Online Data Thinning via Multi-Subspace Tracking.
IEEE transactions on pattern analysis and machine intelligence(2019)
摘要
In an era of ubiquitous large-scale streaming data, the availability of data far exceeds the capacity of expert human analysts. In many settings, such data is either discarded or stored unprocessed in data centers. This paper proposes a method of online data thinning, in which large-scale streaming datasets are winnowed to preserve unique, anomalous, or salient elements for timely expert analysis. At the heart of this proposed approach is an online anomaly detection method based on dynamic, low-rank Gaussian mixture models. Specifically, the high-dimensional covariances matrices associated with the Gaussian components are associated with low-rank models. According to this model, most observations lie near a union of subspaces. The low-rank modeling mitigates the curse of dimensionality associated with anomaly detection for high-dimensional data, and recent advances in subspace clustering and subspace tracking allow the proposed method to adapt to dynamic environments. Furthermore, the proposed method allows subsampling, is robust to missing data, and uses a mini-batch online optimization approach. The resulting algorithms are scalable, efficient, and are capable of operating in real time. Experiments on wide-area motion imagery and e-mail databases illustrate the efficacy of the proposed approach.
更多查看译文
关键词
Streaming media,Task analysis,Saliency detection,Sensors,Anomaly detection,Robustness,Clustering algorithms
AI 理解论文
溯源树
样例
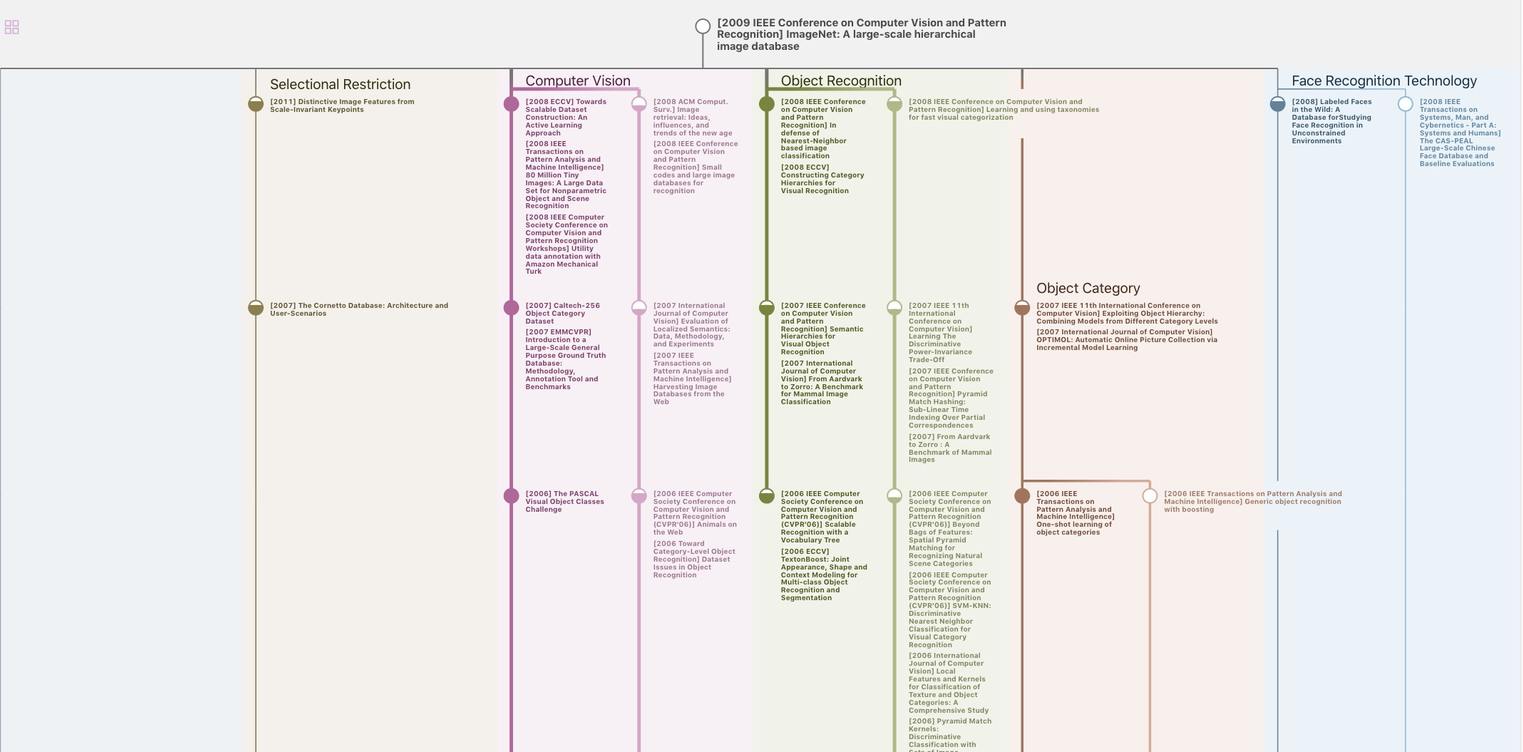
生成溯源树,研究论文发展脉络
Chat Paper
正在生成论文摘要