Combining Manual and Automatic Prosodic Annotation for Expressive Speech Synthesis.
LREC 2016 - TENTH INTERNATIONAL CONFERENCE ON LANGUAGE RESOURCES AND EVALUATION(2016)
摘要
Text-to-speech has long been centered on the production of an intelligible message of good quality. More recently, interest has shifted to the generation of more natural and expressive speech. A major issue of existing approaches is that they usually rely on a manual annotation in expressive styles, which tends to be rather subjective. A typical related issue is that the annotation is strongly influenced - and possibly biased - by the semantic content of the text (e.g. a shot or a fault may incite the annotator to tag that sequence as expressing a high degree of excitation, independently of its acoustic realization). This paper investigates the assumption that human annotation of basketball commentaries in excitation levels can be automatically improved on the basis of acoustic features. It presents two techniques for label correction exploiting a Gaussian mixture and a proportional-odds logistic regression. The automatically re-annotated corpus is then used to train HMM-based expressive speech synthesizers, the performance of which is assessed through subjective evaluations. The results indicate that the automatic correction of the annotation with Gaussian mixture helps to synthesize more contrasted excitation levels, while preserving naturalness.
更多查看译文
关键词
speech synthesis,expressive style annotation,machine learning
AI 理解论文
溯源树
样例
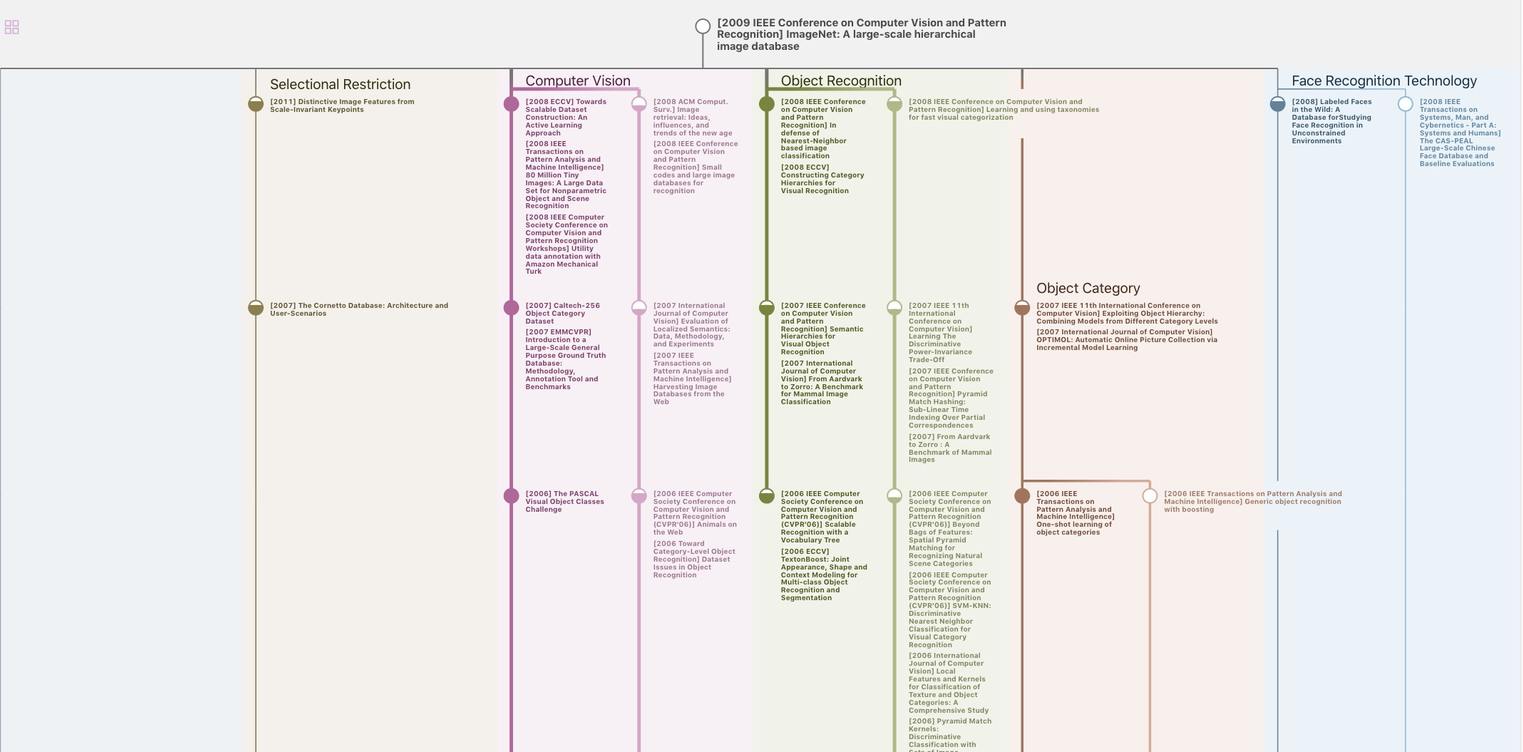
生成溯源树,研究论文发展脉络
Chat Paper
正在生成论文摘要