Page Segmentation for Historical Document Images Based on Superpixel Classification with Unsupervised Feature Learning
2016 12th IAPR Workshop on Document Analysis Systems (DAS)(2016)
摘要
In this paper, we present an efficient page segmentation method for historical document images. Many existing methods either rely on hand-crafted features or perform rather slow as they treat the problem as a pixel-level assignment problem. In order to create a feasible method for real applications, we propose to use superpixels as basic units of segmentation, and features are learned directly from pixels. An image is first oversegmented into superpixels with the simple linear iterative clustering (SLIC) algorithm. Then, each superpixel is represented by the features of its central pixel. The features are learned from pixel intensity values with stacked convolutional autoencoders in an unsupervised manner. A support vector machine (SVM) classifier is used to classify superpixels into four classes: periphery, background, text block, and decoration. Finally, the segmentation results are refined by a connected component based smoothing procedure. Experiments on three public datasets demonstrate that compared to our previous method, the proposed method is much faster and achieves comparable segmentation results. Additionally, much fewer pixels are used for classifier training.
更多查看译文
关键词
page segmentation,layout analysis,historical document image,superpixel,SLIC,autoencoder
AI 理解论文
溯源树
样例
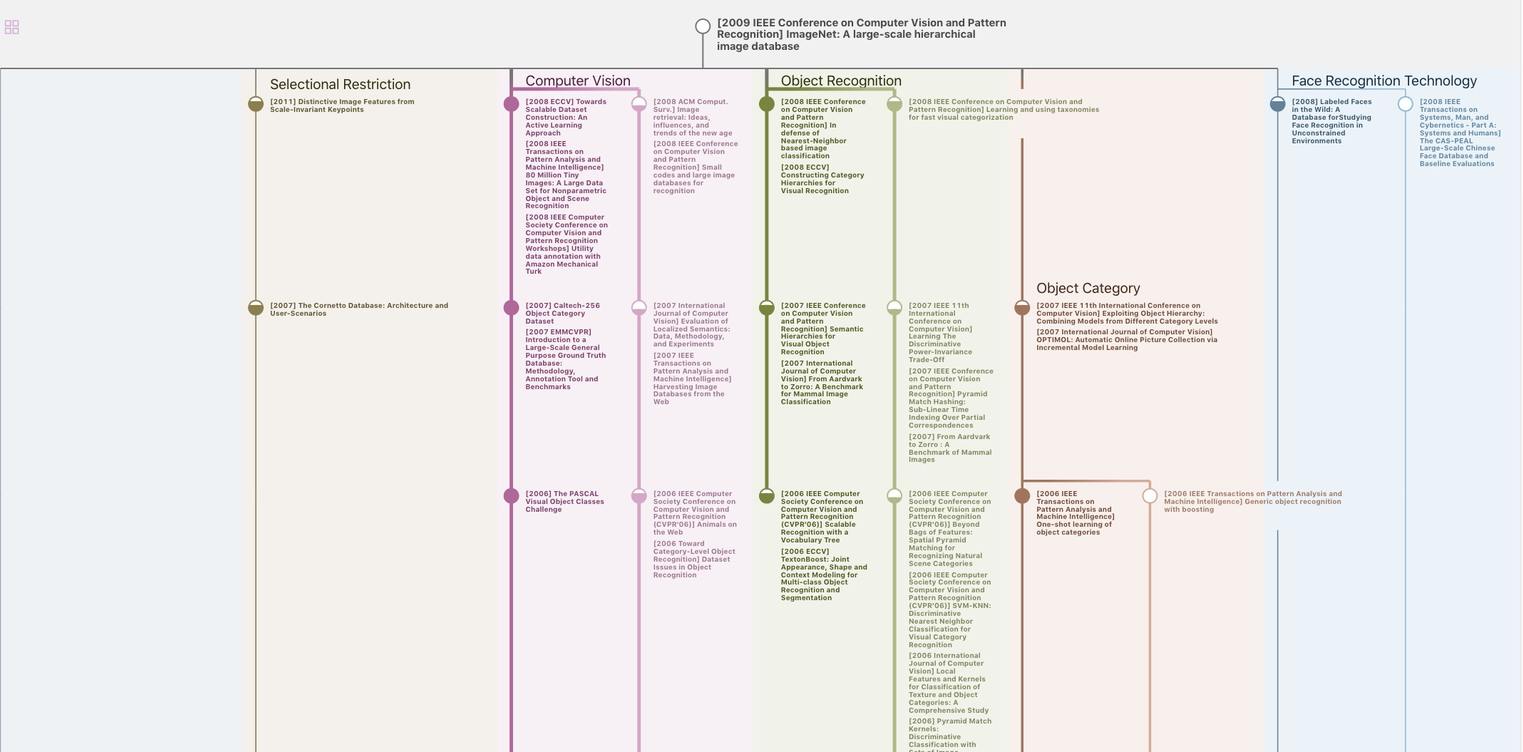
生成溯源树,研究论文发展脉络
Chat Paper
正在生成论文摘要