A Bayesian Hashing approach and its application to face recognition.
Neurocomputing(2016)
摘要
With the rapid development in the computer vision community, many recent studies show that high-dimensional feature representations can produce better accuracies in various image and video content recognition tasks. However, it also brings high costs for both computation and storage. In this paper, we introduce a novel method called Bayesian Hashing, which learns an optimal Hamming embedding to encode high-dimensional features to binary bits, and discuss its application to the challenging problem of face recognition. The learned hashing representation is modeled with a well-designed supervised Bayesian learning framework, which consists of three ingredients. First, we elaborately model local bit correlations using Naive Bayesian model (FERN), and boost FERNs to obtain a classifier for the hashing bit stream. Second, without incurring additional storage cost, we impose hashing bit-stream permutations to obtain a series of classifiers, which could achieve better performance. Third, we introduce the sequential forward floating search (SFFS) algorithm to perform model selection on multiple-permutation models, gaining further performance improvement. We carry out extensive evaluations and comparative studies, which demonstrate that the proposed approach gives superior performance on both accuracy and speed. State-of-the-art results are achieved on several well-known face recognition benchmarks.
更多查看译文
关键词
Bayesian Hashing,Hamming embedding,Face recognition
AI 理解论文
溯源树
样例
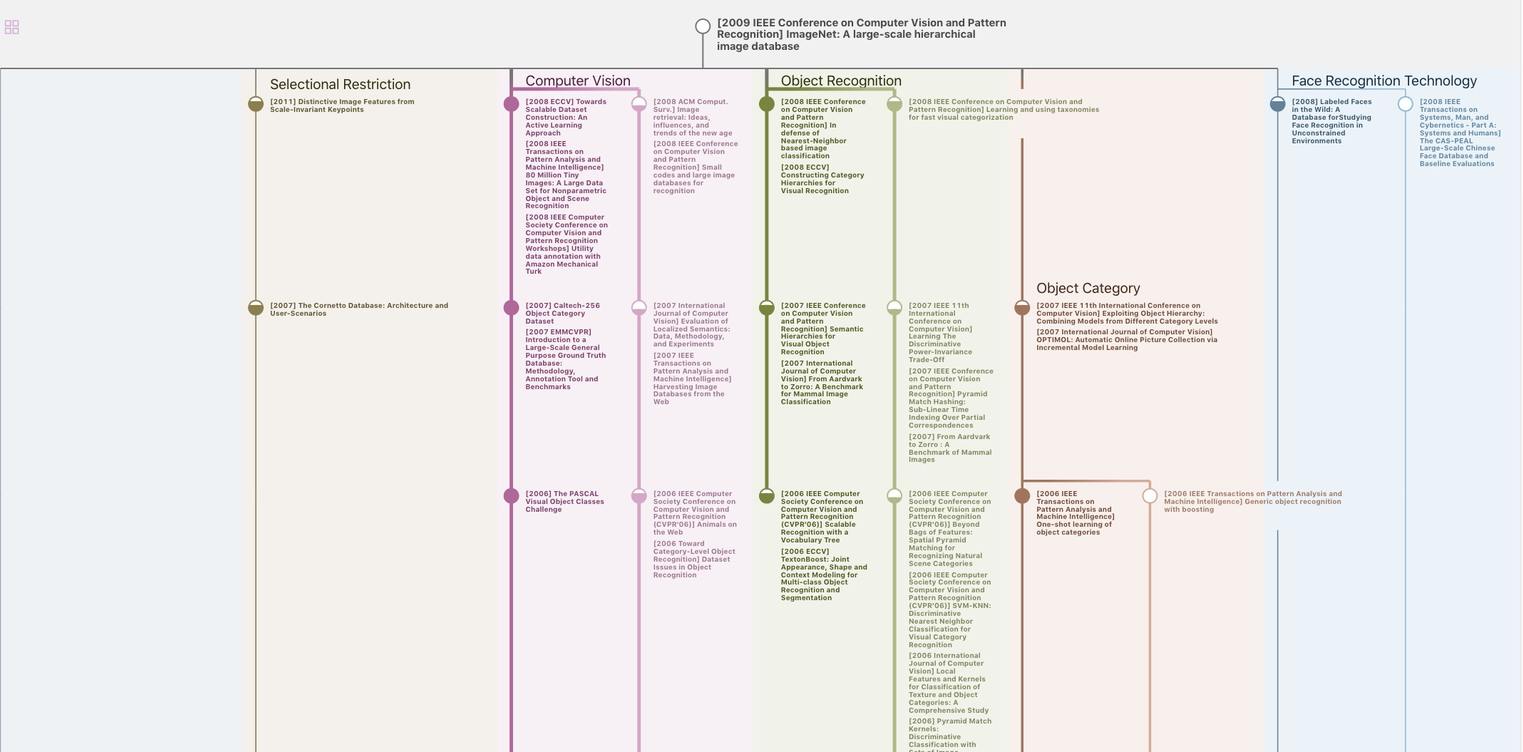
生成溯源树,研究论文发展脉络
Chat Paper
正在生成论文摘要