Online Multi-view Clustering with Incomplete Views
2016 IEEE INTERNATIONAL CONFERENCE ON BIG DATA (BIG DATA)(2016)
摘要
In the era of big data, it is common to have data with multiple modalities or coming from multiple sources, known as "multi-view data". Multi-view clustering provides a natural way to generate clusters from such data. Since different views share some consistency and complementary information, previous works on multi-view clustering mainly focus on how to combine various numbers of views to improve clustering performance. However, in reality, each view may be incomplete, i.e., instances missing in the view. Furthermore, the size of data could be extremely huge. It is unrealistic to apply multi-view clustering in large real-world applications without considering the incompleteness of views and the memory requirement. None of previous works have addressed all these challenges simultaneously. In this paper, we propose an online multi-view clustering algorithm, OMVC, which deals with large-scale incomplete views. We model the multi-view clustering problem as a joint weighted nonnegative matrix factorization problem and process the multi-view data chunk by chunk to reduce the memory requirement. OMVC learns the latent feature matrices for all the views and pushes them towards a consensus. We further increase the robustness of the learned latent feature matrices in OMVC via lasso regularization. To minimize the influence of incompleteness, dynamic weight setting is introduced to give lower weights to the incoming missing instances in different views. More importantly, to reduce the computational time, we incorporate a faster projected gradient descent by utilizing the Hessian matrices in OMVC. Extensive experiments conducted on four real data demonstrate the effectiveness of the proposed OMVC method.
更多查看译文
关键词
Multi-view clustering, Online algorithm, Incomplete views, Nonnegative matrix factorization
AI 理解论文
溯源树
样例
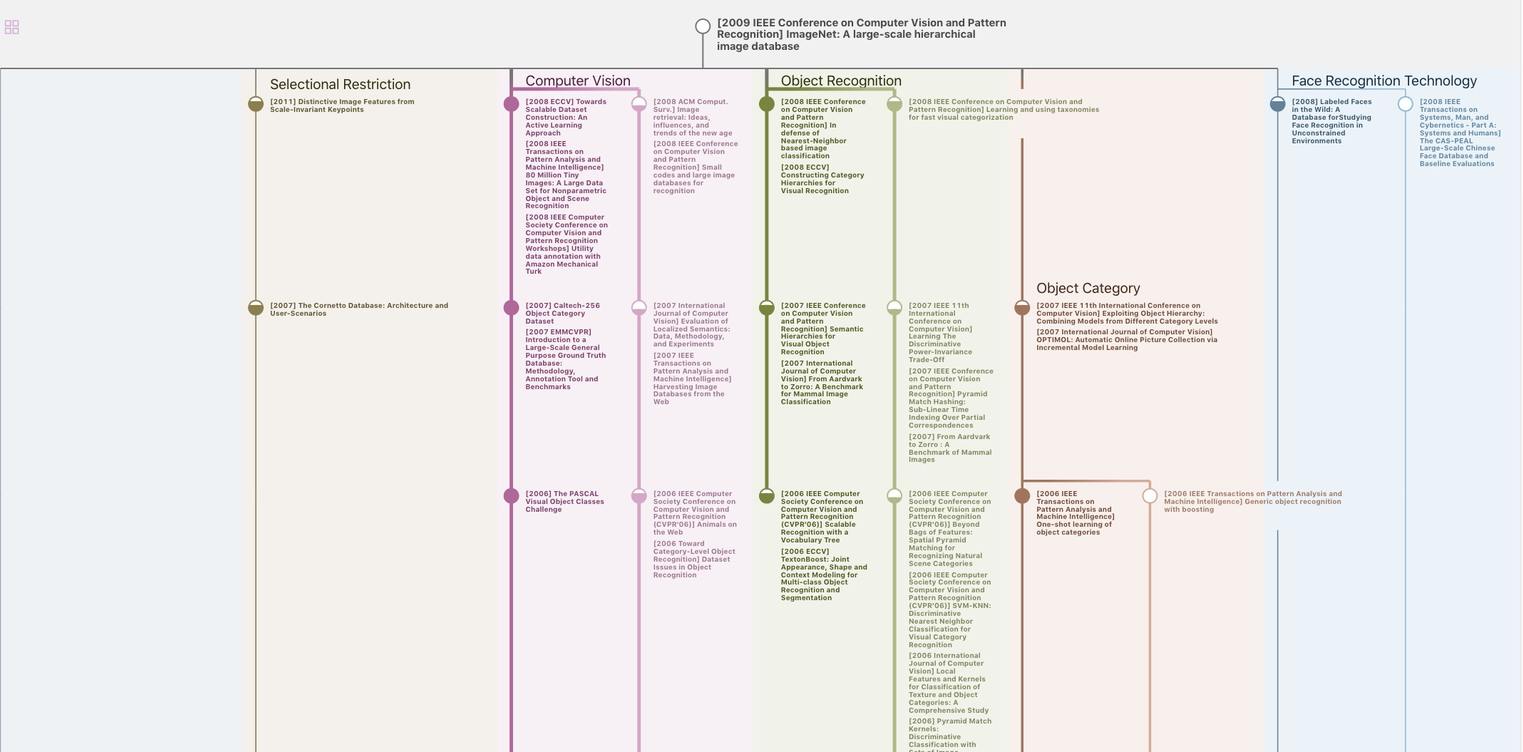
生成溯源树,研究论文发展脉络
Chat Paper
正在生成论文摘要