A Novel Isolation-Based Outlier Detection Method.
PRICAI(2016)
摘要
Outlier detection is one of the most important tasks in data analysis. It refers to the process of recognizing unusual characteristics which may provide useful insights in helping us to understand the behaviors of data. In the paper, an isolation-based outlier detection method, called Entropy-based Greedy Isolation Tree (EGiTree), is proposed. Unlike other treelike detection methods, our method exploits a half-baked isolation tree, which is constructed via three entropy-based heuristics, to identify outliers. Specifically, the heuristics are used to guide the selection process of attribute and its split value when constructing the tree. Thus, the outlierness score of each data point is estimated based on the total partition cost of the isolation node in the tree, as well as the path length and complexity of partition. Experiment results on public real-world datasets show that our approach outperforms distanced-based, density-based, subspace-based as well as state-of-the-art isolation-based approaches.
更多查看译文
关键词
Outlier detection, Data mining, Isolation, Isolation tree, Entropy
AI 理解论文
溯源树
样例
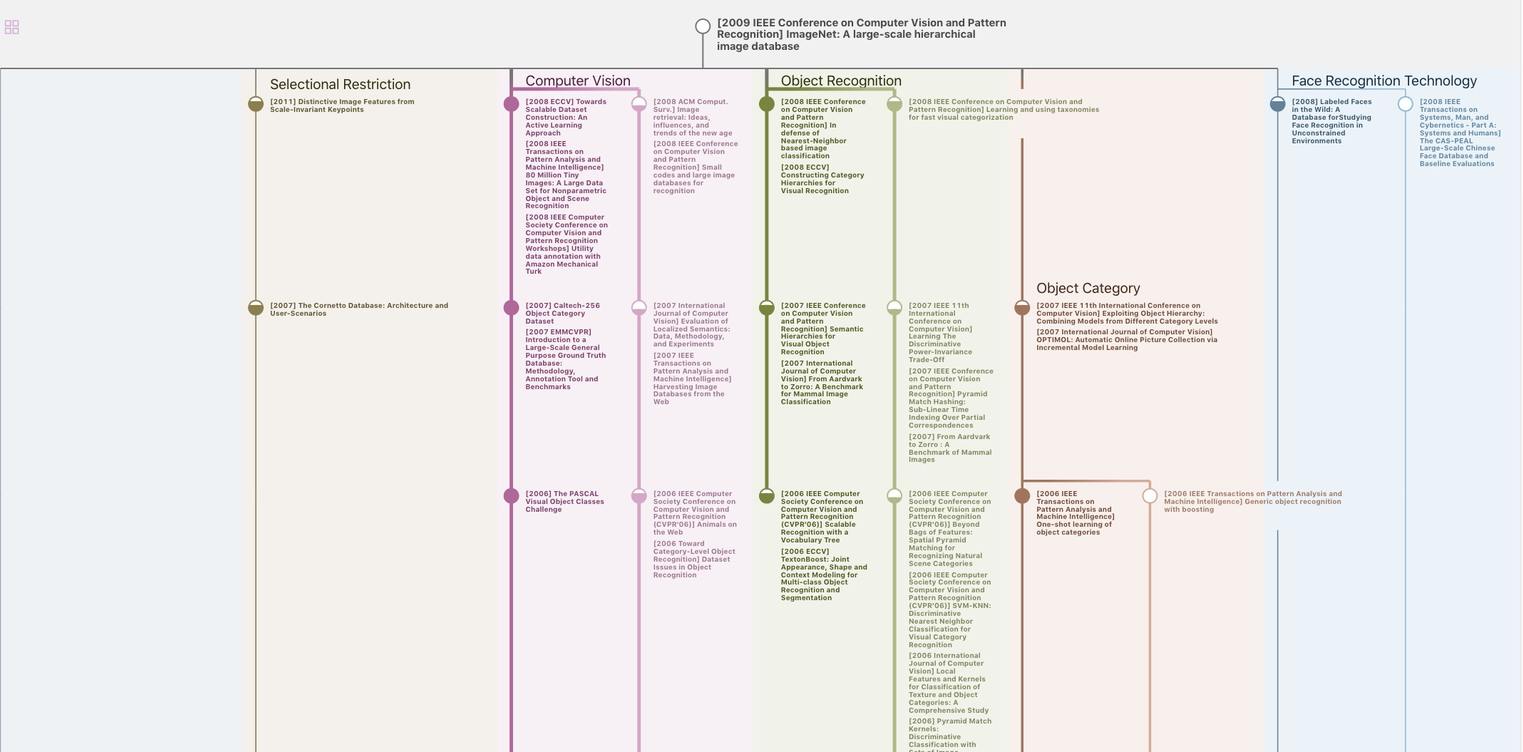
生成溯源树,研究论文发展脉络
Chat Paper
正在生成论文摘要