Solving Visual Madlibs with Multiple Cues.
BMVC(2016)
摘要
This paper presents an approach for answering fill-in-the-blank multiple choice questions from the Visual Madlibs dataset. Instead of generic and commonly used representations trained on the ImageNet dataset, our approach employs a combination of networks trained for specialized tasks such as scene recognition, person activity classification, and attribute prediction. We also present a method for localizing phrases from candidate answers in order to provide spatial support for feature extraction. We map each of these features, together with candidate answers, to a joint embedding space through normalized canonical correlation analysis (CCA). Finally, we solve an optimization problem to learn to combine CCA scores from multiple cues to select the best answer. Extensive experimental results show a significant improvement over the previous state of the art and confirm that answering questions from a wide range of types benefits from examining a variety of image cues and carefully choosing the spatial support of feature extraction.
更多查看译文
AI 理解论文
溯源树
样例
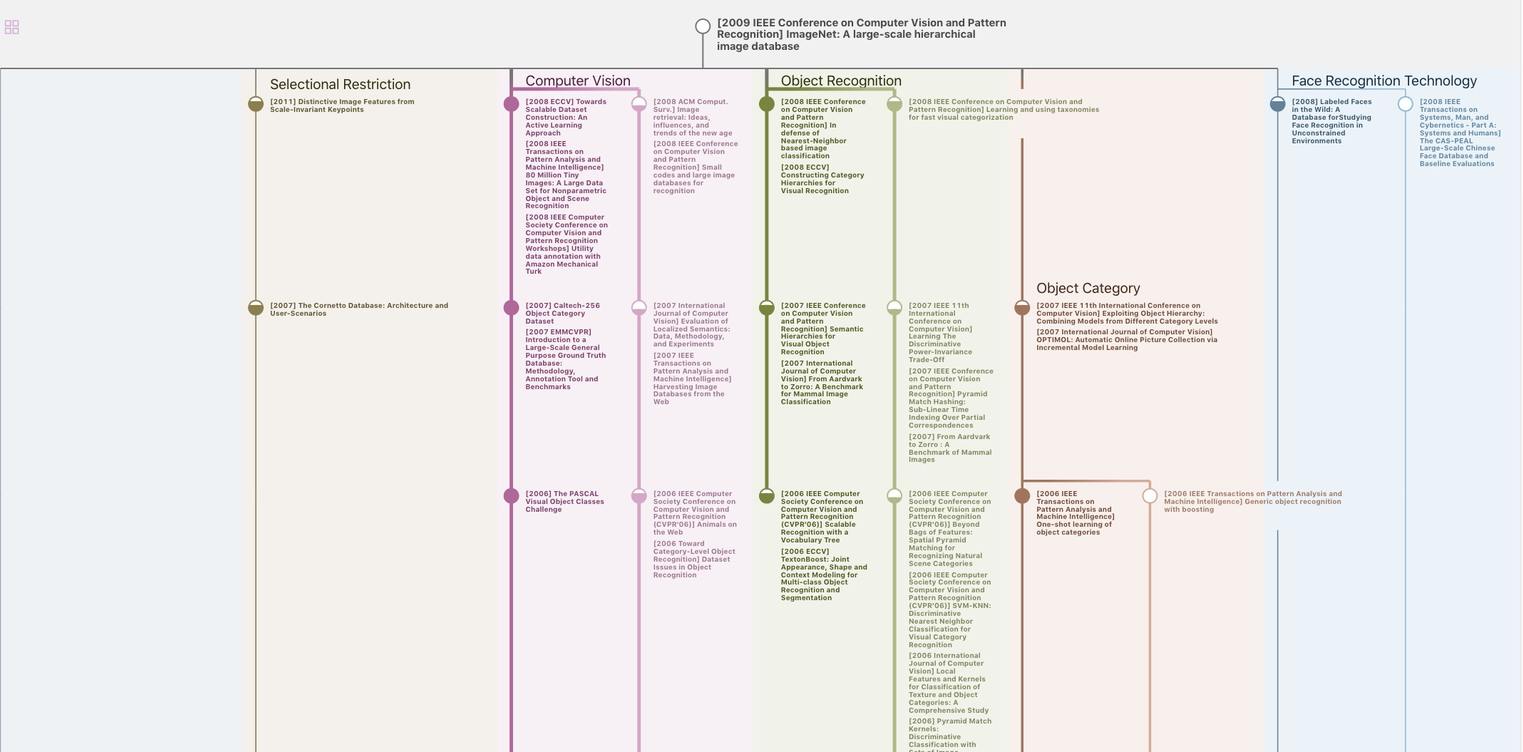
生成溯源树,研究论文发展脉络
Chat Paper
正在生成论文摘要