Annealed Mcmc For Bayesian Learning Of Linear Gaussian State Space Models With Changepoints
2016 19TH INTERNATIONAL CONFERENCE ON INFORMATION FUSION (FUSION)(2016)
摘要
Adding changepoints to a linear Gaussian state space model, such that it can switch between multiple regimes of action, provides a powerful extension which allows more complex system behaviour to be described. However, it also means that more sophisticated algorithms are needed for parameter estimation. This paper addresses the task of Bayesian learning of changepoint model parameters using MCMC. Standard Gibbs-based algorithms can suffer from poor mixing, because of the correlation between the changepoints and parameters. To avoid this, algorithms must propose changes to both simultaneously, but naive implementations of this variety generally do not perform well. The idea of using an annealed sequence of distributions to bridge between two changepoint sequences allows us to improve the mixing. We show how to implement such a scheme for a general class of linear changepoint transition models and demonstrate with a simple simulation.
更多查看译文
关键词
annealed MCMC,Bayesian learning,linear Gaussian state space model,changepoint model parameter,standard Gibbs-based algorithm,linear changepoint transition model
AI 理解论文
溯源树
样例
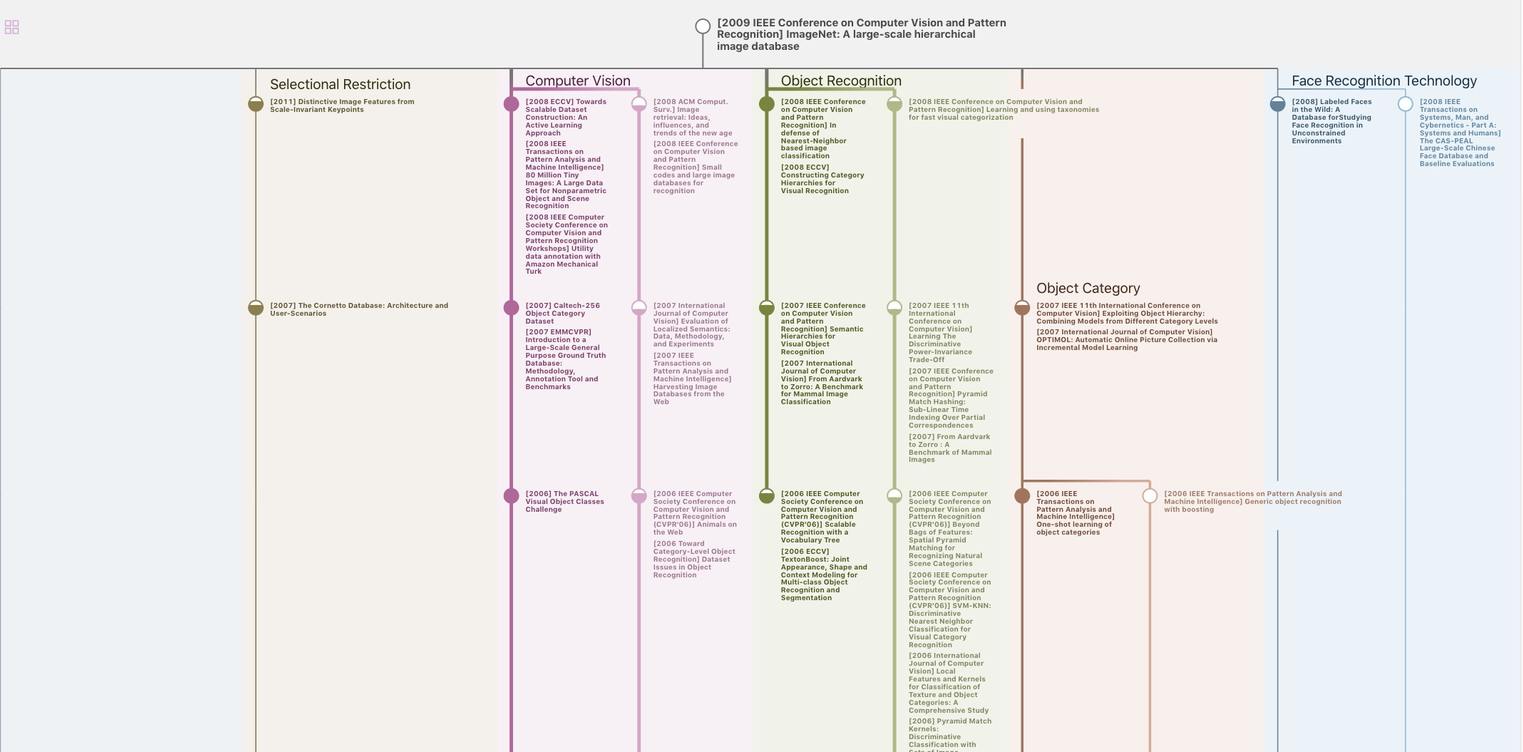
生成溯源树,研究论文发展脉络
Chat Paper
正在生成论文摘要