Deep Neural Networks for Improved, Impromptu Trajectory Tracking of Quadrotors
2017 IEEE International Conference on Robotics and Automation (ICRA)(2016)
摘要
Trajectory tracking control for quadrotors is important for applications ranging from surveying and inspection, to film making. However, designing and tuning classical controllers, such as proportional-integral-derivative (PID) controllers, to achieve high tracking precision can be time-consuming and difficult, due to hidden dynamics and other non-idealities. The Deep Neural Network (DNN), with its superior capability of approximating abstract, nonlinear functions, proposes a novel approach for enhancing trajectory tracking control. This paper presents a DNN-based algorithm as an add-on module that improves the tracking performance of a classical feedback controller. Given a desired trajectory, the DNNs provide a tailored reference input to the controller based on their gained experience. The input aims to achieve a unity map between the desired and the output trajectory. The motivation for this work is an interactive "fly-as-you-draw" application, in which a user draws a trajectory on a mobile device, and a quadrotor instantly flies that trajectory with the DNN-enhanced control system. Experimental results demonstrate that the proposed approach improves the tracking precision for user-drawn trajectories after the DNNs are trained on selected periodic trajectories, suggesting the method's potential in real-world applications. Tracking errors are reduced by around 40-50 highlighting the DNNs' capability of generalizing knowledge.
更多查看译文
关键词
deep neural networks,quadrotors,trajectory tracking control,proportional-integral-derivative controllers,PID,tracking precision,deep neural network,DNN-based algorithm,add-on module,feedback controller,unity map,interactive fly-as-you-draw application,DNN-enhanced control system,periodic trajectories
AI 理解论文
溯源树
样例
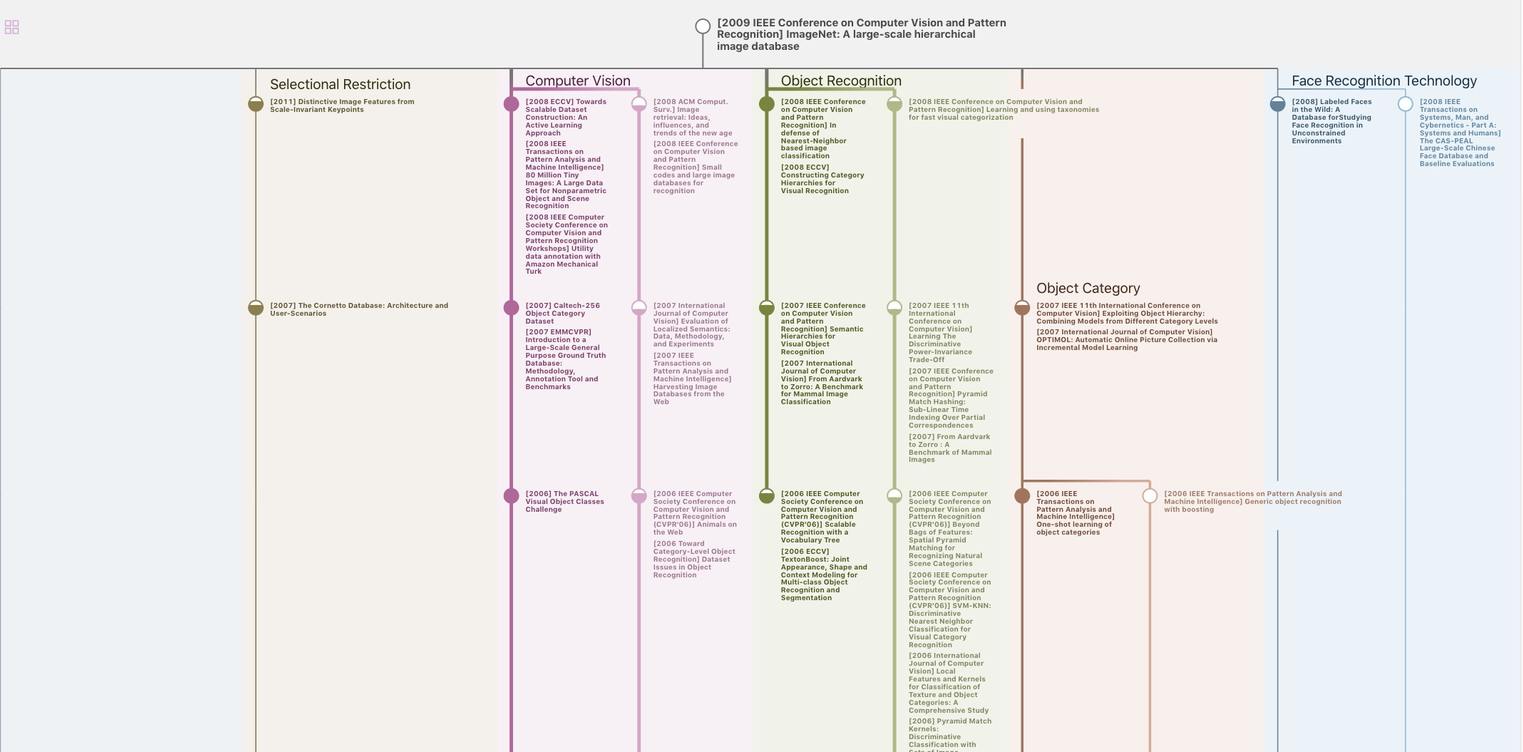
生成溯源树,研究论文发展脉络
Chat Paper
正在生成论文摘要