Hypervolume-Based Direct For Multi-Objective Optimisation
GECCO '16: Genetic and Evolutionary Computation Conference Denver Colorado USA July, 2016(2016)
摘要
DIRECT is a deterministic optimization algorithm based on the "divide-and-conquer" paradigm. It looks for optimal solutions by systematic sampling of the feasible decision space in the form of partitioning hyperrectangles. DIRECT has previously been extended to solve black-box multi-objective problems in its pure form in MO-DIRECT and in combination with multi-objective genetic algorithms in NS-DIRECT-GA. In this paper, we examine the two multi-objective variants of the algorithm in terms of diversification (well spread), exploration (global search) and exploitation (local search). Furthermore, motivated by the experimental success of indicator based methods, we provide a hypervolume-based multi-objective DIRECT, which we refer to as MO-DIRECT-hv. Through the use of the hypervolume indicator, we seek to enhance the diversification of its search through selecting potentially optimal hyperrectangles with higher hypervolume contribution, whilst preserving the exploratory and exploitation balance of the algorithm. To validate the efficacy of the proposed strategy, we compare the performance of the three variants on 55 bi-objective problems from the COCO platform. The results indicate MO-DIRECT-hv performs better than other variants due to diversified sampling.
更多查看译文
关键词
Benchmarking,Black-box optimization,Bi-objective optimization
AI 理解论文
溯源树
样例
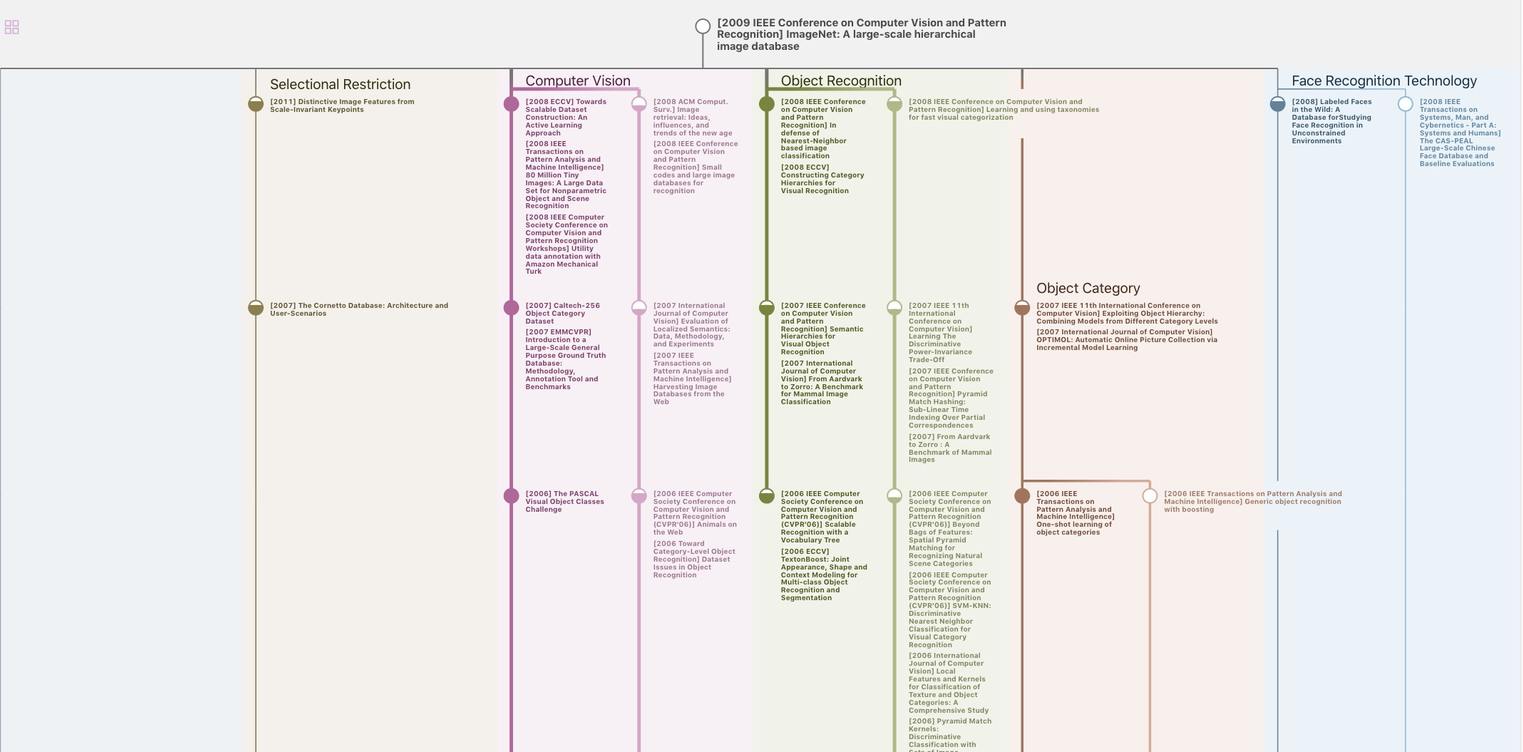
生成溯源树,研究论文发展脉络
Chat Paper
正在生成论文摘要