomes should be refreshed, following a sequence of data change events, requires problem-specific functions to quantify their value and its decay over time, as well as models for estimating the cost of their re-computation. What makes this problem challenging is the ambition to develop a decision support system for informing data analytics re-computation decisions over time, that is both generic and customisable. With the help of a case study from genomics, in this vision paper we offer an initial formalisation of this problem, highlight research challenges, and outline a possible approach based on the collection and analysis of metadata from a history of past computations.
更多查看译文
关键词
Selective re-computation, Incremental computation, Partial re-computation, Provenance, Metadata management
AI 理解论文
溯源树
样例
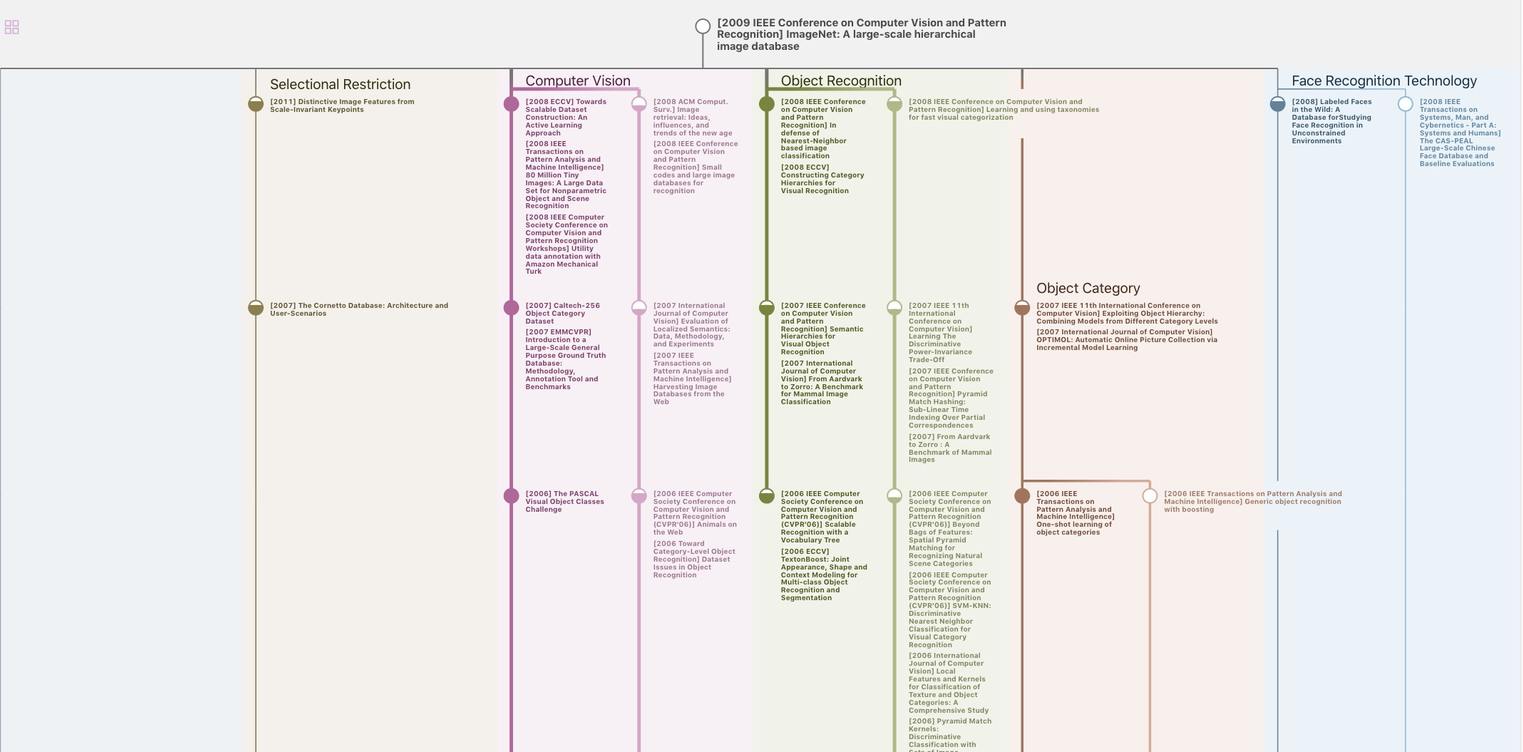
生成溯源树,研究论文发展脉络
Chat Paper
正在生成论文摘要