Application of LC-MS-based metabolomics method in differentiating septic survivors from non-survivors
Analytical and bioanalytical chemistry(2016)
摘要
Septic shock is the most severe form of sepsis, which is still one of the leading causes of death in the intensive care unit (ICU). Even though early prognosis and diagnosis are known to be indispensable for reaching an optimistic outcome, pathogenic complexities and the lack of specific treatment make it difficult to predict the outcome individually. In the present study, serum samples from surviving and non-surviving septic shock patients were drawn before clinical intervention at admission. Metabolic profiles of all the samples were analyzed by liquid chromatography-mass spectrometry (LC-MS)-based metabolomics. One thousand four hundred nineteen peaks in positive mode and 1878 peaks in negative mode were retained with their relative standard deviation (RSD) below 30 %, in which 187 metabolites were initially identified by retention time and database in the light of the exact molecular mass. Differences between samples from the survivors and the non-survivors were investigated using multivariate and univariate analysis. Finally, 43 significantly varied metabolites were found in the comparison between survivors and non-survivors. Concretely, metabolites in the tricarboxylic acid (TCA) cycle, amino acids, and several energy metabolism-related metabolites were up-regulated in the non-survivors, whereas those in the urea cycle and fatty acids were generally down-regulated. Metabolites such as lysine, alanine, and methionine did not present significant changes in the comparison. Six metabolites were further defined as primary discriminators differentiating the survivors from the non-survivors at the early stage of septic shock. Our findings reveal that LC-MS-based metabolomics is a useful tool for studying septic shock. Graphical abstract ᅟ
更多查看译文
关键词
Catabolism,Early mortality,Liquid chromatography-mass spectrometry,Metabolomics,Septic shock
AI 理解论文
溯源树
样例
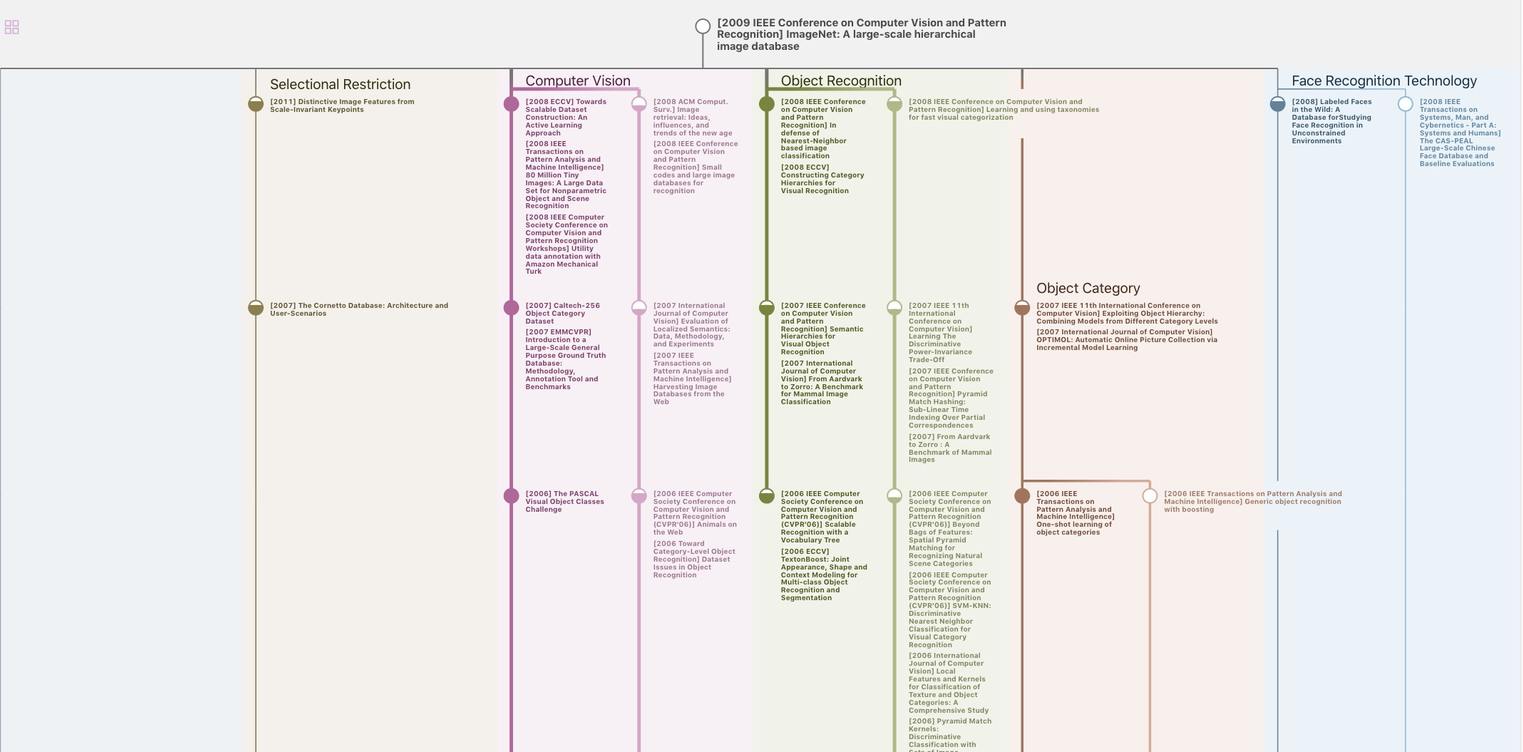
生成溯源树,研究论文发展脉络
Chat Paper
正在生成论文摘要