Parallel attribute reduction in dominance-based neighborhood rough set.
Information Sciences(2016)
摘要
The amount of data collected from different real-world applications is increasing rapidly. When the volume of data is too large to be loaded to memory, it may be impossible to analyze it using a single computer. Although efforts have been taken to manage big data by using a single computer, the problem may not be solved in an acceptable time frame, making parallel computing an indispensable way to handle big data. In this paper, we investigate approaches to attribute reduction in parallel using dominance-based neighborhood rough sets (DNRS), which take into consideration the partial orders among numerical and categorical attribute values, and can be utilized in a multicriteria decision-making method. We first present some properties of attribute reduction in DNRS, and then investigate principles of parallel attribute reduction in DNRS. Parallelization on different components of attribute reduction are explored in detail. Furthermore, parallel attribute reduction algorithms in DNRS are proposed. Experimental results on UCI data and big data show that the proposed parallel algorithm is both effective and efficient.
更多查看译文
关键词
Parallel algorithm,Rough sets,Big data,Attribute reduction
AI 理解论文
溯源树
样例
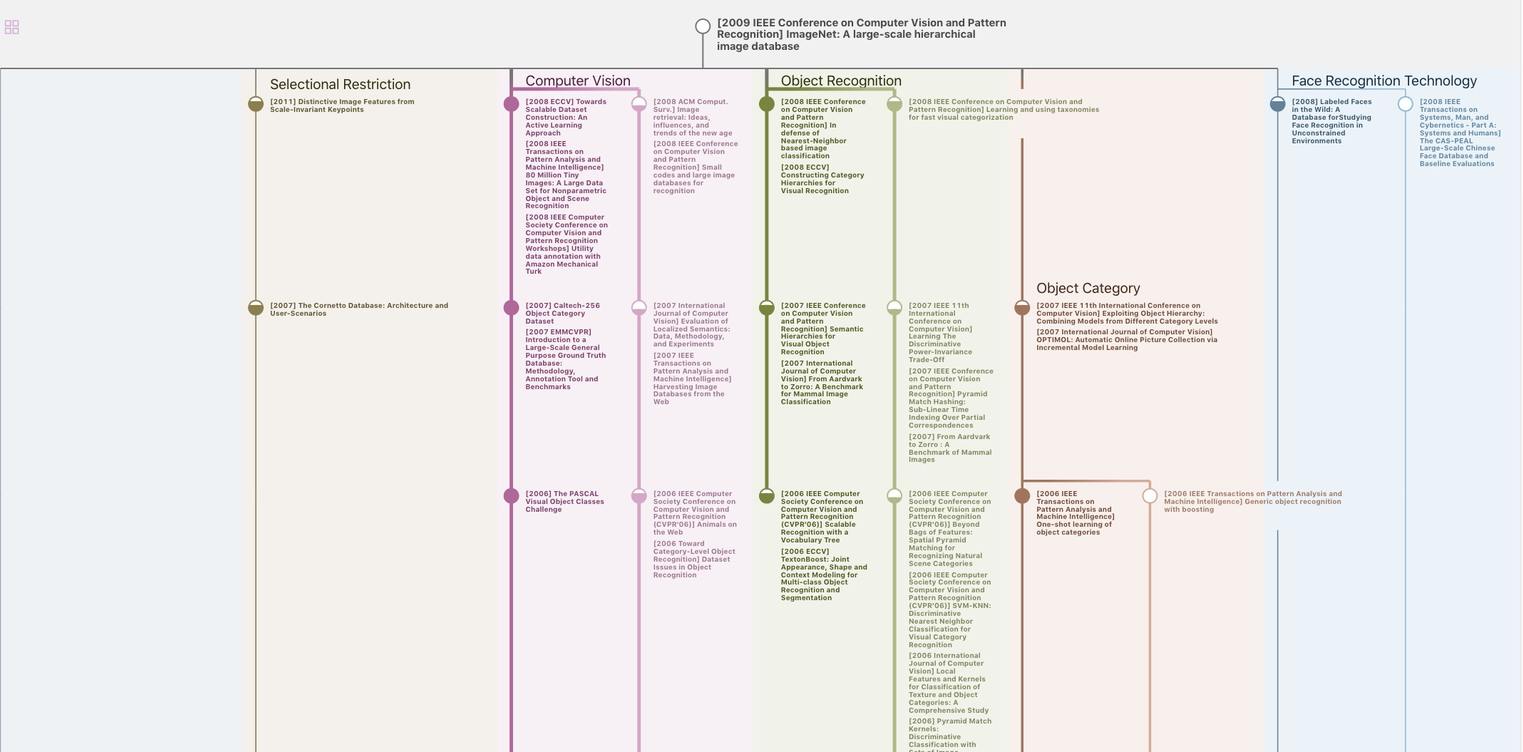
生成溯源树,研究论文发展脉络
Chat Paper
正在生成论文摘要