Detecting highly cyclic structure with complex eigenpairs
arXiv (Cornell University)(2016)
摘要
Many large, real-world complex networks have rich community structure that a network scientist seeks to understand. These communities may overlap or have intricate internal structure. Extracting communities with particular topological structure, even when they overlap with other communities, is a powerful capability that would provide novel avenues of focusing in on structure of interest. In this work we consider extracting highly-cyclic regions of directed graphs (digraphs). We demonstrate that embeddings derived from complex-valued eigenvectors associated with stochastic propagator eigenvalues near roots of unity are well-suited for this purpose. We prove several fundamental theoretic results demonstrating the connection between these eigenpairs and the presence of highly-cyclic structure and we demonstrate the use of these vectors on a few real-world examples.
更多查看译文
关键词
cyclic structure,complex eigenpairs
AI 理解论文
溯源树
样例
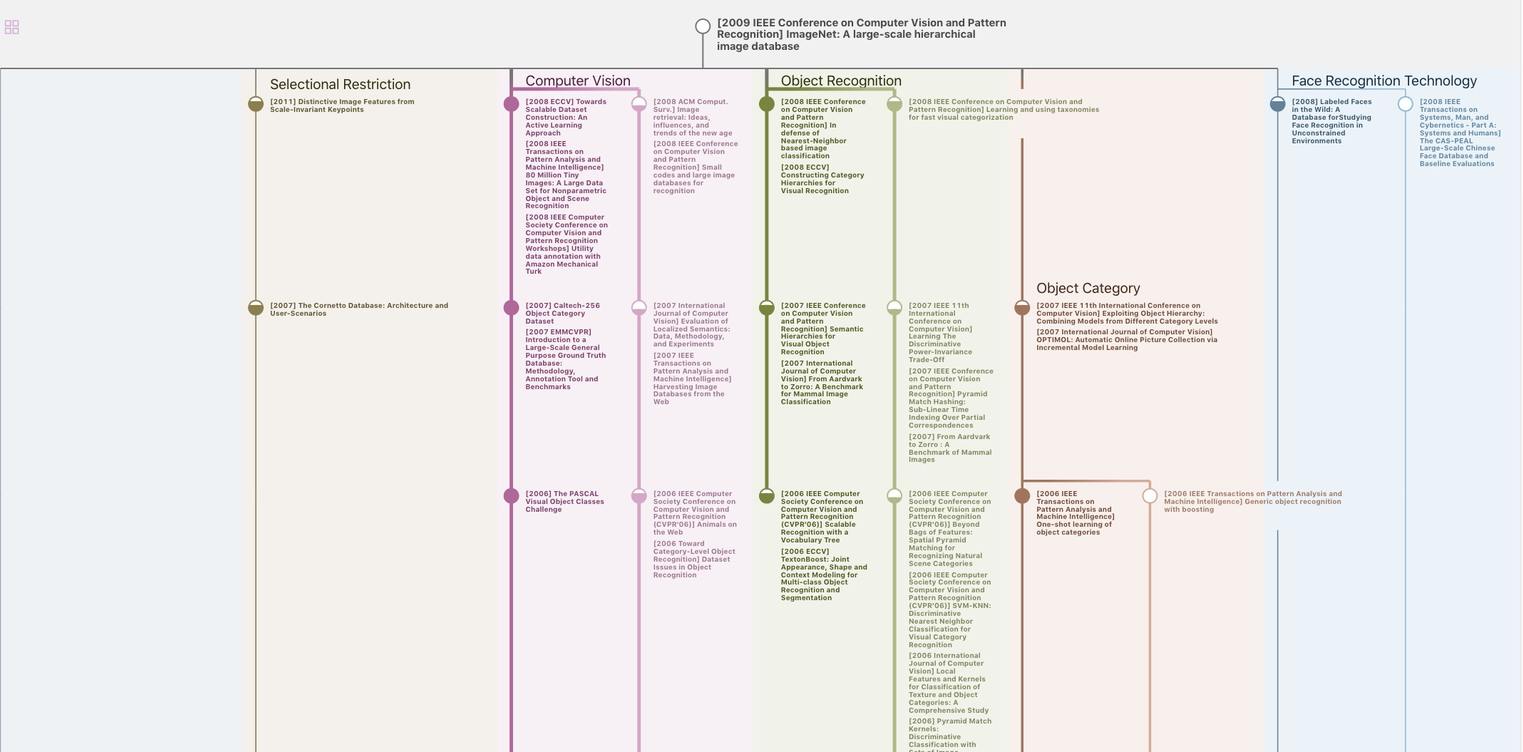
生成溯源树,研究论文发展脉络
Chat Paper
正在生成论文摘要