Graph Topic Scan Statistic For Spatial Event Detection
ACM International Conference on Information and Knowledge Management(2016)
摘要
Spatial event detection is an important and challenging problem. Unlike traditional event detection that focuses on the timing of global urgent event, the task of spatial event detection is to detect the spatial regions (e.g. clusters of neighboring cities) where urgent events occur. In this paper, we focus on the problem of spatial event detection using textual information in social media. We observe that, when a spatial event occurs, the topics relevant to the event are often discussed more coherently in cities near the event location than those far away. In order to capture this pattern, we propose a new method called Graph Topic Scan Statistic (Graph-TSS) that corresponds to a generalized log-likelihood ratio test based on topic modeling. We first demonstrate that the detection of spatial event regions under Graph-TSS is NP-hard due to a reduction from classical node-weighted prize-collecting Steiner tree problem (NW-PCST). We then design an efficient algorithm that approximately maximizes the graph topic scan statistic over spatial regions of arbitrary form. As a case study, we consider three applications using Twitter data, Argentina civil unrest event detection, Chile earthquake detection, and United States influenza disease outbreak. Empirical evidence demonstrates that the proposed Graph-TSS performs superior over state-of-the-art methods on both running time and accuracy.
更多查看译文
关键词
Spatial event detection,Scan Statistic,Latent topics,sub-graph detection,approximation algorithm
AI 理解论文
溯源树
样例
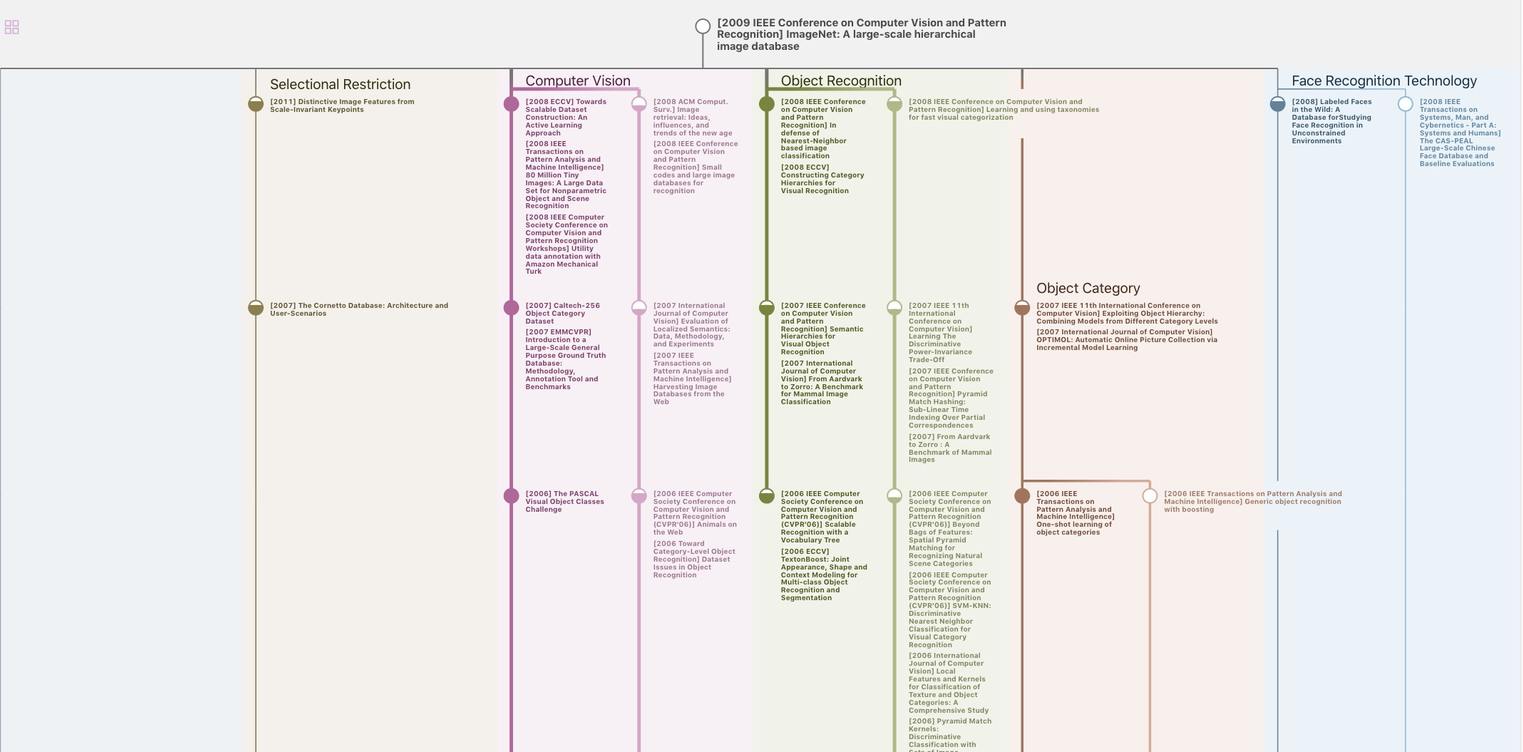
生成溯源树,研究论文发展脉络
Chat Paper
正在生成论文摘要