Uncovering the Spatio-Temporal Dynamics of Memes in the Presence of Incomplete Information
ACM International Conference on Information and Knowledge Management(2016)
摘要
Modeling, understanding, and predicting the spatio-temporal dynamics of online memes are important tasks, with ramifications on location-based services, social media search, targeted advertising and content delivery networks. However, the raw data revealing these dynamics are often incomplete and error-prone; for example, API limitations and data sampling policies can lead to an incomplete (and often biased) perspective on these dynamics. Hence, in this paper, we investigate new methods for uncovering the full (underlying) distribution through a novel spatio-temporal dynamics recovery framework which models the latent relationships among locations, memes, and times. By integrating these hidden relationships into a tensor-based recovery framework - called AirCP - we find that high-quality models of meme spread can be built with access to only a fraction of the full data. Experimental results on both synthetic and real-world Twitter hashtag data demonstrate the promising performance of the proposed framework: an average improvement of over 27% in recovering the spatio-temporal dynamics of hashtags versus five state-of-the-art alternatives.
更多查看译文
关键词
Spatial-temporal dynamics,Tensor factorization,Geo-spatial social media
AI 理解论文
溯源树
样例
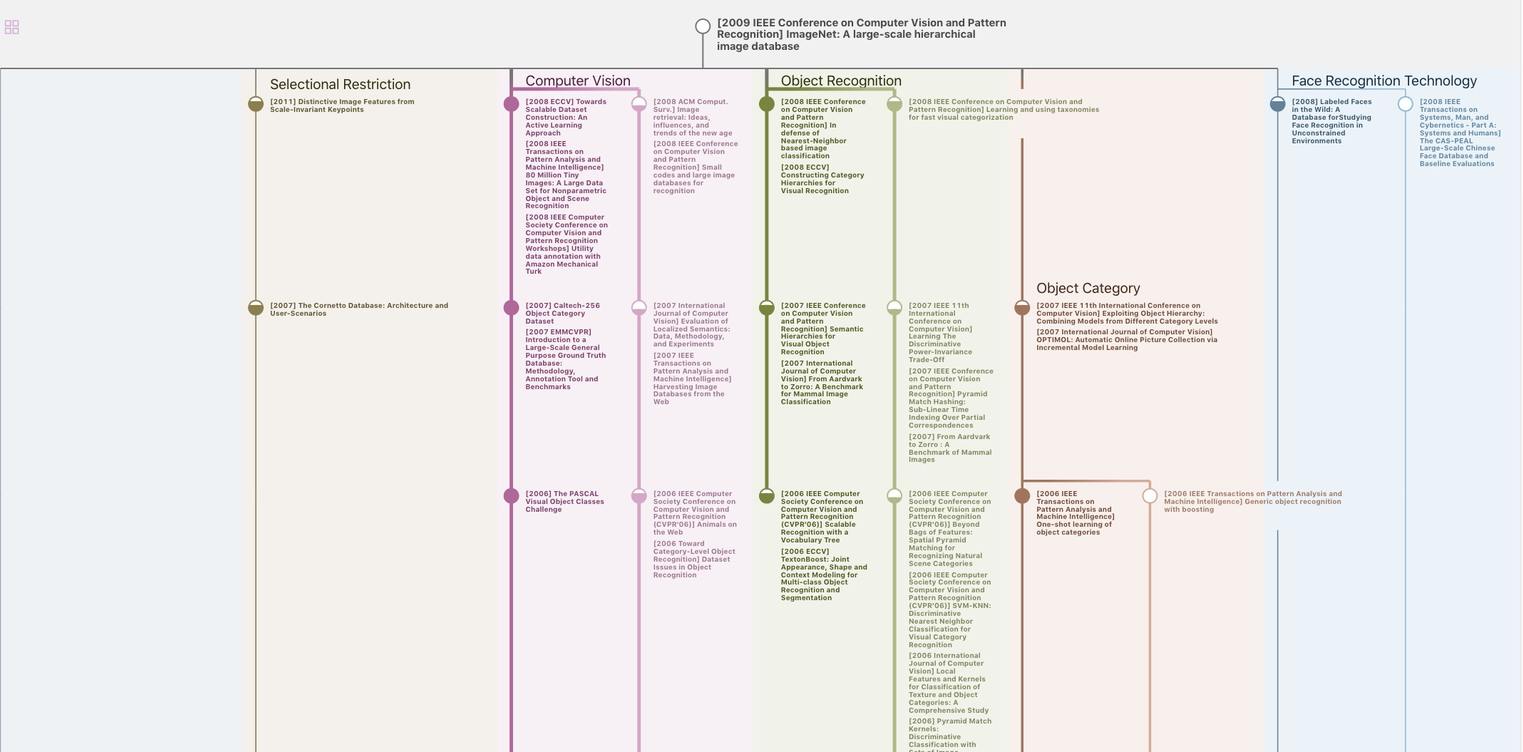
生成溯源树,研究论文发展脉络
Chat Paper
正在生成论文摘要