Numerically stable, scalable formulas for parallel and online computation of higher-order multivariate central moments with arbitrary weights
Computational Statistics(2016)
摘要
Formulas for incremental or parallel computation of second order central moments have long been known, and recent extensions of these formulas to univariate and multivariate moments of arbitrary order have been developed. Such formulas are of key importance in scenarios where incremental results are required and in parallel and distributed systems where communication costs are high. We survey these recent results, and improve them with arbitrary-order, numerically stable one-pass formulas which we further extend with weighted and compound variants. We also develop a generalized correction factor for standard two-pass algorithms that enables the maintenance of accuracy over nearly the full representable range of the input, avoiding the need for extended-precision arithmetic. We then empirically examine algorithm correctness for pairwise update formulas up to order four as well as condition number and relative error bounds for eight different central moment formulas, each up to degree six, to address the trade-offs between numerical accuracy and speed of the various algorithms. Finally, we demonstrate the use of the most elaborate among the above mentioned formulas, with the utilization of the compound moments for a practical large-scale scientific application.
更多查看译文
关键词
Descriptive statistics, Statistical moments, Parallel computing, Large data analysis
AI 理解论文
溯源树
样例
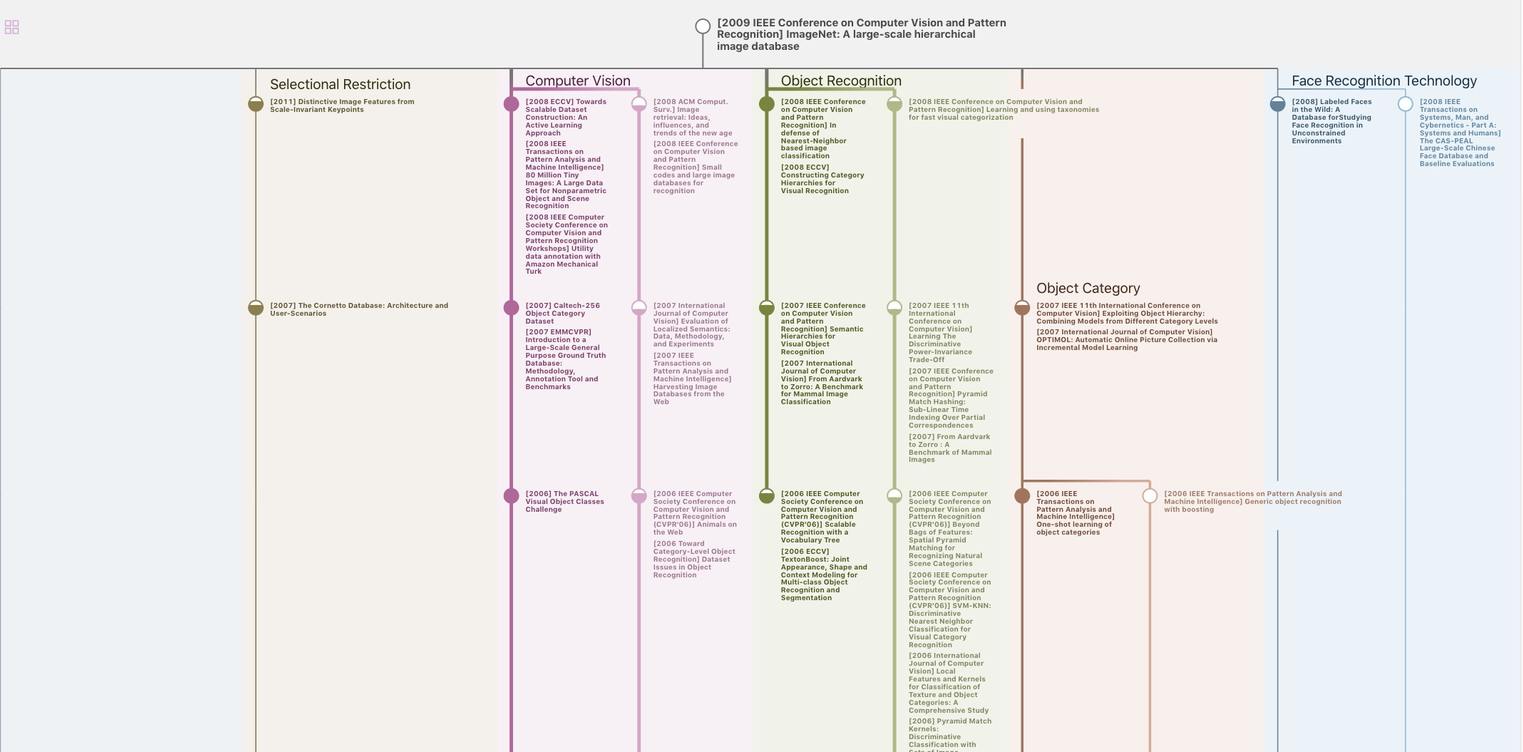
生成溯源树,研究论文发展脉络
Chat Paper
正在生成论文摘要