Generative Transfer Learning between Recurrent Neural Networks.
arXiv: Learning(2016)
摘要
Training a neural network demands a large amount of labeled data. Keeping the data after the training may not be allowed because of legal or privacy reasons. In this study, we train a new RNN, called a student network, using a previously developed RNN, the teacher network, without using the original data. The teacher network is used for generating a data for training the student network. In order to generate a long sequence of data that does not repeat, a random number assisted output label selection method is employed. The softmax output of the teacher RNN is used as for the soft target when training a student network. The performance evaluation is conducted using a character-level language model. The experimental results show that the proposed method yields good performance approaching that of the original data based training. This work not only gives insight to knowledge transfer between RNNs but also can be useful when the original training data is not available.
更多查看译文
AI 理解论文
溯源树
样例
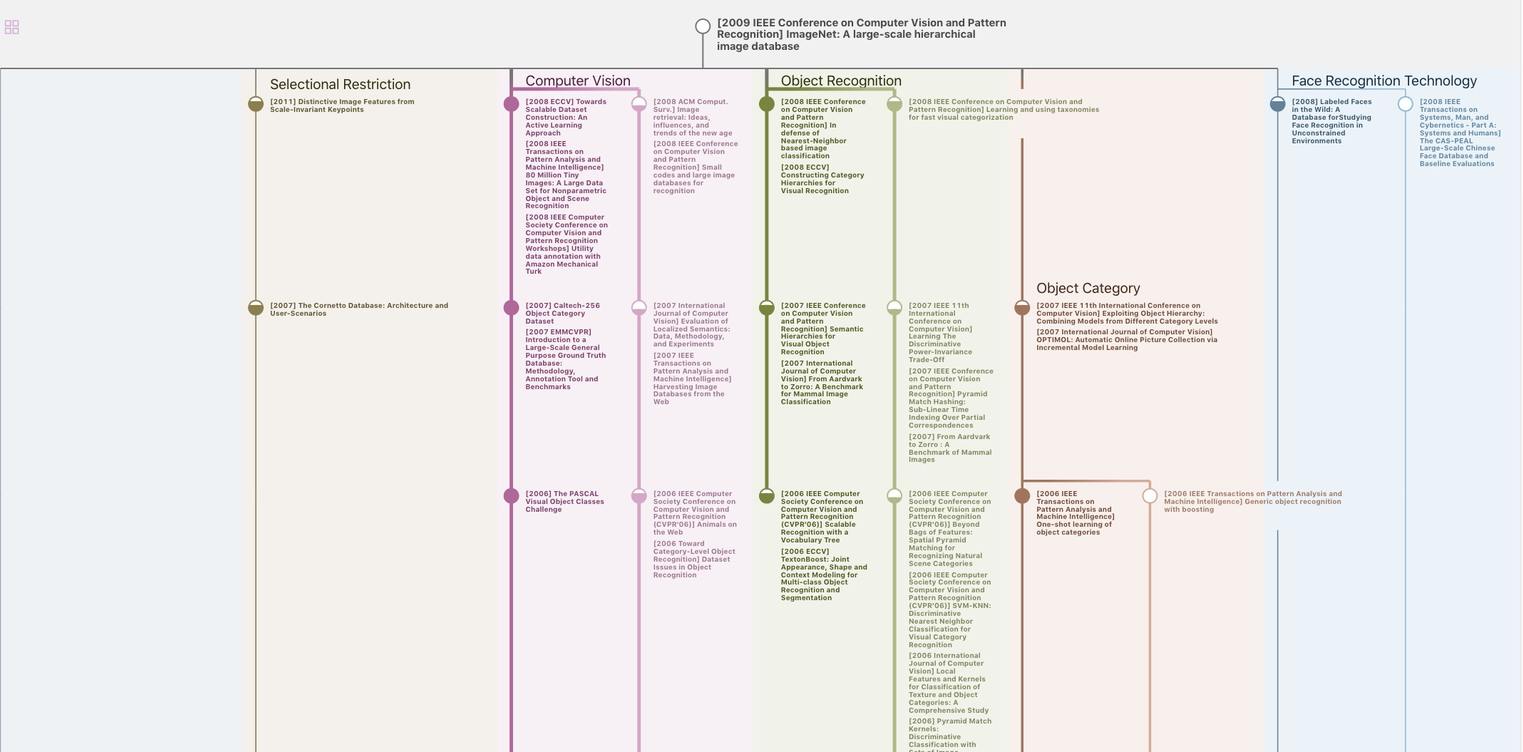
生成溯源树,研究论文发展脉络
Chat Paper
正在生成论文摘要