Quantifying Radiographic Knee Osteoarthritis Severity using Deep Convolutional Neural Networks
2016 23rd International Conference on Pattern Recognition (ICPR)(2016)
摘要
This paper proposes a new approach to automatically quantify the severity of knee osteoarthritis (OA) from radiographs using deep convolutional neural networks (CNN). Clinically, knee OA severity is assessed using Kellgren & Lawrence (KL) grades, a five point scale. Previous work on automatically predicting KL grades from radiograph images were based on training shallow classifiers using a variety of hand engineered features. We demonstrate that classification accuracy can be significantly improved using deep convolutional neural network models pre-trained on ImageNet and fine-tuned on knee OA images. Furthermore, we argue that it is more appropriate to assess the accuracy of automatic knee OA severity predictions using a continuous distance-based evaluation metric like mean squared error than it is to use classification accuracy. This leads to the formulation of the prediction of KL grades as a regression problem and further improves accuracy. Results on a dataset of X-ray images and KL grades from the Osteoarthritis Initiative (OAI) show a sizable improvement over the current state-of-the-art.
更多查看译文
关键词
Knee osteoarthritis,KL grades,Convolutional neural network,classification,regression,wndchrm
AI 理解论文
溯源树
样例
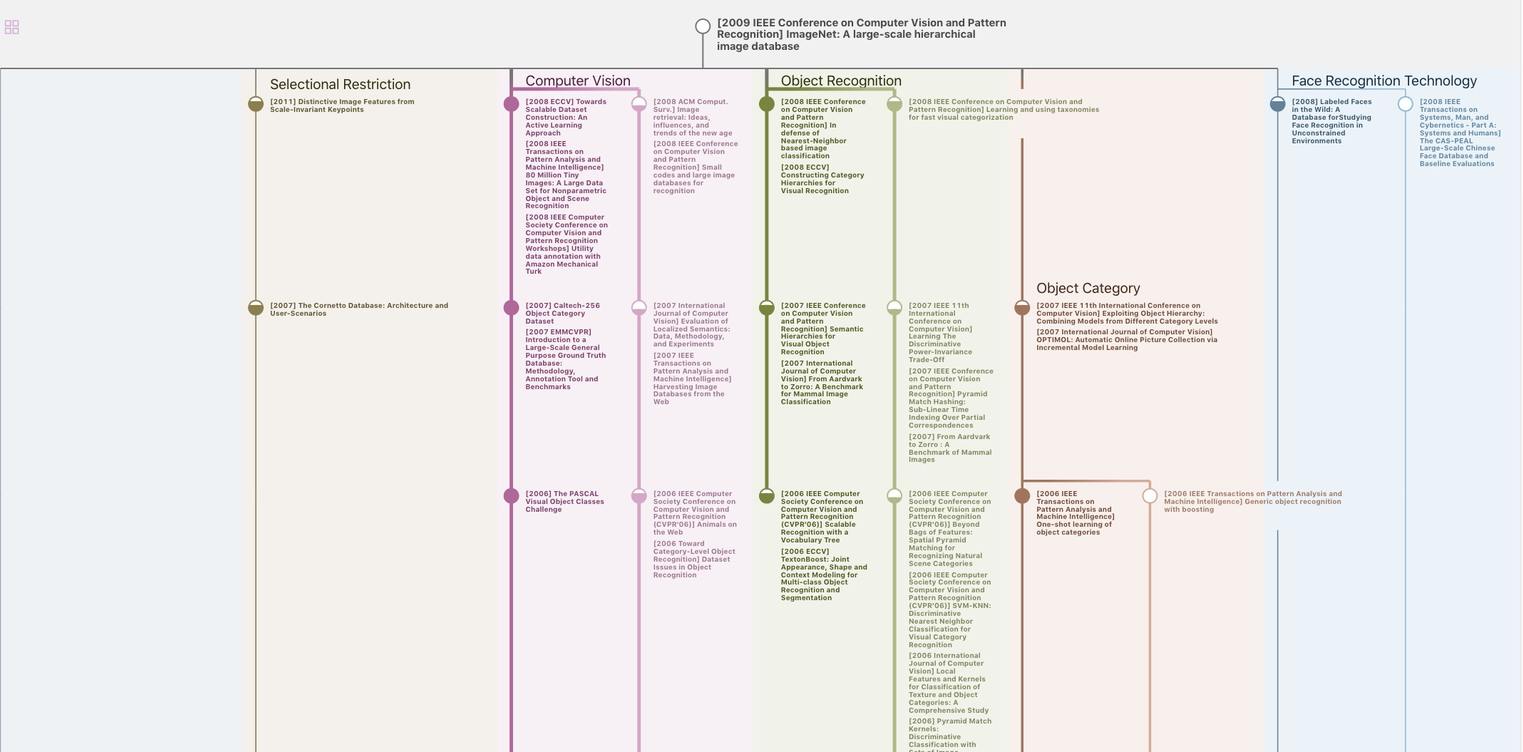
生成溯源树,研究论文发展脉络
Chat Paper
正在生成论文摘要