A Hybrid Algorithm for Missing Data Imputation and Its Application to Electrical Data Loggers.
SENSORS(2016)
摘要
The storage of data is a key process in the study of electrical power networks related to the search for harmonics and the finding of a lack of balance among phases. The presence of missing data of any of the main electrical variables (phase-to-neutral voltage, phase-to-phase voltage, current in each phase and power factor) affects any time series study in a negative way that has to be addressed. When this occurs, missing data imputation algorithms are required. These algorithms are able to substitute the data that are missing for estimated values. This research presents a new algorithm for the missing data imputation method based on Self-Organized Maps Neural Networks and Mahalanobis distances and compares it not only with a well-known technique called Multivariate Imputation by Chained Equations (MICE) but also with an algorithm previously proposed by the authors called Adaptive Assignation Algorithm (AAA). The results obtained demonstrate how the proposed method outperforms both algorithms.
更多查看译文
关键词
missing data imputation,multivariate imputation by chained equations (MICE),Mahalanobis distances,Self-Organized Maps Neural Networks (SOM),Adaptive Assignation Algorithm (AAA),Multivariate Adaptive Regression Splines (MARS),quality of electric supply,voltage,current,power factor
AI 理解论文
溯源树
样例
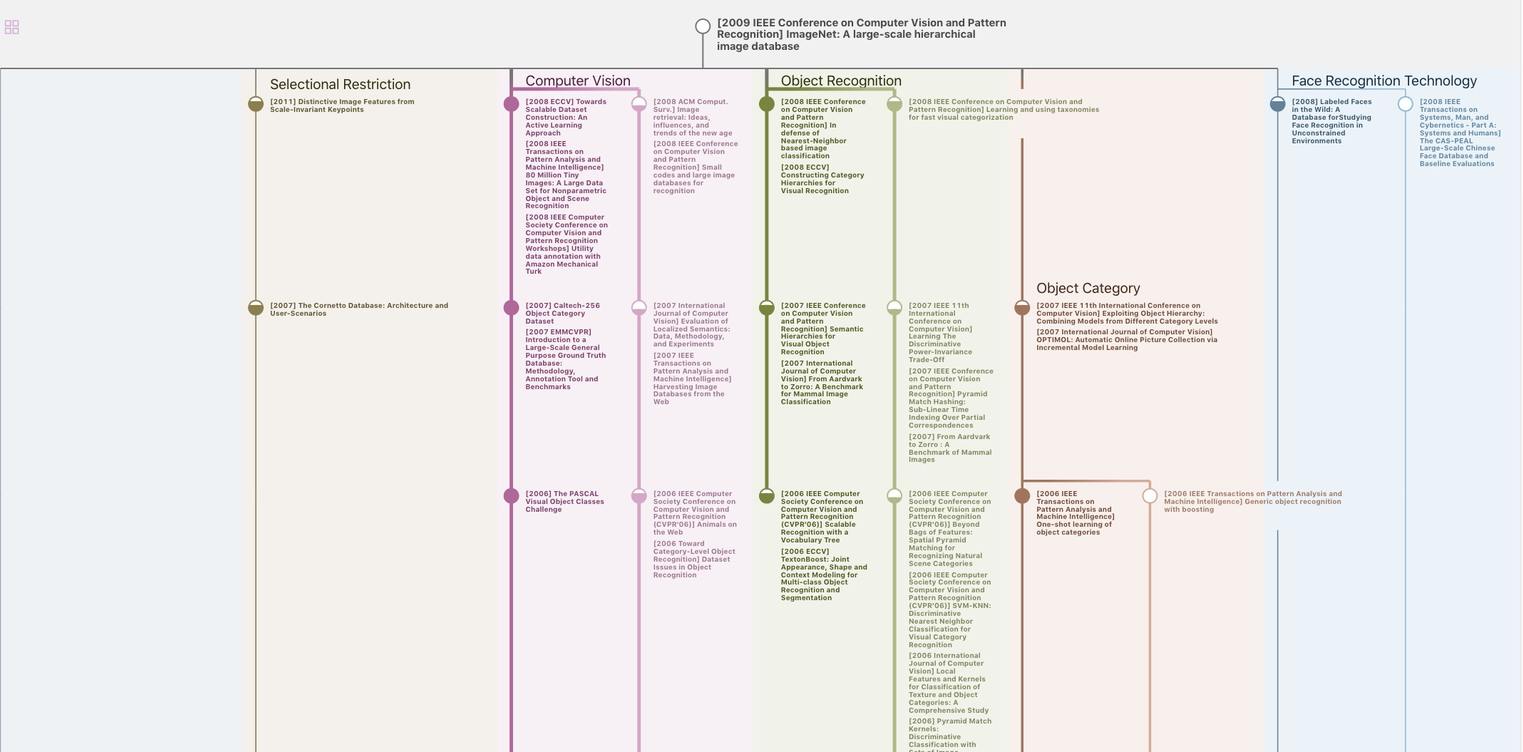
生成溯源树,研究论文发展脉络
Chat Paper
正在生成论文摘要