Factorizing Sequential And Historical Purchase Data For Basket Recommendation
INFORMATION RETRIEVAL TECHNOLOGY, AIRS 2016(2016)
摘要
Basket recommendation is an important task in market basket analysis. Existing work on this problem can be summarized into two paradigms. One is the item-centric paradigm, where sequential patterns are mined from users' transactional data and leveraged for prediction. However, these approaches usually suffer from the data sparseness problem. The other is the user-centric paradigm, where collaborative filtering techniques have been applied on users' historical data. However, these methods ignore the sequential behaviors of users, which are often crucial for basket recommendation. In this paper, we introduce a hybrid method, namely the Co-Factorization model over Sequential and Historical purchase data (CFSH for short) for basket recommendation. Compared with existing methods, our approach enjoys the following merits: (1) By mining and factorizing global sequential patterns, we can avoid the sparseness problem in traditional item-centric methods; (2) By factorizing item-item and user-item matrices simultaneously, we can exploit both sequential and historical behaviors to learn user and item representations better; (3) Experimental results on three real-world transaction datasets demonstrated the effectiveness of our approach as compared with the existing methods.
更多查看译文
关键词
Basket recommendation, Sequential patterns, Recommendation
AI 理解论文
溯源树
样例
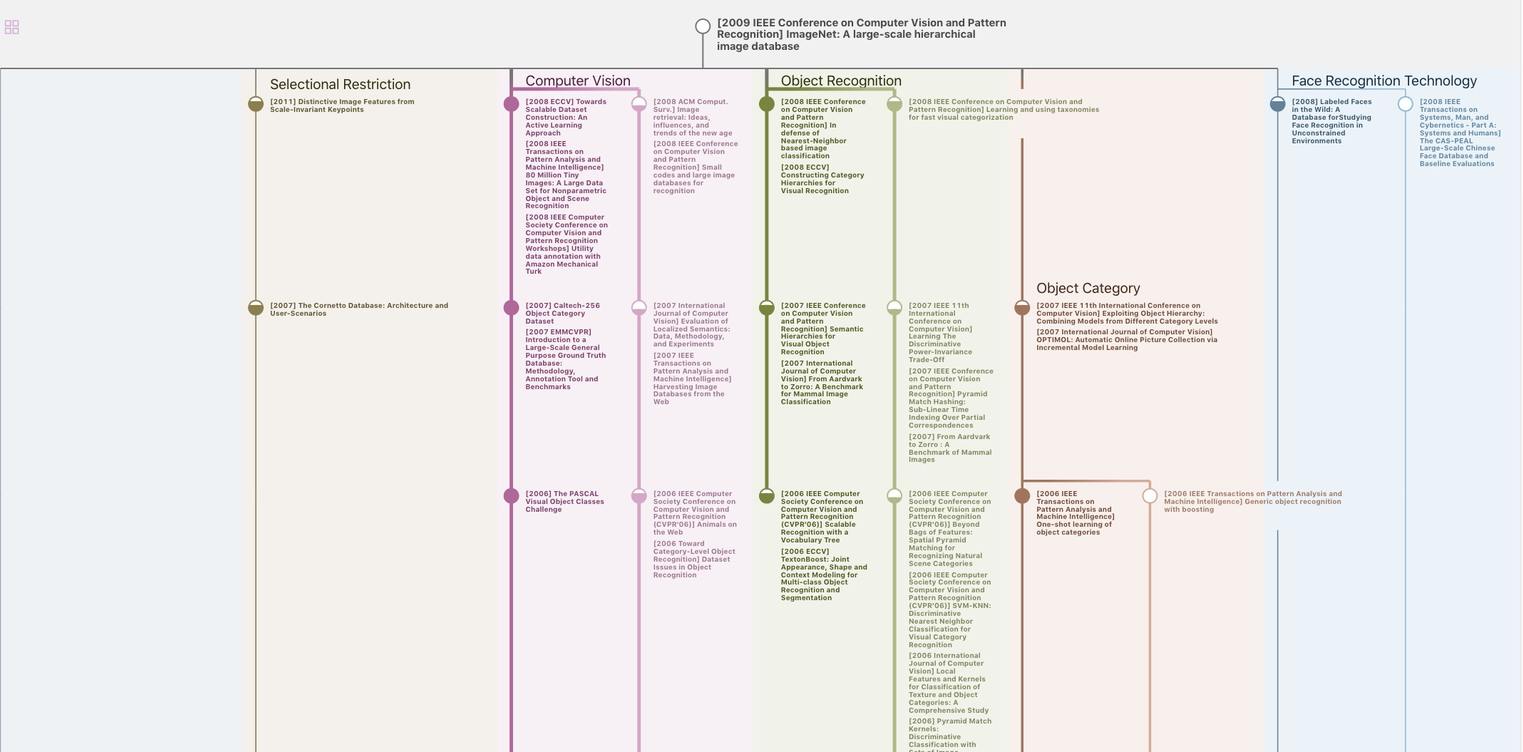
生成溯源树,研究论文发展脉络
Chat Paper
正在生成论文摘要