Utilizing cumulative population distribution information in differential evolution.
Appl. Soft Comput.(2016)
摘要
Display OmittedThe mutation, crossover, and selection of CPI-DE. Due to the fact that single population fails to contain enough information to estimate the covariance matrix reliably, this paper updates the covariance matrix in DE by an adaptation procedure, which makes use of the cumulative distribution information of the population.CPI-DE provides a simple yet efficient synergy of two kinds of crossover: the crossover in the Eigen coordinate system and the crossover in the original coordinate system. The former aims at identifying the properties of the fitness landscape and improving the efficiency and effectiveness of DE by producing the offspring toward promising directions. In addition, the purpose of the latter is to maintain the superiority of the original DE. Moreover, no extra parameters are required in CPI-DE.Our experimental studies have shown that CPI-DE is capable of enhancing the performance of several classic DE versions and advanced DE variants. Differential evolution (DE) is one of the most popular paradigms of evolutionary algorithms. In general, DE does not exploit distribution information provided by the population and, as a result, its search performance is limited. In this paper, cumulative population distribution information of DE has been utilized to establish an Eigen coordinate system by making use of covariance matrix adaptation. The crossover operator of DE implemented in the Eigen coordinate system has the capability to identify the features of the fitness landscape. Furthermore, we propose a cumulative population distribution information based DE framework called CPI-DE. In CPI-DE, for each target vector, two trial vectors are generated based on both the original coordinate system and the Eigen coordinate system. Then, the target vector is compared with these two trial vectors and the best one will survive into the next generation. CPI-DE has been applied to two classic versions of DE and three state-of-the-art variants of DE for solving two sets of benchmark test functions, namely, 28 test functions with 30 and 50 dimensions at the 2013 IEEE Congress on Evolutionary Computation, and 30 test functions with 30 and 50 dimensions at the 2014 IEEE Congress on Evolutionary Computation. The experimental results suggest that CPI-DE is an effective framework to enhance the performance of DE.
更多查看译文
关键词
Cumulative population distribution information,Differential evolution,Eigen coordinate system,Evolutionary algorithms
AI 理解论文
溯源树
样例
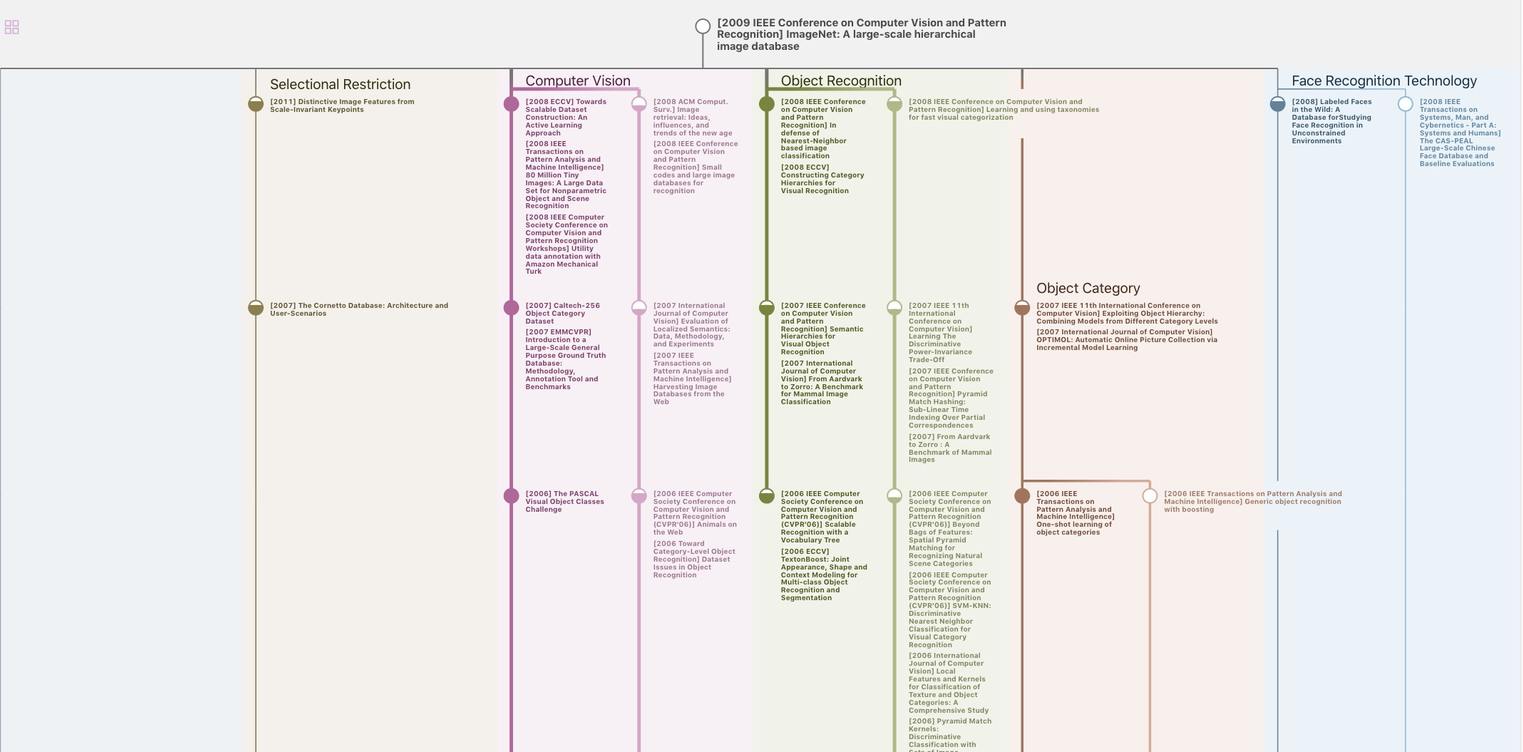
生成溯源树,研究论文发展脉络
Chat Paper
正在生成论文摘要