Empirical Evaluations of Preprocessing Parameters' Impact on Predictive Coding's Effectiveness
BigData(2016)
摘要
Predictive coding, once used in only a small fraction of legal and business matters, is now widely deployed to quickly cull through increasingly vast amounts of data and reduce the need for costly and inefficient human document review. Previously, the sole front-end input used to create a predictive model was the exemplar documents (training data) chosen by subjectmatter experts. Many predictive coding tools require users to rely on static preprocessing parameters and a single machine learning algorithm to develop the predictive model. Little research has been published discussing the impact preprocessing parameters and learning algorithms have on the effectiveness of the technology. A deeper dive into the generation of a predictive model shows that the settings and algorithm can have a strong effect on the accuracy and efficacy of a predictive coding tool. Understanding how these input parameters affect the output will empower legal teams with the information they need to implement predictive coding as efficiently and effectively as possible. This paper outlines different preprocessing parameters and algorithms as applied to multiple real-world data sets to understand the influence of various approaches.
更多查看译文
关键词
predictive coding,technology assisted review,electronic discovery,ediscovery,e-discovery
AI 理解论文
溯源树
样例
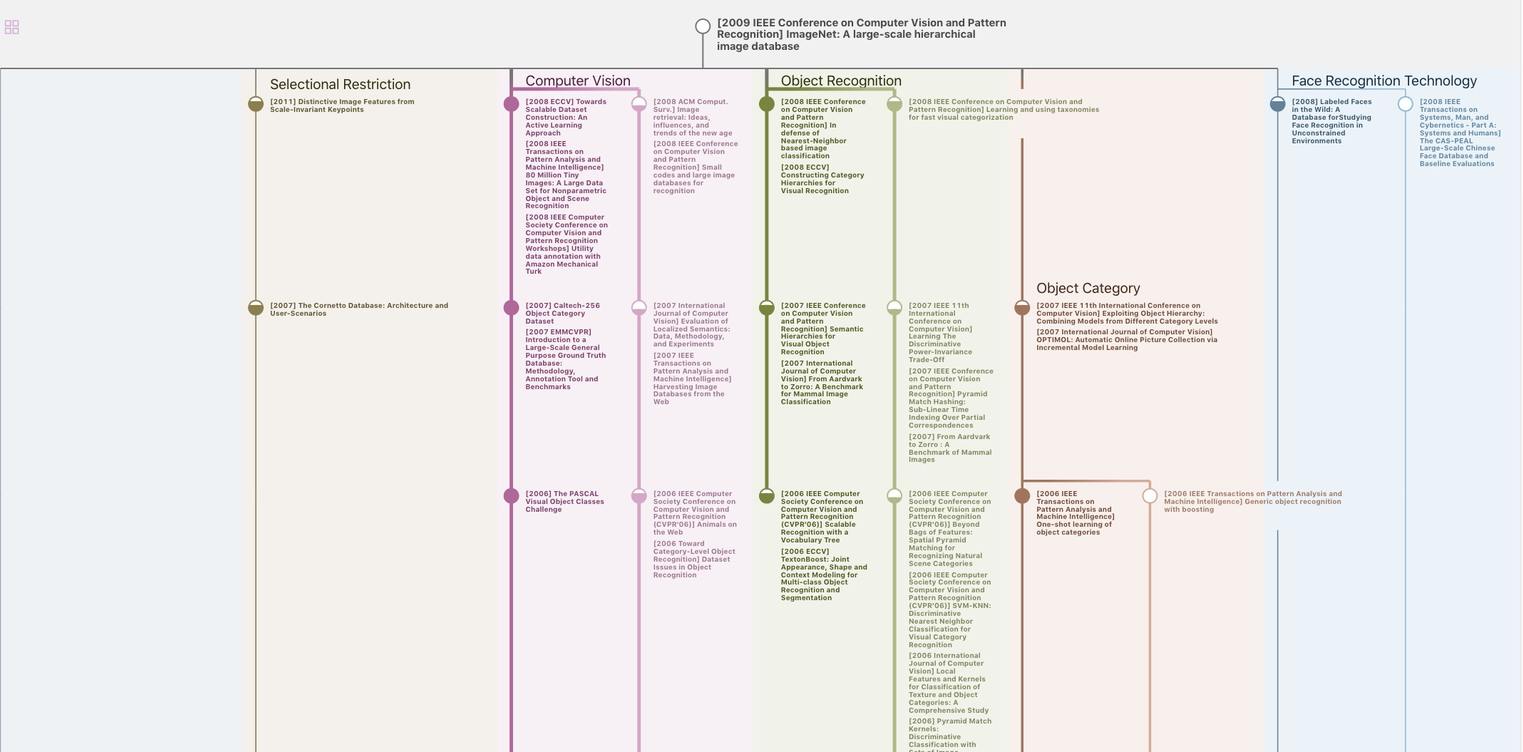
生成溯源树,研究论文发展脉络
Chat Paper
正在生成论文摘要