Local-based active classification of test report to assist crowdsourced testing.
ASE(2016)
摘要
In crowdsourced testing, an important task is to identify the test reports that actually reveal fault - true fault, from the large number of test reports submitted by crowd workers. Most existing approaches towards this problem utilized supervised machine learning techniques, which often require users to manually label a large amount of training data. Such process is time-consuming and labor-intensive. Thus, reducing the onerous burden of manual labeling while still being able to achieve good performance is crucial. Active learning is one potential technique to address this challenge, which aims at training a good classifier with as few labeled data as possible. Nevertheless, our observation on real industrial data reveals that existing active learning approaches generate poor and unstable performances on crowdsourced testing data. We analyze the deep reason and find that the dataset has significant local biases. To address the above problems, we propose LOcal-based Active ClassiFication (LOAF) to classify true fault from crowdsourced test reports. LOAF recommends a small portion of instances which are most informative within local neighborhood, and asks user their labels, then learns classifiers based on local neighborhood. Our evaluation on 14,609 test reports of 34 commercial projects from one of the Chinese largest crowdsourced testing platforms shows that our proposed LOAF can generate promising results. In addition, its performance is even better than existing supervised learning approaches which built on large amounts of labelled historical data. Moreover, we also implement our approach and evaluate its usefulness using real-world case studies. The feedbacks from testers demonstrate its practical value.
更多查看译文
关键词
Crowdsourced Testing, Test Report Classification, Active Learning
AI 理解论文
溯源树
样例
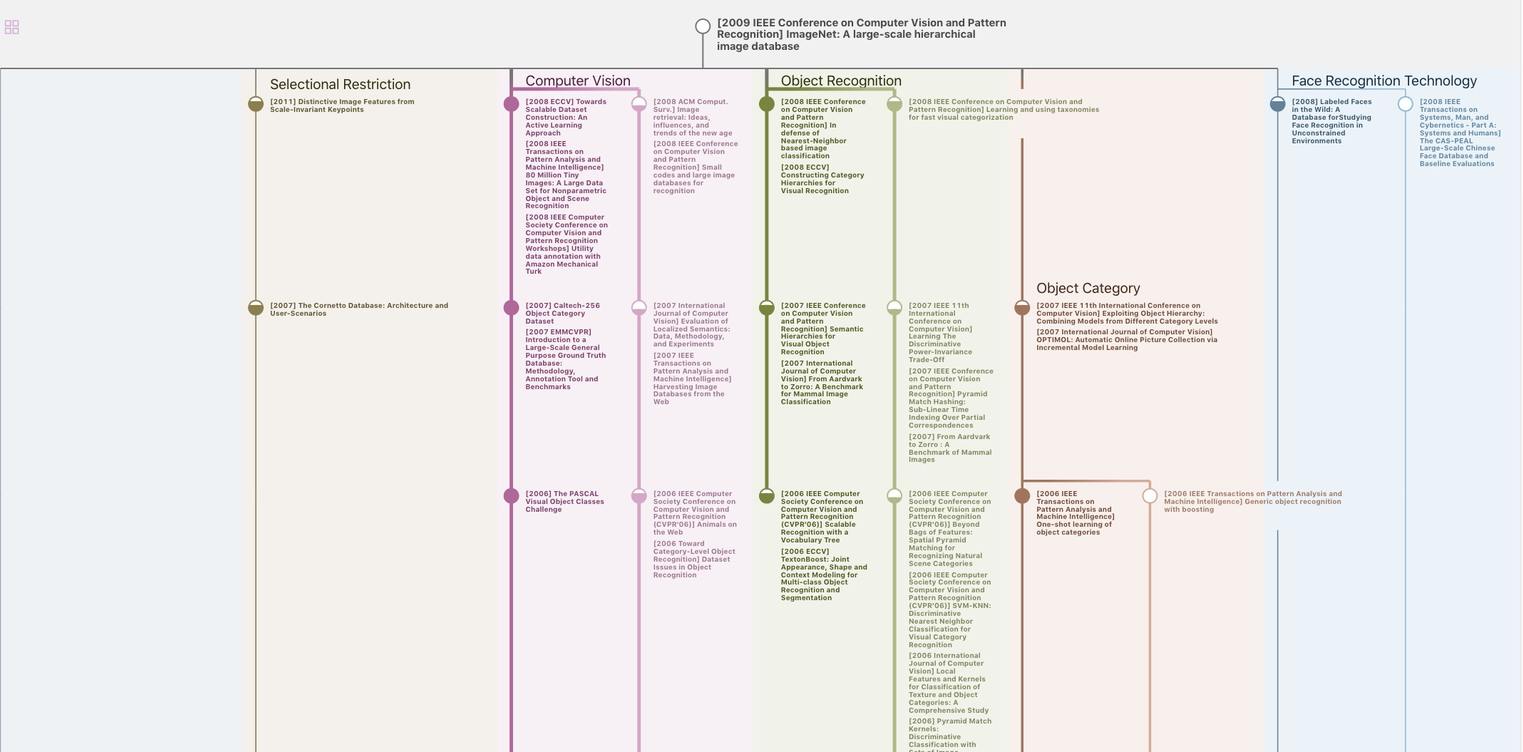
生成溯源树,研究论文发展脉络
Chat Paper
正在生成论文摘要