Nonparametric models for characterizing the topical communities in social network.
Neurocomputing(2016)
摘要
Topical communities have shown useful tools for characterizing social networks. However data in social networks often come as streams, i.e., both text content (e.g., emails, user postings) and network structure (e.g., user friendship) evolve over time. We propose two nonparametric statistic models where infinite latent community variables coupled with infinite latent topic variables. The temporal dependencies between variables across epochs are modeled via a rich-gets-richer scheme. We focus on characterizing three dynamic aspects in social streams: the number of communities or topics changes (e.g., new communities or topics are born and old ones die out); the popularity of communities or topics evolves; the semantics such as community topic distribution, community participant distribution and topic word distribution drift. Furthermore, we develop an effective online posterior inference algorithm for the models, which is concordant with the online nature of social streams. Experiments using real-world data show the effectiveness of our model at discovering the dynamic topical communities in social streams.
更多查看译文
关键词
Topical community,Social streams,Bayesian nonparametric models
AI 理解论文
溯源树
样例
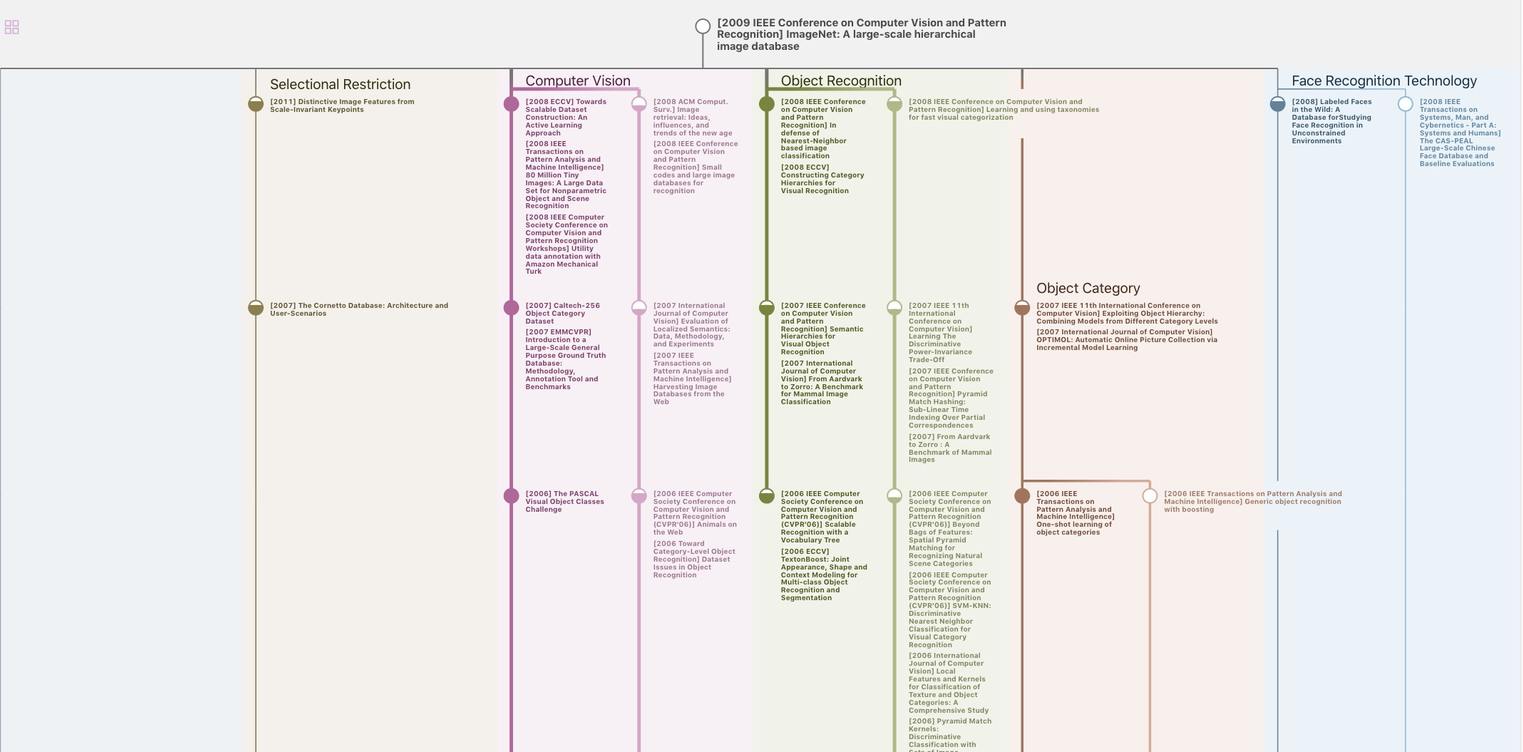
生成溯源树,研究论文发展脉络
Chat Paper
正在生成论文摘要