Weight Uncertainty in Boltzmann Machine
Cognitive Computation(2016)
摘要
Background Based on restricted Boltzmann machine (RBM), the deep learning models can be roughly divided into deep belief networks (DBNs) and deep Boltzmann machine (DBM). However, the overfitting problems commonly exist in neural networks and RBM models. In order to alleviate the overfitting problem, lots of research has been done. This paper alleviated the overfitting problem in RBM and proposed the weight uncertainty semi-restricted Boltzmann machine (WSRBM) to improve the ability of image recognition and image reconstruction. Methods First, this paper built weight uncertainty RBM model based on maximum likelihood estimation. And in the experimental section, this paper verified the effectiveness of the weight uncertainty deep belief network and the weight uncertainty deep Boltzmann machine. Second, in order to obtain better reconstructed images, this paper used the semi-restricted Boltzmann machine (SRBM) as the feature extractor and built the WSRBM. Lastly, this paper used hybrid Monte Carlo sampling and cRBM to improve the classification ability of WSDBM. Results The experiments showed that the weight uncertainty RBM, weight uncertainty DBN and weight uncertainty DBM were effective compared with the dropout method. And the WSDBM model performed well in image recognition and image reconstruction as well. Conclusions This paper introduced the weight uncertainty method to RBM, and proposed a WSDBM model, which was effective in image recognition and image reconstruction.
更多查看译文
关键词
RBM,DBM,DBN,Weight uncertainty
AI 理解论文
溯源树
样例
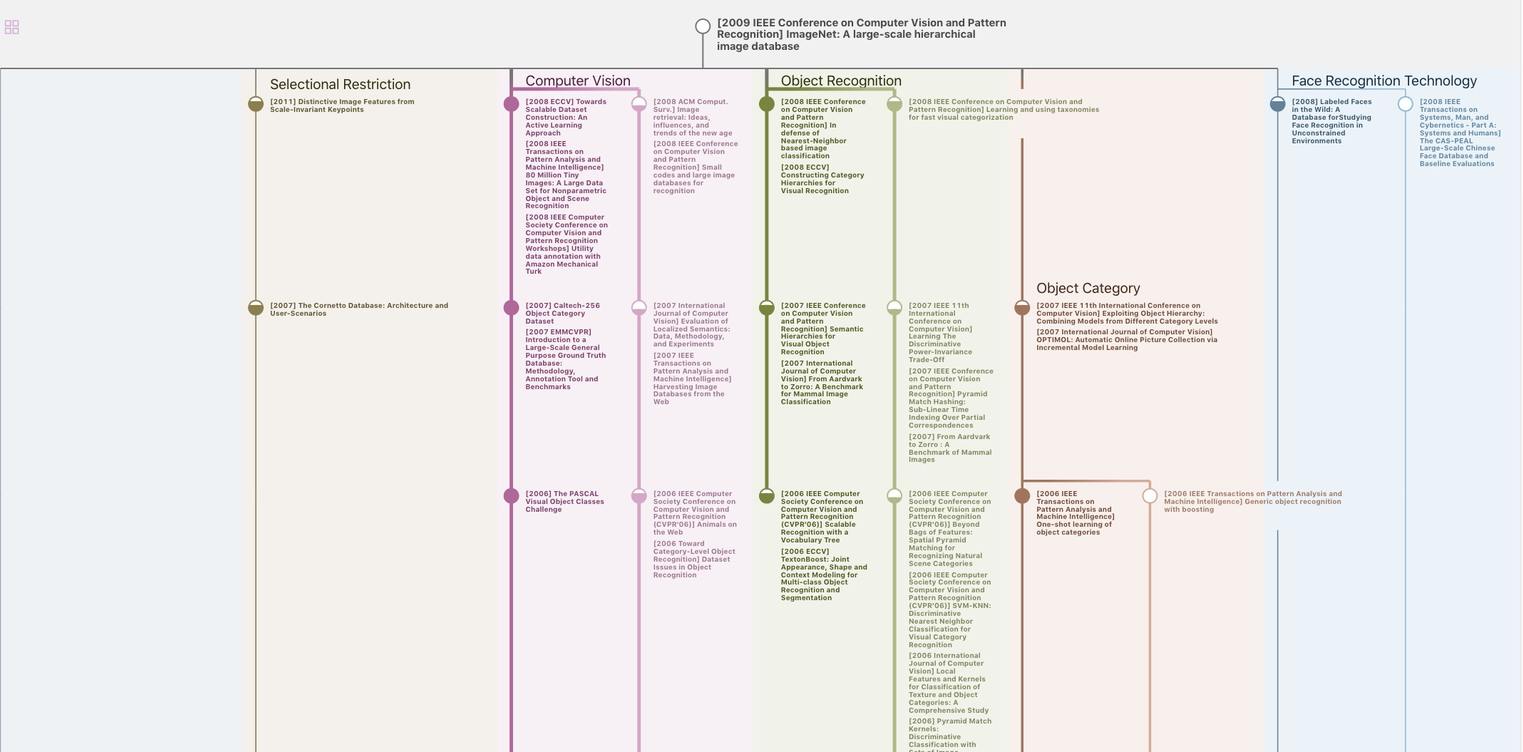
生成溯源树,研究论文发展脉络
Chat Paper
正在生成论文摘要