Motion Primitive Forests for Human Activity Recognition Using Wearable Sensors.
PRICAI(2016)
摘要
Human activity recognition is important in many applications such as fitness logging, pervasive healthcare, near-emergency warning, and social networking. Using body-worn sensors, these applications detect activities of the users to understand the context and provide them appropriate assistance. For accurate recognition, it is crucial to design appropriate feature representation of sensor data. In this paper, we propose a new type of motion features: motion primitive forests, which are randomized ensembles of decision trees that act on original local features by clustering them to form motion primitives (or words). The bags of these features, which accumulate histograms of the resulting motion primitives over each data frame, are then used to build activity models. We experimentally validated the effectiveness of the proposed method on accelerometer data on three benchmark datasets. On all three datasets, the proposed motion primitive forests provided substantially higher accuracy than existing state-of-the-art methods, and were much faster in both training and prediction, compared with k-means feature learning. In addition, the method showed stable results over different types of original local features, indicating the ability of random forests in selecting relevant local features.
更多查看译文
关键词
Human activity recognition, Wearable sensors, Motion primitive forests, Random forests, Bag of features
AI 理解论文
溯源树
样例
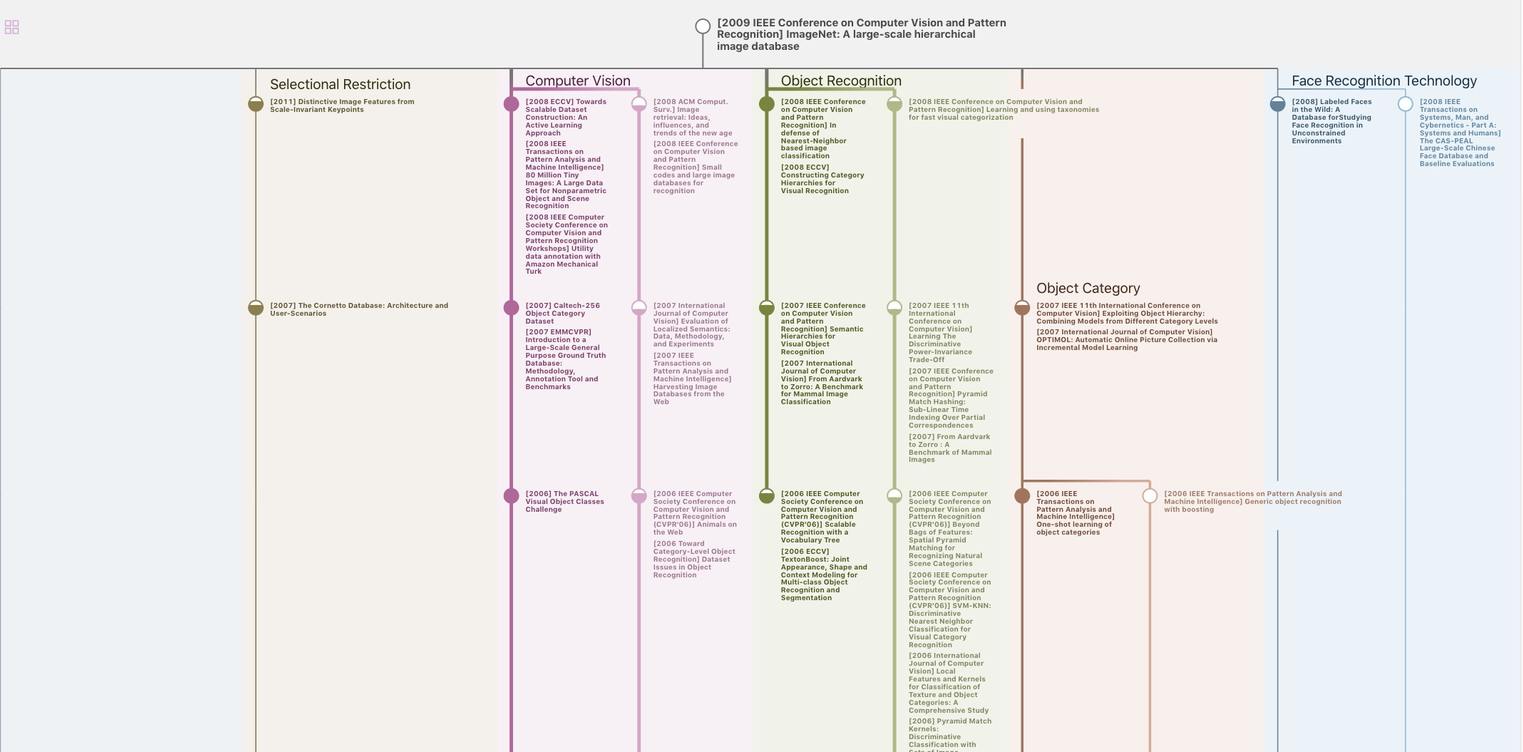
生成溯源树,研究论文发展脉络
Chat Paper
正在生成论文摘要