Learning joint demosaicing and denoising based on sequential energy minimization
2016 IEEE International Conference on Computational Photography (ICCP)(2016)
摘要
Demosaicing is an important first step for color image acquisition. For practical reasons, demosaicing algorithms have to be both efficient and yield high quality results in the presence of noise. The demosaicing problem poses several challenges, e.g. zippering and false color artifacts as well as edge blur. In this work, we introduce a novel learning based method that can overcome these challenges. We formulate demosaicing as an image restoration problem and propose to learn efficient regularization inspired by a variational energy minimization framework that can be trained for different sensor layouts. Our algorithm performs joint demosaicing and denoising in close relation to the real physical mosaicing process on a camera sensor. This is achieved by learning a sequence of energy minimization problems composed of a set of RGB filters and corresponding activation functions. We evaluate our algorithm on the Microsoft Demosaicing data set in terms of peak signal to noise ratio (PSNR) and structured similarity index (SSIM). Our algorithm is highly efficient both in image quality and run time. We achieve an improvement of up to 2.6 dB over recent state-of-the-art algorithms.
更多查看译文
关键词
joint demosaicing-and-denoising learning,sequential energy minimization,color image acquisition,zippering,false color artifacts,edge blur,image restoration problem,regularization learning,variational energy minimization framework,sensor layouts,physical mosaicing process,camera sensor,RGB filters,activation functions,Microsoft Demosaicing data set,peak signal-to-noise ratio,PSNR,structured similarity index,SSIM,image quality,run time
AI 理解论文
溯源树
样例
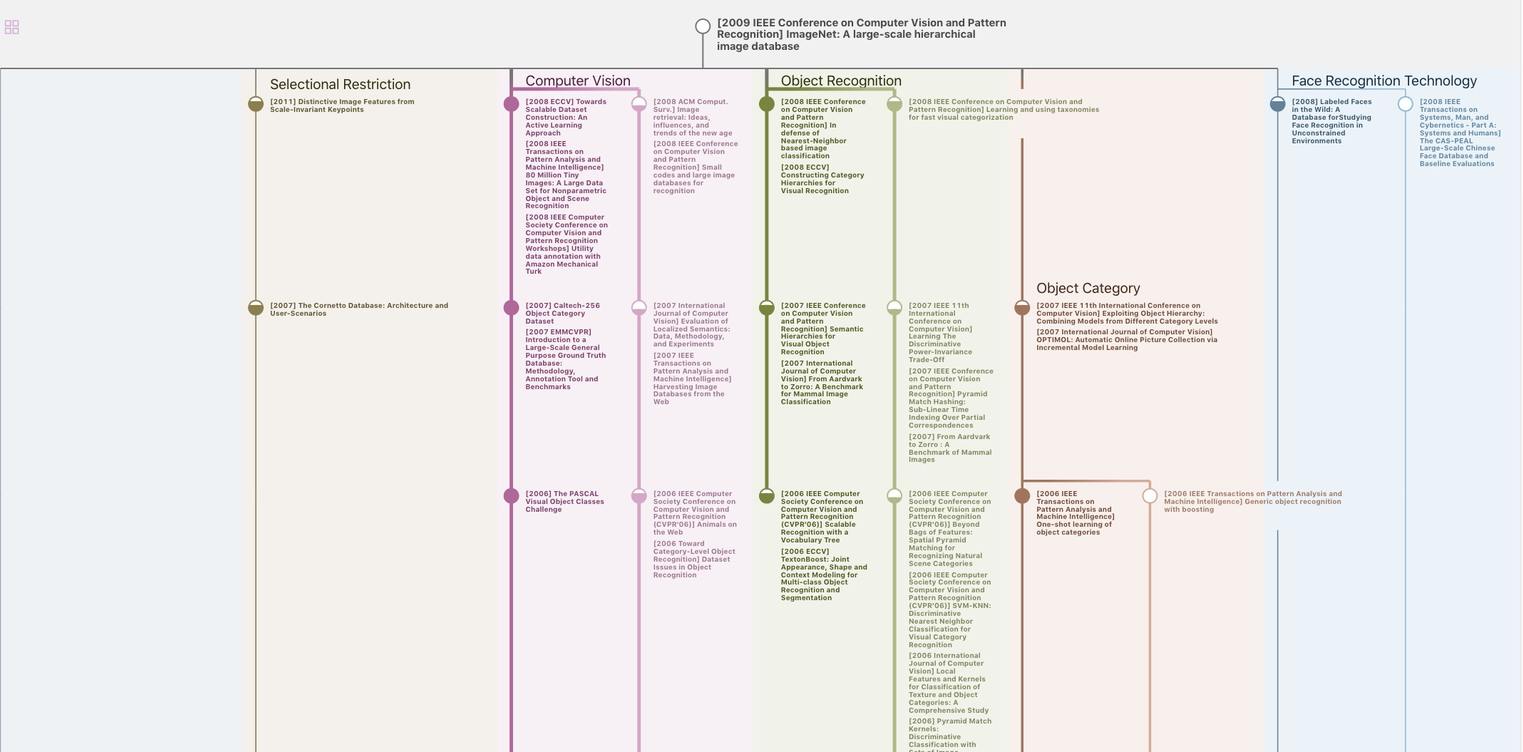
生成溯源树,研究论文发展脉络
Chat Paper
正在生成论文摘要