An Incremental Local-First Community Detection Method For Dynamic Graphs
2016 IEEE INTERNATIONAL CONFERENCE ON BIG DATA (BIG DATA)(2018)
摘要
Community detections for large-scale real world networks have been more popular in social analytics. In particular, dynamically growing network analyses become important to find long-term trends and detect anomalies. In order to analyze such networks, we need to obtain many snapshots and apply same analytic methods to them. However, it is inefficient to extract communities from these whole newly generated networks with little differences every time, and then it is impossible to follow the network growths in the real time. We proposed an incremental community detection algorithm for high-volume graph streams. It is based on the top of a well-known batch-oriented algorithm named DEMON [1]. We also evaluated performance and precisions of our proposed incremental algorithm with real-world big networks with up to 410,236 vertices and 2,439,437 edges and computed in less than one second to detect communities in an incremental fashion-which achieves up to 107 times faster than the original algorithm without sacrificing accuracies.
更多查看译文
关键词
Network theory (graphs), High performance computing
AI 理解论文
溯源树
样例
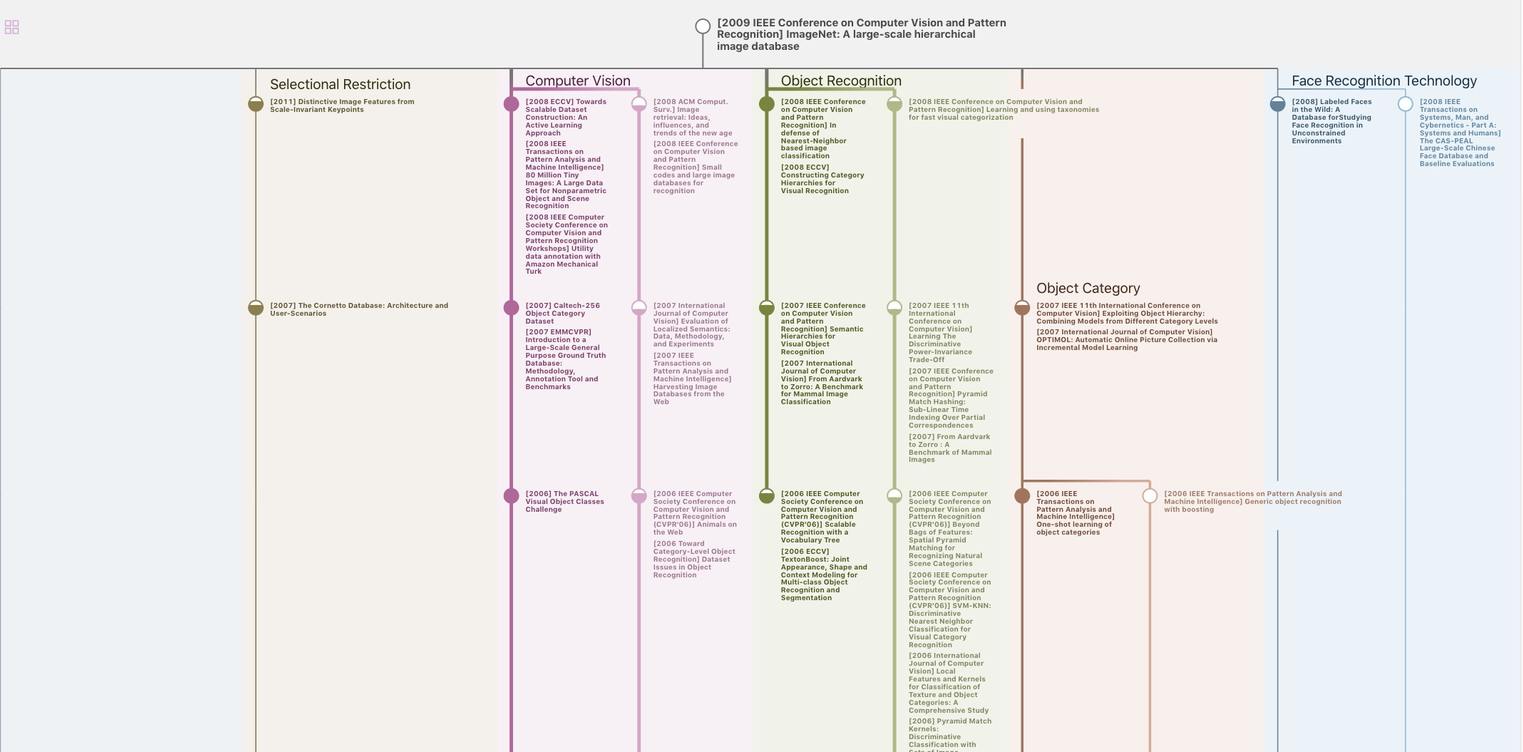
生成溯源树,研究论文发展脉络
Chat Paper
正在生成论文摘要