Network-based analysis of omics data: The LEAN method.
BIOINFORMATICS(2017)
摘要
Motivation: Most computational approaches for the analysis of omics data in the context of interaction networks have very long running times, provide single or partial, often heuristic, solutions and/or contain user-tuneable parameters. Results: We introduce local enrichment analysis (LEAN) for the identification of dysregulated sub-networks from genome-wide omics datasets. By substituting the common subnetwork model with a simpler local subnetwork model, LEAN allows exact, parameter-free, efficient and exhaustive identification of local subnetworks that are statistically dysregulated, and directly implicates single genes for follow-up experiments. Evaluation on simulated and biological data suggests that LEAN generally detects dysregulated subnetworks better, and reflects biological similarity between experiments more clearly than standard approaches. A strong signal for the local subnetwork around Von Willebrand Factor (VWF), a gene which showed no change on the mRNA level, was identified by LEAN in transcriptome data in the context of the genetic disease Cerebral Cavernous Malformations (CCM). This signal was experimentally found to correspond to an unexpected strong cellular effect on the VWF protein. LEAN can be used to pinpoint statistically significant local subnetworks in any genomescale dataset.
更多查看译文
AI 理解论文
溯源树
样例
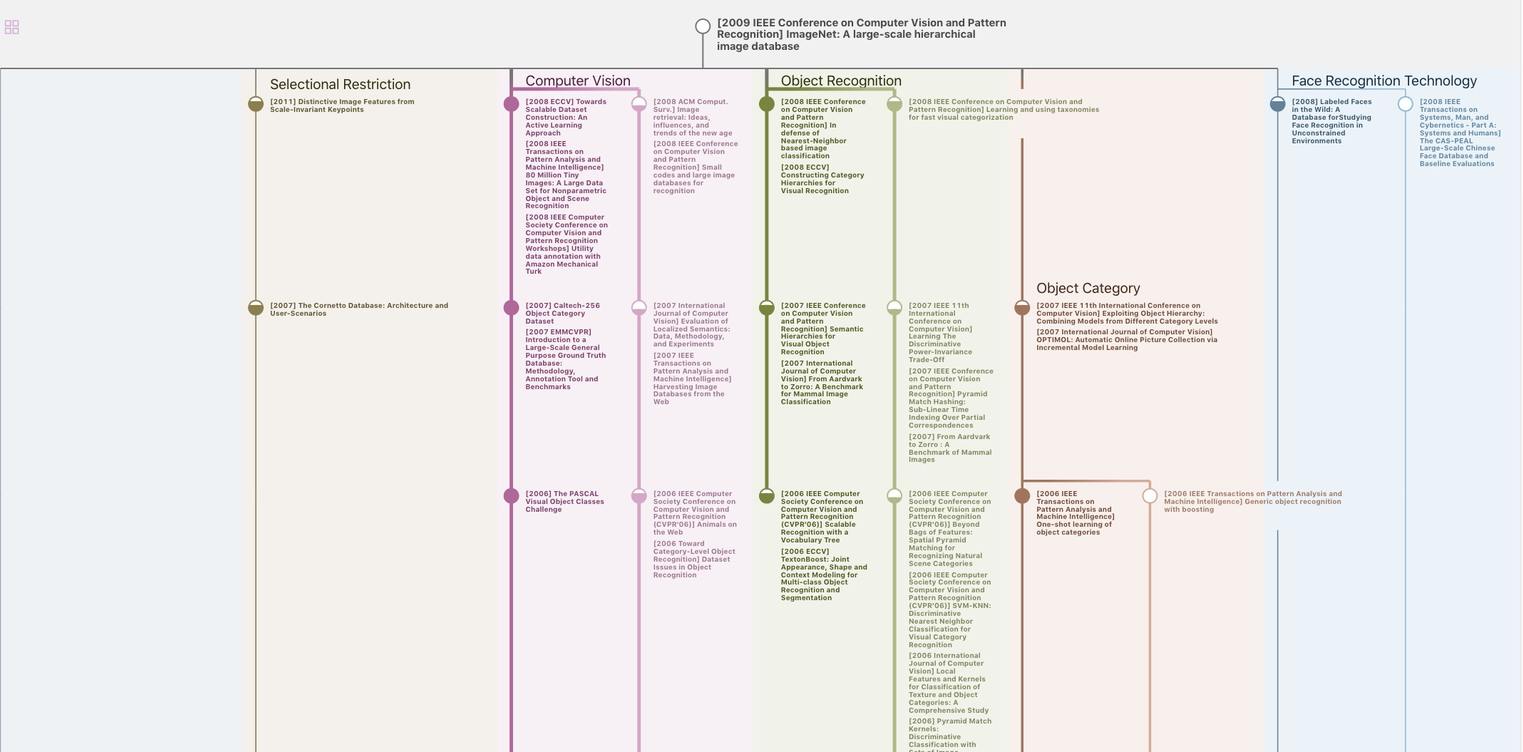
生成溯源树,研究论文发展脉络
Chat Paper
正在生成论文摘要