Content-Based Recommendation For Podcast Audio-Items Using Natural Language Processing Techniques
2016 IEEE INTERNATIONAL CONFERENCE ON BIG DATA (BIG DATA)(2016)
摘要
A podcast combines the liveliness of a FM radio channel with the economy of internet blog posting. They are especially convenient for scenarios when there is limited internet ability awl connectivity for example the car, the gym, etc. While both the volume and heterogeneity of content is huge it becomes operationally difficult to manually categorize or tag these audio items, thus manage them in a system for users to discover. Furthermore, due to the incompleteness of audio associated meta data there are not enough features for a typical recommender system to learn the item similarities thus make recommendations. In this paper We propose and examine a novel approach to generate latent embeddings for podcast items utilizing the aggregated information from all the text-based features associated with the audio items. These embeddings that are generated using well established Natural Language Processing (NLP) techniques for the podcast items can be used to measure or indicate the content similarity among the various podcast items. Both GPU (CUDA) and CPU computing architectures are experimented and bench marked for the model training, cross-validation of the content predictions on large scale datasets.
更多查看译文
关键词
Podcast item,Podcast,Various podcast item
AI 理解论文
溯源树
样例
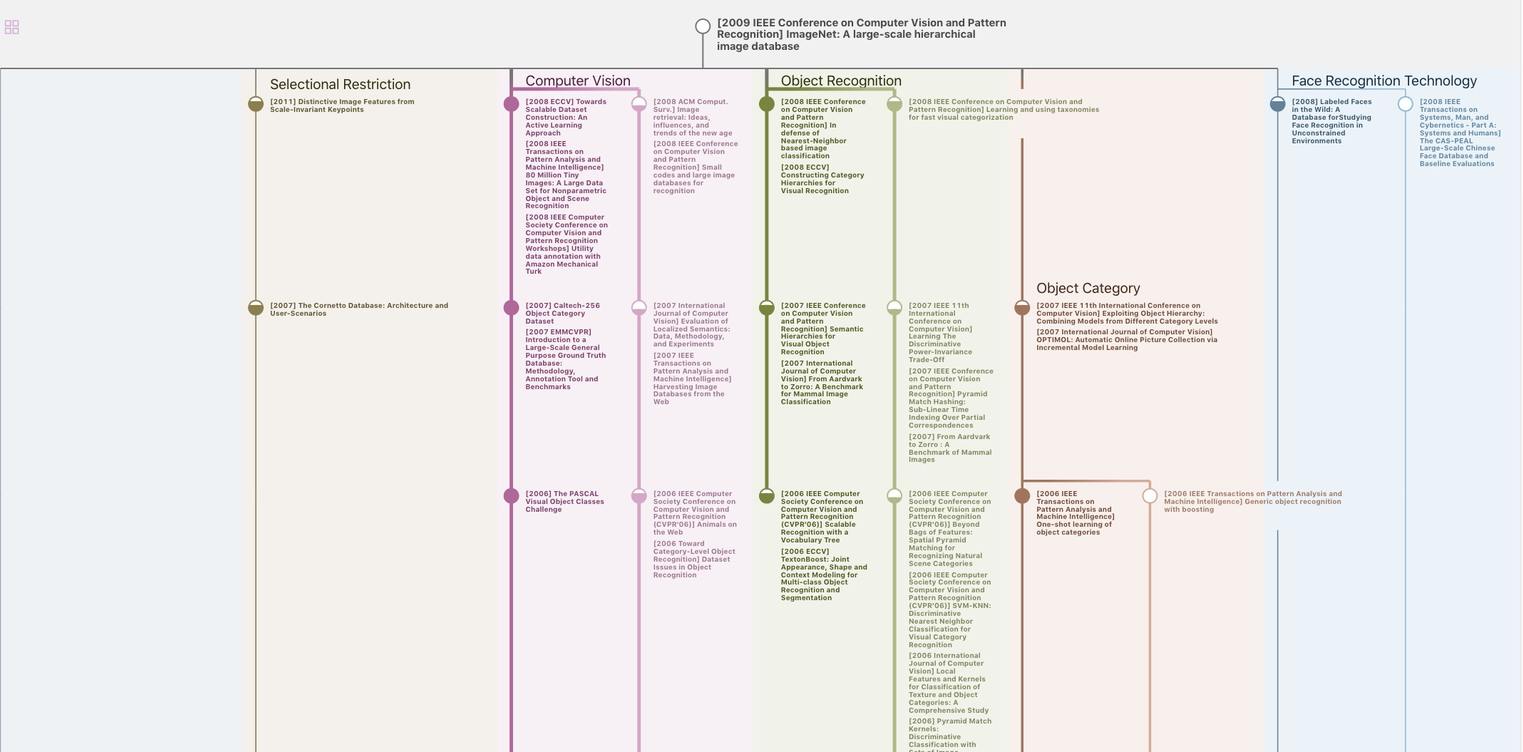
生成溯源树,研究论文发展脉络
Chat Paper
正在生成论文摘要