Estimating user behavior toward detecting anomalous ratings in rating systems.
Knowl.-Based Syst.(2016)
摘要
Online rating system plays a crucial role in collaborative filtering recommender systems (CFRSs). However, CFRSs are highly vulnerable to \"shilling\" attacks in reality. How to quickly and effectively spot and remove anomalous ratings before recommendation also is a big challenge. In this paper, we propose an unsupervised method to detect the attacks, which consists of three stages. Firstly, an undirected user-user graph is constructed from original user profiles. Based on the graph, a graph mining method is employed to estimate the similarity between vertices for creating a reduced graph. Then, similarity analysis is used to distinguish the difference between the vertices in order to rule out a part of genuine users. Finally, the remained genuine users are further filtered out by analyzing target items and the attackers can be detected. Extensive experiments on the MovieLens datasets demonstrate the effectiveness of the proposed method as compared to benchmark methods.
更多查看译文
关键词
Recommender system,Graph mining,Shilling attack,Abnormal detection
AI 理解论文
溯源树
样例
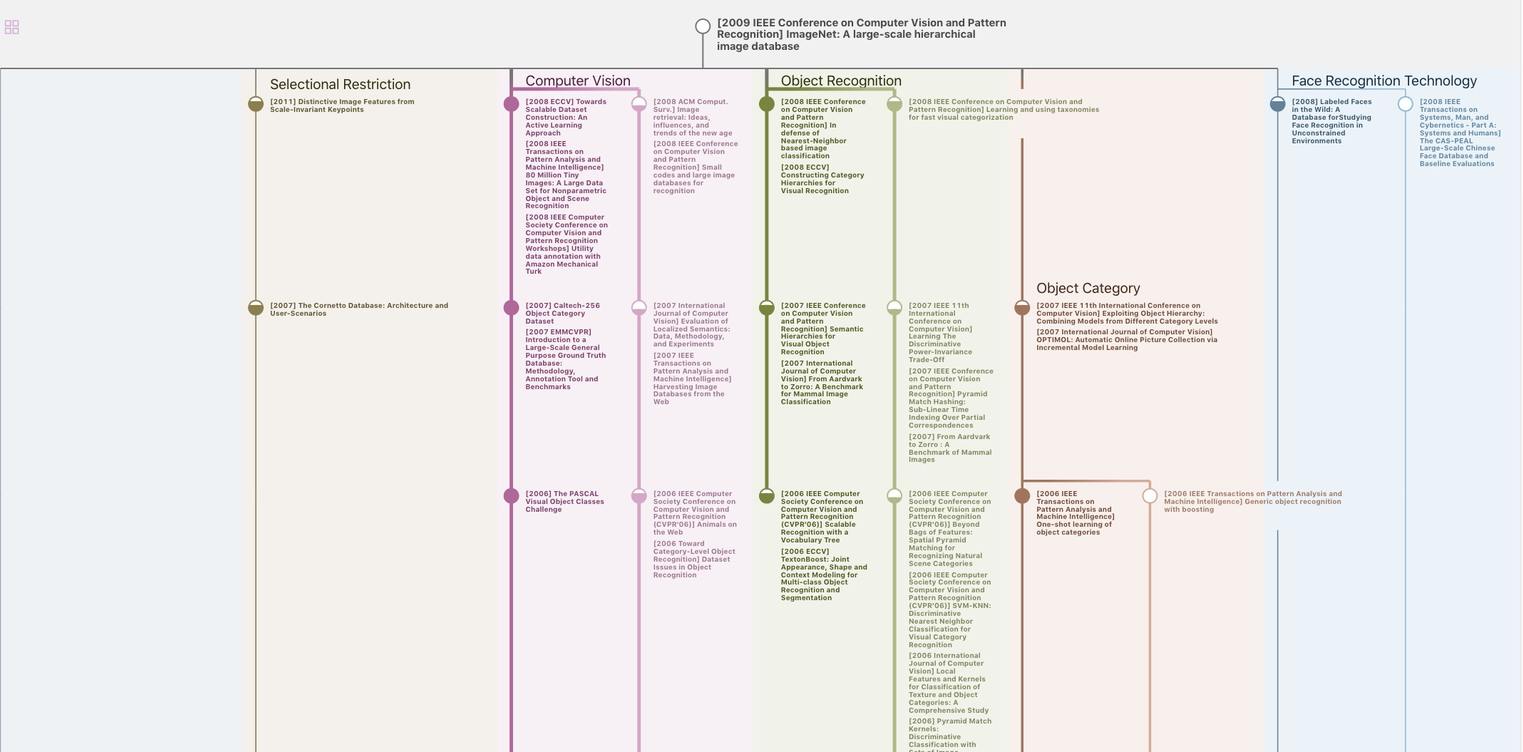
生成溯源树,研究论文发展脉络
Chat Paper
正在生成论文摘要