Improved bounds for sparse recovery from subsampled random convolutions.
ANNALS OF APPLIED PROBABILITY(2018)
摘要
We study the recovery of sparse vectors from subsampled random convolutions via l(1)-minimization. We consider the setup in which both the subsampling locations as well as the generating vector are chosen at random. For a sub-Gaussian generator with independent entries, we improve previously known estimates: if the sparsity s is small enough, that is, s less than or similar to root n/log(n), we show that m greater than or similar to s log(en/s) measurements are sufficient to recover s-sparse vectors in dimension n with high probability, matching the well-known condition for recovery from standard Gaussian measurements. If s is larger, then essentially m >= s log(2)(s) log(log(s)) log(n) measurements are sufficient, again improving over previous estimates. Our results are shown via the so-called robust null space property which is weaker than the standard restricted isometry property. Our method of proof involves a novel combination of small ball estimates with chaining techniques which should be of independent interest.
更多查看译文
关键词
Circulant matrix,compressive sensing,generic chaining,small ball estimates,sparsity
AI 理解论文
溯源树
样例
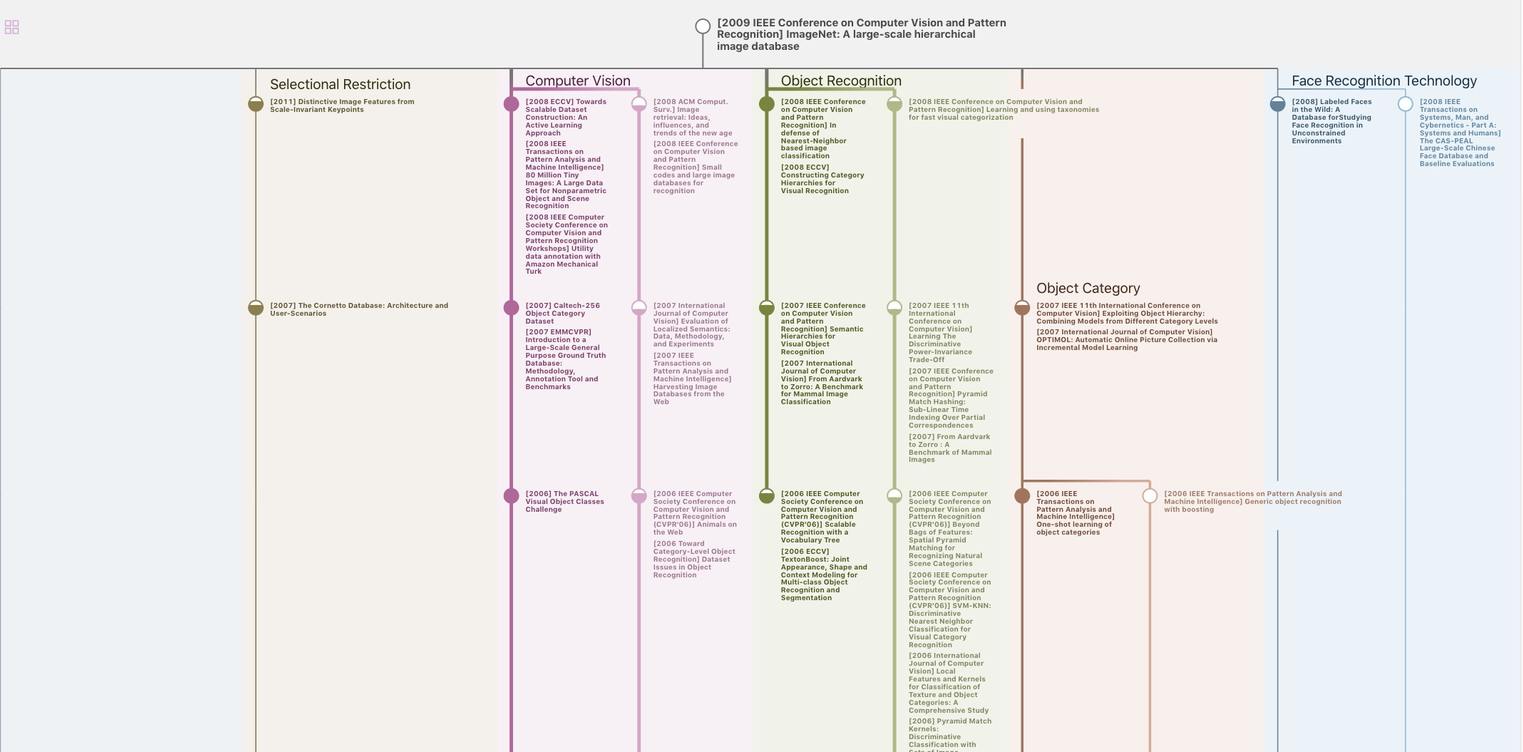
生成溯源树,研究论文发展脉络
Chat Paper
正在生成论文摘要